The Four Horsepersons of the AI Apocalypse: Part One
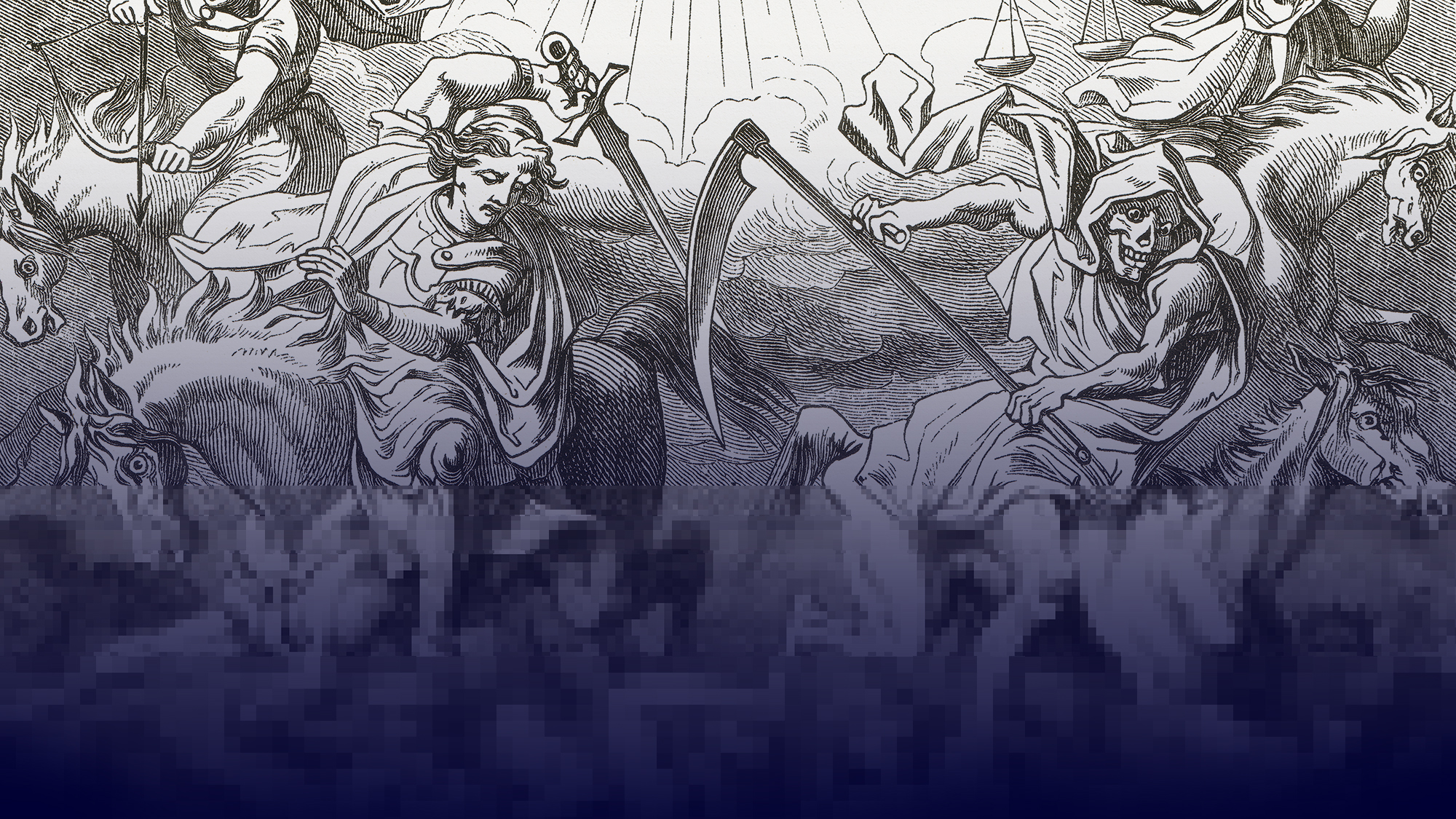
In my three decades of working with data, mathematics, computers, and the digital Bermuda Triangle that exists between them, I have come to appreciate many of the difficulties we humans face in putting technology to work. This is particularly true of the field of AI, which over the last century has endured a decades-long boom and bust cycle of hype, adoption, catastrophe, repentance and repeat.
Very few organizations manage to squeeze actual value out of AI, with many researchers pegging the success rate at five percent or less.
