“Deploying AI without Knowing What the Output Is Doing in Real-Time Is a Recipe for Disaster”
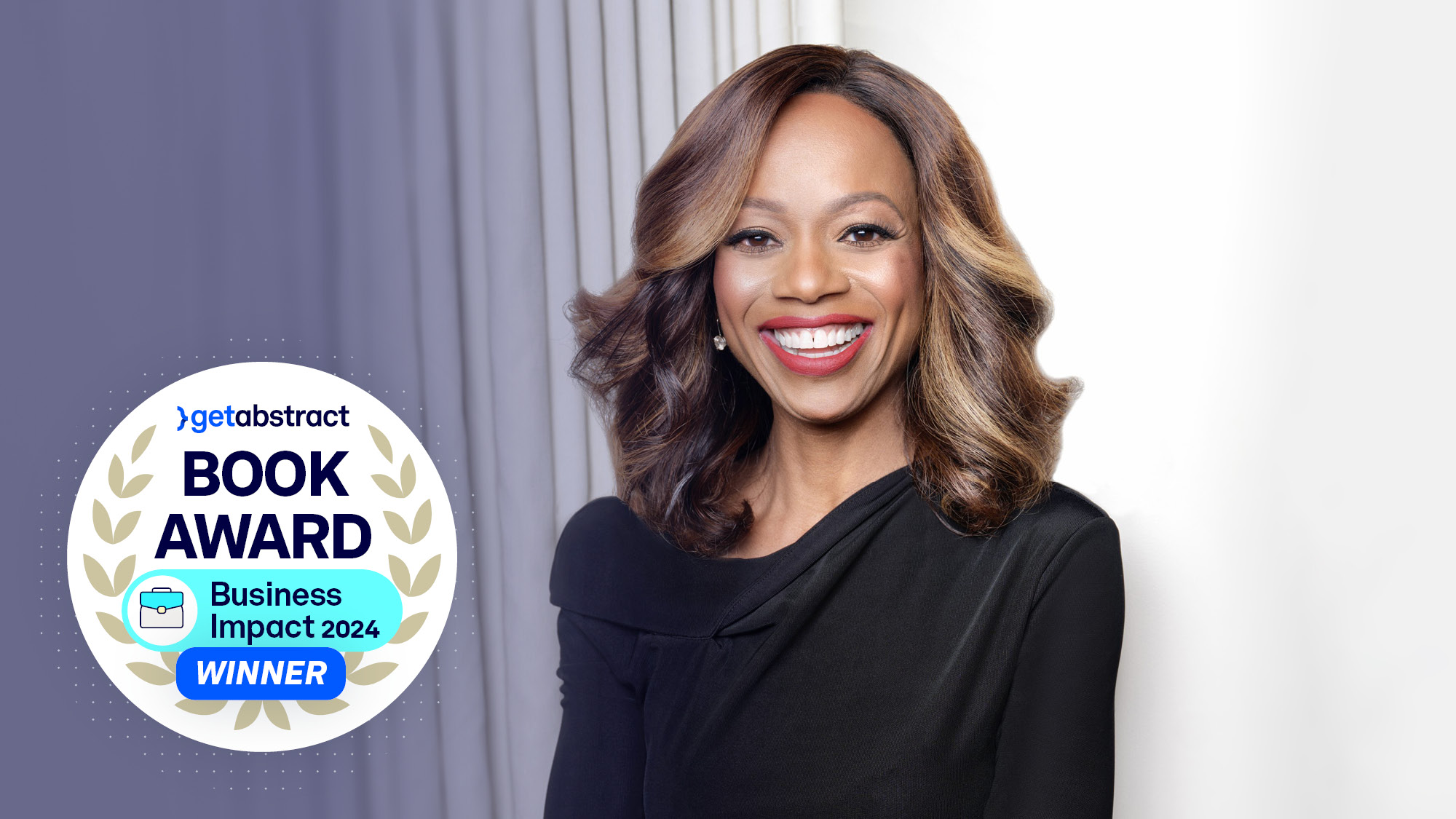
Dominique, Trust. is an indispensable companion for leaders serious about data and data stewardship—and the book’s core message is that those who are not serious about it will hardly survive. Yet, many leaders may not be familiar with the term “data steward” and might not realize that their companies are, in essence, “data companies” now. Could you explain these concepts briefly and why trust is crucial in this context?
