“Many People Get Data Storytelling Wrong.”
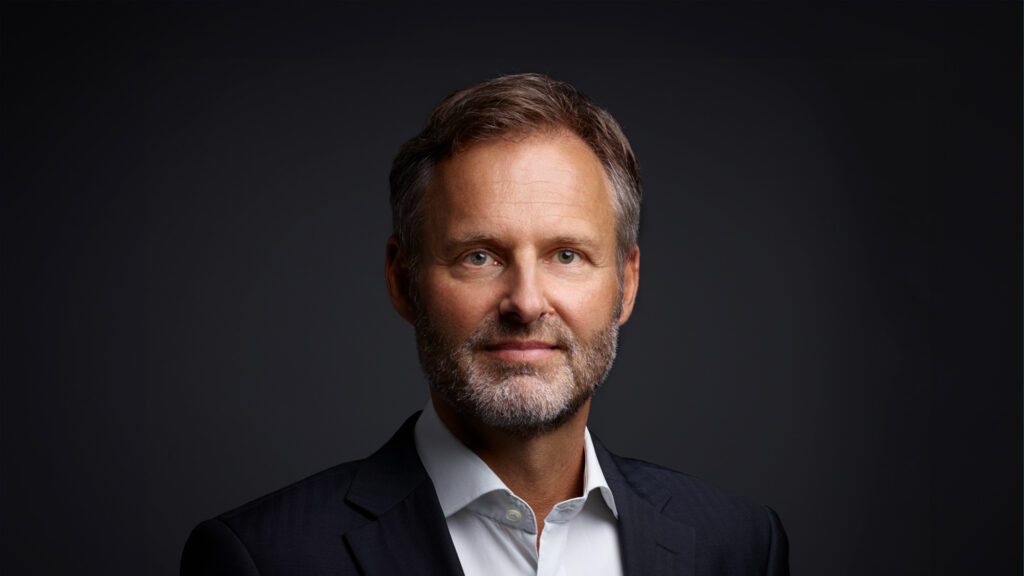
Mr. Eckert, the topic of data storytelling is discussed in many companies these days. Why is that?
Hans-Wilhelm Eckert: In recent decades, many organizations have collected a huge amount of data – both internal and external – and, in line with the “data-driven” approach, have started to make important decisions based on specific key figures. Whereas in the past, “assumptions” were perhaps still made if no precise information was available, for example on purchasing behavior or the target group of a product, today we rely on data that is sometimes more, sometimes less precise. However, this goes hand in hand with a kind of compulsion to give reasons: The figures themselves often do not provide the justification for decisions based on them. You have to interpret them and then explain why you have come to this or that assessment. This is where data storytelling comes in.
Who usually analyzes and writes?
On the one hand, we have the analysis level. And on the other, the storytelling with the analyzed data. Data experts usually do the analysis, and they do not need a more extensive story. But then there are those, usually colleagues from the communications department or marketing, who want to use good storytelling – based on the analyses – to convey the information they need to an audience.
What does that mean in concrete terms?
In most cases, data storytelling is intended to bring about a concrete change in user behavior, sales, and marketing. The data then serves as “facts” to justify such a change. The story should explain to the people in the target group as clearly as possible why this change is necessary. At the same time – and this is even more important – the data serves as a basis for determining which story or which topic can best be presented and how.
So, we are actually talking about a story with data based on data.
Well, I collect data to understand what motivates people to change and then turn it into a story in which this data is used to convince people. This fundamental understanding is very important because often exactly the opposite is done: stories are written around data without understanding where the lever for change should be applied. And then people are surprised that they have no effect.