In most cases, people blame technology for their poor results, as if the crooked house is the fault of the hammer, not the carpenter or architect. The issue is that the technology is misapplied for the task at hand or operated without adequate skill or experience for the job. Organizations make four key errors in their attempts to do something useful with AI. If there is a 95 percent chance of getting AI wrong, one or more of these factors is likely the cause, and your project will die a slow, painful, and likely very expensive death. Here are the first two:
Horseperson #1: You Invested in Technology, but not Training
In most IT projects, the cost of buying, building and deploying new technology is far greater than data, testing and training. This cost is so common within the IT world that most of us are familiar with budgeting models that point out your error if you do not dogmatically follow this operating assumption.
Successful AI projects are the opposite. Regardless of the technologies, models or algorithms used, an AI can perform no better than the data with which it is trained. Training data is the key to getting meaningful results from AI, yet few plans and budgets reflect this reality.
Imagine trying to learn to speak Mandarin by taking classes in Spanish. Imagine trying to become a math professor not by studying one hundred math books on different math principles but instead by reading one hundred math books all on the same principle. Imagine a teacher expecting students to infer facts about a subject without ever teaching those facts. Then imagine that same teacher telling their students, “Take your best guess,” when a fact or question arises that the teacher never anticipated or considered.
These hypotheticals are a pretty close approximation to reality in the vast majority of AI projects.
The importance of training and data is dramatically underestimated, leading to exceptionally poor results. These poor results are not an anomaly, they are the predictable and inevitable result of an under-funded data engineering and training effort.
Horseperson #2: You Left Abnormal Data Out of Your Data Sets
One of the benefits of data is that, for the most part, it’s normal. By this, I mean that any data regarding a certain characteristic of a certain population follow a normal distribution. I won’t explain normal distributions here, but suffice it to say that on average, most of a population is “average,” with decreasing numbers of below- and above-outliers falling to the extremes. The further away an outlier is from the population’s “average” value, the rarer the outlier becomes.
In many AI initiatives, analysts feed their algorithm with a set of “normal” data, representing the population’s actual distribution. In this way, their AI learns what “normal” is and accurately reflects the statistical probability of a certain value showing up in the data. This approach seems eminently practical: Teach your AI what actually occurs. But is this approach really effective for what we are trying to achieve with AI? Arguably not.
Assume you were analyzing a set of data where the possible values for a property ranged from 1 to 10, with an average of 5. If it follows a normal distribution, most of the data will be at or near ‘5’, while far less of it will have values closer to 1 or 10. If you train an AI with this data, and that AI is then presented with a result of ‘5’ to evaluate, the AI should have an excellent idea of how to respond. It’s been trained what to do with a ‘5’. However, present the same AI with a value of ‘1’ or ‘10’, and the AI has been exposed to vastly fewer examples of those numbers, so has far less training on responding to these edge conditions.
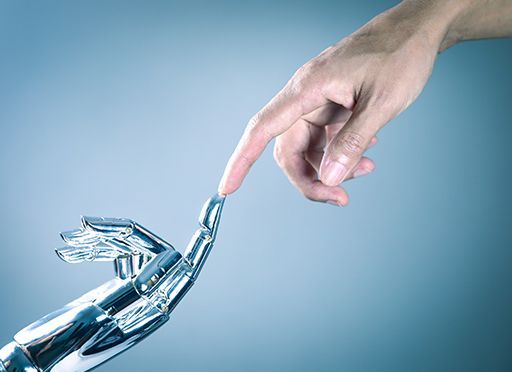
Here is the problem with the “normal” approach: knowing what to do most of the time is not what we need AI to address. In fact, basic rule-based logic is more than adequate for normal use cases.
It is the boundary situations where we need greater assistance, yet these situations are those for which we typically train AI the least, if at all.
It may be counter-intuitive, but to get AI to really be of value, we need to flip our datasets on their head, and over-represent the rare boundary cases in our datasets. In so doing, we front-load the AI’s statistical engine to know what to do when rare events occur, which is exactly when we need AI in the first place. Teaching a digital thermostat, like a NEST, that we like our house to be 70 degrees all of the time is not terribly useful. Teaching it to call the fire department when our house temperature suddenly jumps over 100 degrees is dramatically more useful when and if it occurs.
Here’s some additional reading:
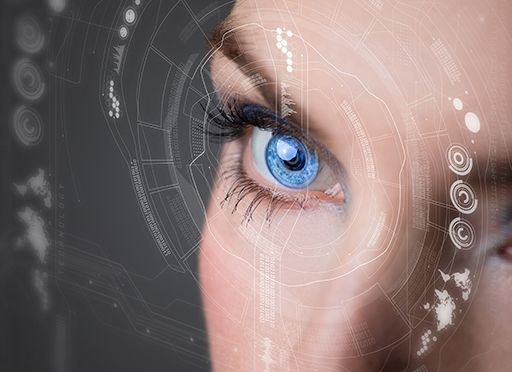
In Part Two of this article series, I examine two more “Horsepersons of the AI Apocalypse” and how to avoid them.