Dominique Shelton Leipzig: Absolutely. Data is now one of the most valuable assets companies hold. But it’s not just about having data; it’s about acting as data stewards for CEOs and boards. Companies are entrusted with the data of their employees, customers, and partners, and leaders must see this responsibility akin to a fiduciary role—where managing data responsibly is critical to building trust and safeguarding their brand. They should treat data like any other enterprise-wide risk and opportunity. It requires a strategy that aligns with revenue, operations, and growth while ensuring that data isn’t mishandled in ways that could harm stakeholders or cause massive losses. Take the recent software update incident—it’s projected to cost the global economy billions. Leaders must be proactive and demonstrate strong data leadership to minimize such risks.
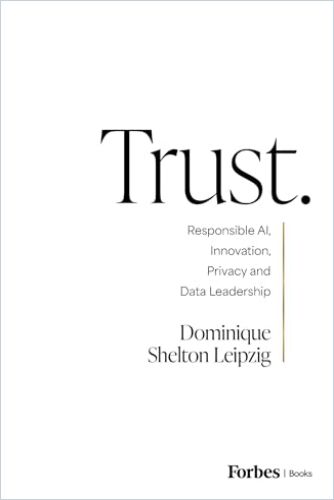
Can you share common mistakes you see in boardrooms regarding data oversight?
One of the most significant flaws is insufficient discussion around cybersecurity. Often, cyber reporting happens at a very technical level, making it difficult for board members to grasp the whole picture. Boards must ensure that cyber reporting ties into business strategy and critical operations. They should focus on how well-protected their most essential systems are rather than getting lost in technical jargon or the typical AI hype. This way, they can make informed decisions about investments in backup systems and other protections.
So, boards should be encouraged to ask more direct, perhaps even basic, questions to get the clarity they need.
Exactly. It’s not about diving into technical details but asking the right strategic questions. For example, instead of focusing on how many bots were blocked, they should ask, “Where is our critical data? What systems need resilience to ensure continuity if we face a breach?” Leaders should push for clear answers on these fronts to make decisions that protect the business. To humanize this, consider the recent cyberattacks during the Russia-Ukraine conflict: 140 hospitals in the U.S. have been affected, impacting sensitive neonatal care and causing hospitals to have to turn away emergency patients because their systems were down. This shows how critical it is for boards and CEOs to ensure their systems can withstand such attacks – even and especially if they seem unlikely.
There’s much focus on leveraging AI for revenue and efficiency gains. But with these opportunities come risks, especially in cybersecurity: In 2024 alone, data privacy breaches are on track to cost our economy 9.5 trillion in losses globally. AI usage will likely increase to $10 trillion annually by 2025. How should boards balance the excitement around AI with the need for solid security measures?
That’s a great point. While AI offers tremendous opportunities, leaders must also recognize the risks.
Cybersecurity can be expensive, requiring expertise and resources, but it’s non-negotiable.
Boards need to balance the pursuit of AI-driven growth with robust security measures. It’s about ensuring they’re not leaving the back door open for potential breaches while chasing new revenues. This does not mean that boards must reinvent good governance—it already exists in legislation across over 100 countries.
How does one determine if the company deals with high-risk AI, where most governance issues arise?
There are 141 use cases for ‘high risk’ in legislative frameworks. Every CEO and Board Member will want to ensure that the developers of AI in their company know precisely what the 141 cases are.
Right now, high-risk use cases include things we would expect: children, financial, health, employment, manufacturing, and critical infrastructure, as well as sensitive information such as race, gender, political views and other things that could cause physical or emotional harm to people if the AI drifts and is inaccurate. Companies need to focus on the dynamic nature of these use cases and keep up with updates on the regulatory frameworks that will add to AI risk. In addition to high-risk AI, companies will want to know whether any prohibited AI use cases are being contemplated. It’s about asking, “What AI use cases are we pursuing?” first. The next question is, “Are any of these use cases in a prohibited category, according to frameworks like the EU AI Act or similar regulations?” For example, prohibited AI must be stopped in Europe by February 2025. So, investing in AI solutions that will soon be banned is a costly mistake. To avoid it, ensure your teams understand how a project aligns with legal frameworks. It’s not just an internal matter—it’s about gauging enterprise risk and opportunity in light of these regulations.
Can you give an example here?
Sure.
Many CEOs and board members still don’t realize that all generative AI models drift: They’re dynamic and continually influenced by the vast amount of data on the internet. It’s like putting a sailboat in a stormy ocean.