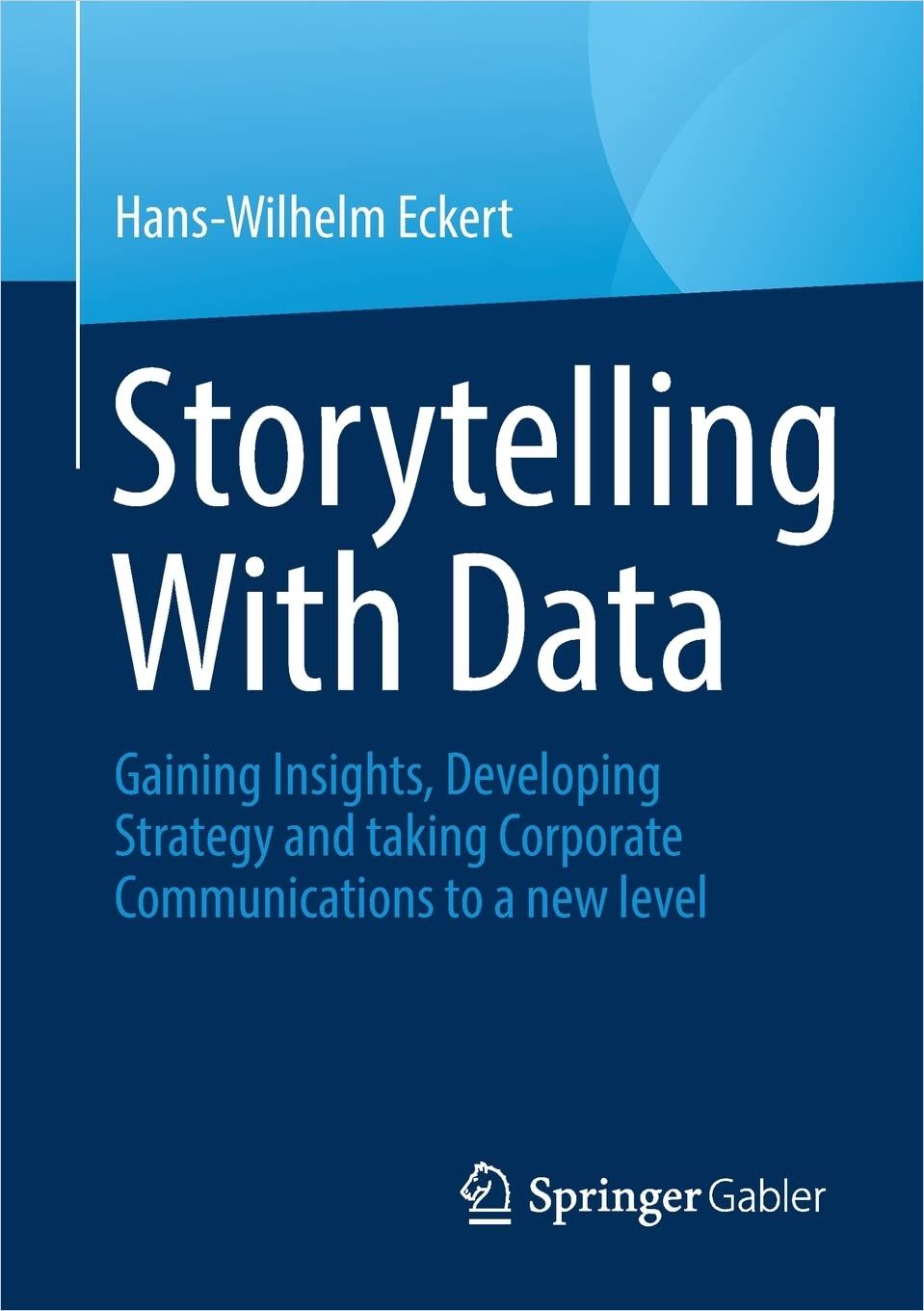
How important are visualizations in data storytelling?
Let’s put it this way: the shortest data line is between our eyes and our brain, which is why visualizations are particularly memorable. We first perceive what we see immediately and process it. We have learned to recognize patterns and immediately deduce messages from them. There is a very good example of this. The climatologist Ed Hawkins has described the so-called warming stripes, a visualization of climate science data that could not be simpler and easier to understand at first glance: blue lines for comparatively cold years, red for warm ones. At first glance, you can see the long-term temperature changes in our world. The Warming Stripes are so easy to understand and memorize that they do not need any history at all. That’s why my advice is always: leave out what you can leave out in visualizations – and then explain the rest.
Take-aways:
- Data storytelling means defining target groups and desired changes based on data to use data in the second step to convince people.
- Data storytelling is based on the classic principle of storytelling: introduction of a conflict, direct address of the target group by the protagonist and presentation of the solution.
- Visualizations are essential in data storytelling: data must be recognizable and understandable at first glance.
It starts with the headline, doesn’t it?
Exactly. A good headline is already part of the story. “The development of the economy from 1950 to 2023” may “pick up” a few economic historians, but “Economic growth defies the crisis.” may be more relevant to your target group and, therefore – with the same data! – ensures that the following is read. Again, it’s about picking people up based on data: What is the target group interested in? What problems are they dealing with? What information do they need? Get to the heart of the matter for the target group! Define a core message that the data visualization should convey and focus on it.
Can you explain this using a specific example?
Of course. I am currently advising a company on a change process. We use data to clarify to employees that changes are needed to ensure the company’s success. During implementation, we also use data to determine where we are taking employees along on the change process and where we are not. In concrete terms, we ask them for feedback at every event, workshop and communication activity. This gives us a better understanding of which topics resonate with which groups and where there is resistance. This gives us a good insight into where we are in the change process and what specific challenges we are facing.
In this case, data storytelling should help managers to convince employees. Can employees also turn the tables to present new ideas and improvements to their managers?
In any case. Data storytelling is one of the most effective methods to convince the manager of an idea or an investment. However, it is time-consuming because you usually have to gather the data laboriously. Let’s take the example of you working in sales and realizing that My turnover is falling. When searching for the causes, you need access to the relevant data, for example, from store and CRM systems. Once you have collected and evaluated the data, you realize your core target group has changed. Therefore, you must adapt your product selection, sales channels and communication, among other things. You can’t do this in one day.
Today, data storytelling is something that is particularly suitable for large projects or topics.
In other words, truly comprehensive transformations in business processes or fundamental changes in the business model if you realize that your service or product is no longer selling as well as you would like.