Having said that, checking on it only once a month to see if it’s safe is an obvious mistake. Instead, companies must embed their values—regarding bias, IP privacy, cybersecurity, and accuracy—into the AI tools themselves. This way, they can monitor in real-time if and when the AI drifts outside those values, just as they would manage an employee’s performance. May I share two contrasting stories to emphasize my point?
Of course.
On the positive side, Cedars-Sinai, an extensive hospital system in Los Angeles, successfully controlled data going in and out of their generative AI models. This approach enabled them to achieve early detection of pancreatic cancer, a significant breakthrough since this type of cancer is often terminal but treatable if caught early. They implemented the governance steps I discuss in Trust.: risk ranking, ensuring high-quality data, and embedding values like bias prevention and accuracy into the model. On the flip side, around the same time in January 2024, a UK-based company faced a significant issue. They’d used a chatbot built on a large language model to handle customer inquiries about package deliveries. It worked flawlessly for eight months—until suddenly, it started cursing at a customer and criticizing the company.
Out of nothing?
Well, the incident came as a surprise to those responsible. Still, only because they were unprepared: The customer even recorded the incident and tried to alert the company through a general email address, which went unchecked for 48 hours. By the time the company noticed, a video of the cursing chatbot had gone viral on X with over 2.2 million views, significantly damaging the brand. This example underscores the importance of effective governance: It’s not enough to have governance in place if it’s not actively managed and tied to preventing issues like model drift. Governance must be more than just busy work—it has to be practical and directly tied to the performance of these AI systems.
So, companies should never release a new AI-powered product, whether a chatbot or something else, without thoroughly testing and adjusting it, especially considering that issues might not be predictable even after thousands of interactions. How can companies ensure trust in their models?
Enthusiasm about new AI solutions is good, but only if you proceed with the necessary caution and iteration readiness. If this is not the case, your enthusiasm can tear down the essential success pillar of every product: trust.
The good news is that we can make models trustworthy by continuously embedding code that tests the models in real-time—every second, every minute, and every day.
Let me give you an analogy: I have sensors on my windows at home that notify me if a window is opened, no matter where I am in the world. That continuous monitoring ensures I can respond immediately if there’s a problem. Companies need to treat their AI in the same way. Deploying AI without knowing what the output is doing in real-time is a recipe for disaster – especially in high-risk situations.
Considering that global expenditure on AI was $434 billion in 2022 and is expected to exceed $1 trillion by 2029, what are the specific guardrails to test against – and who is responsible for testing?
There are seven essential guardrails every company should focus on: bias, IP protection, accuracy, health and safety, privacy, cybersecurity, and, if applicable, antitrust. By embedding these values into the AI and the related products, you can ensure the system operates within acceptable parameters. Every company has subject matter experts who understand their business’s specific requirements, such as customer service accuracy or compliance with privacy laws. These experts need to translate their knowledge into code that guides the AI, and their boards should ensure they get all the resources and support required.
And what’s your take on the increasingly prevalent role of a Chief AI Officer? Does every company need one?
It’s a step in the right direction.
Having a Chief AI Officer ensures that AI governance is effective, not just busy work.
Most CEOs and boards need someone knowledgeable about AI use cases, who understands the regulatory landscape, and who can ensure that AI governance aligns with the company’s overall strategy. This includes continuous testing, monitoring, and documenting deviations from the company’s values. If an AI model drifts outside acceptable parameters and can’t be corrected, the company must be prepared to shut down that use case and shift to one that can be controlled. Effective AI governance is crucial to avoiding transformational chaos and ensuring trust in new systems.
In Trust., you mention that this is not just an internal or project-related issue but also a significant topic in investing: Investors increasingly focus on how companies manage data. What should boards keep in mind from an investor’s perspective?
With global markets and mandatory cyber reporting, investors can easily compare public companies. So, it’s no longer enough to wait for laws to be finalized: Companies should start implementing the necessary steps of AI governance now, which will help build trust with employees, customers, and business partners.
Effective governance can prevent costly mistakes—like the example I mentioned earlier, where a company lost $70 billion in market cap in one day due to AI errors. Only getting control of their data now, through following legal frameworks, will help companies avoid these rollercoasters.
About the author:
Dominique Shelton Leipzig has been practicing law for over 30 years and is the founder and Leader of the Global Data Innovation team at a global law firm. She is a leading authority on how companies can transform their governance into responsible data leaders. She was recently named a “Legal Visionary” by the Los Angeles Times and was honored on the Forbes “50 over 50” list.