Usually, there is no shortage of collected data in most companies. But how do I find the right and relevant ones that are important for my storytelling?
Finding relevant data is not the biggest problem. It is much more challenging to ask the right questions. Or, to put it so succinctly, if you don’t know what you want or want to know and should know, then all the data is wrong. For example, I am currently supporting a company in the aviation sector. They have to channel a huge flood of market research data. They want to make their global communication more precise and target group-oriented. The first step is to summarize the data in clusters to see where there are correlations. The analysis of the data showed that there are two important stakeholder groups that have very different expectations, experiences and needs. Even “global” communication cannot be controlled in this context; the respective countries must focus on different topics. You convince American stakeholders and customers with different topics than you do with Indian ones. So, if you forget to ask yourself the question about your target groups and their needs at the very beginning, no one will get a satisfactory answer.
And what if your own data does not answer a specific question?
Let’s stick with my practical example: the company I just mentioned has a vast database that is continuously fed by new digital surveys and media monitoring. The aim here is to recognize patterns and filter out specific data that provides answers to questions such as: Which contact points play which role in the funnel, for example, in terms of reach and impact? Which topics generate a strong response from the target group, and which ones are less so? We are currently reviewing this. Data storytelling usually takes place with a view to existing KPIs. Here, however, we are taking a different approach. We make assumptions and try to validate or falsify them. In this case, we are looking at the resonance of topics in terms of content and their impact on the brand’s image. If the assumption is confirmed, we can pat each other on the back and be pleased that we were right. If we manage to disprove the thesis, we learn something new. That’s actually even more important. And if we can’t find any answers within our own team, i.e., the data situation is inadequate, we have to look for external sources.
Market research institutes and consulting firms offer many services in this area, but they are expensive. Many companies, therefore, look for “free” data. In other words, they search the Internet and Google. It’s particularly important to take a close look at the sources here, isn’t it? Especially now when AI-generated fakes are flooding the web.
Absolutely. When it comes to large language models and generative AI, they tend to hallucinate when it comes to data, numbers and logic – without any criminal energy, which plays a role in fake news or fakes. If the machine provides answers, we no longer really know how it arrived at the corresponding result, which makes it difficult to verify. The topic is currently the subject of heated debate among data analysts.
In the past, one of the main problems in dealing with data was that it was misinterpreted by humans or that errors occurred during collection. Now, a third level has been added: that the machine spits out incorrect data because it uses the wrong or incorrect sources, and nobody asks critical questions.
What role does data protection play, and what data should you keep your hands off when it comes to data storytelling, even if you have it?
First and foremost, data protection means protecting personal data. If, for example, you tap into sources such as customer relationship data or information collected by store systems, you are walking a fine line, as this involves personal data. In these cases, anonymization is important. It is best to talk to your data protection officer regularly. Exchange information. And: If you conduct surveys or studies, ensure participant or user data is anonymous. If you keep these things in mind, there will be no problems from a data storytelling perspective.
Let’s get specific again: I have found the relevant data because I asked the right question. The sources are reputable. I know my target group. What are my next steps to write the story?
They now start the exploratory phase. In other words, analysts look for anomalies in the data: They look at a table and then tap directly with their finger on relevant results because they are very skilled at this. Of course, you can also use evaluation tools to help you, but:
When it comes to preparing data to be used for data storytelling, humans are still the best choice.
He can prepare, restructure and aggregate the data and see where it may need to be enriched or supplemented. This is basically when the storytelling begins. With the specific question: What do I want to change with my story?
What are the biggest mistakes I can make in this process?
If you don’t think enough about the target group in advance, you will never find the right question. So, in what context does it operate? Where can I dock my story? What patterns do these people follow? This is where the circle closes: you first need data on your target group in order to motivate them to change with further data packaged in a visualization or a story.
Who is actually responsible for data storytelling in an organization?
A good question. Especially when there are many silos in the company, i.e., everyone collects their own data and doesn’t share it, it becomes difficult. When we advise companies, we talk to the people who collect the data and the users, such as the communications and marketing departments. This is where the threads should come together. After all, they are the ones who ultimately have to make decisions, approve the associated goals and measures and are therefore also responsible for success.
Which brings us to the topic of whether the success of data storytelling can actually be measured.
This is one of the most difficult questions and the one that is even more hotly debated than the question of how good AI is as a source! (Laughs.) Our advice is to use the question as a basis for setting a clear goal. The more concrete it is, the more measurable it is.
Use the familiar SMART goals: specific, measurable, attractive, realistic and time-bound.
Unfortunately, I keep finding that goals are rather abstract: We want to increase our turnover! We want to win new target groups! This requires concrete figures and a specific date by when something should have happened with the target group. For example, a company wants to increase a certain aspect of its brand image among its core target group in a specific target market by a factor of X in the current year. Only when you define these things in such detail can you measure success.
About the author:
Hans-Wilhelm Eckert owns the consulting agency Momentum Communication and works as a press spokesman, journalist, consultant, marketing and investor relations manager.