“This Process is Relentless and You Will Either Adapt or Die”
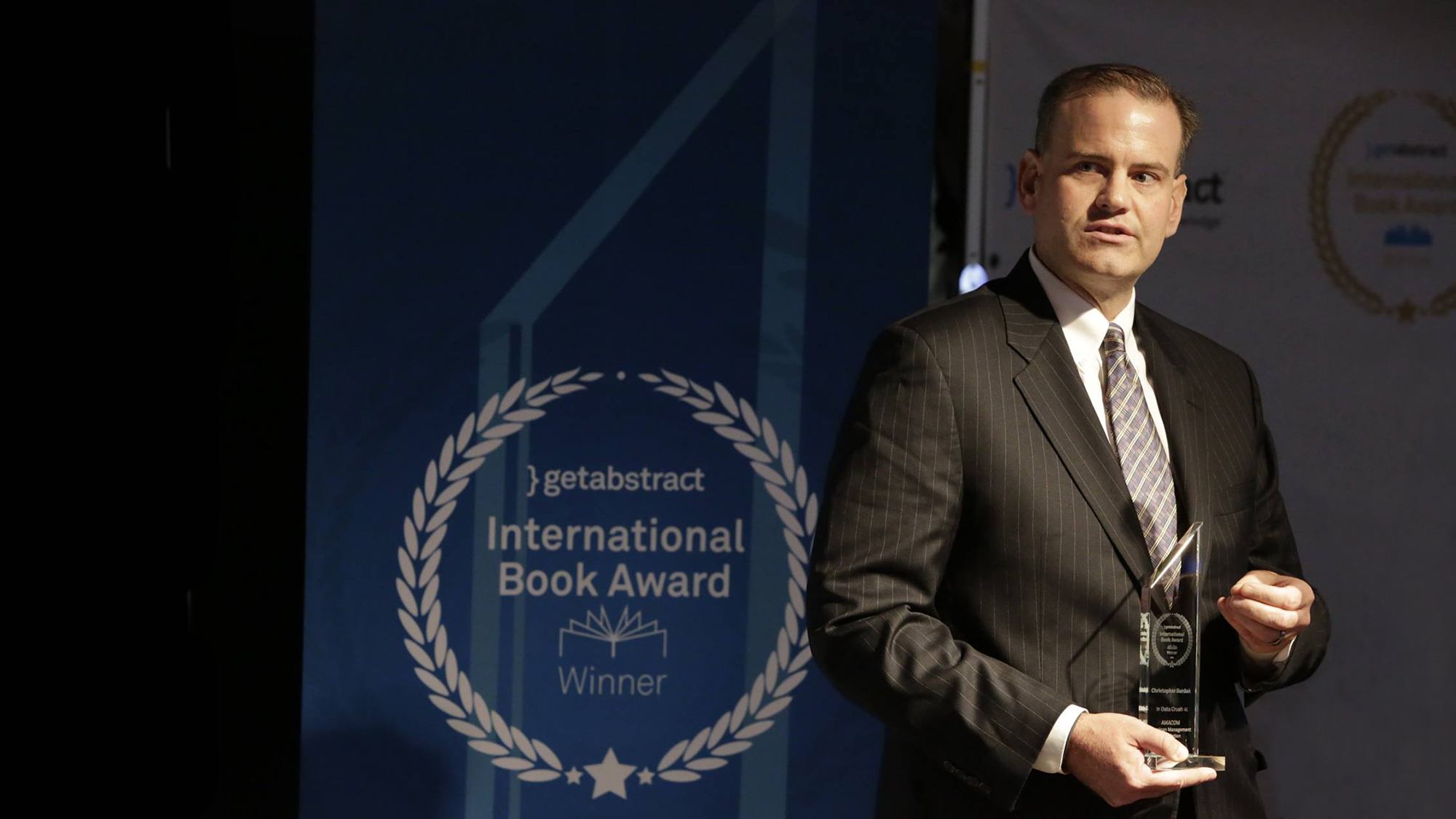
In your first book, Data Crush, a getAbstract International Book Award winner, you surveyed the coming digital transformation that we’re now in the midst of. Your latest book, The Care and Feeding of Bots, focuses on robotic process automation (RPA). What is robotic process automation and can you place it within the larger context of digital transformation for businesses?
Chris Surdak: RPA really hit its stride about eight years ago, but from a technology perspective, it goes back almost 20 years. RPA is a fancy marketing term for macros. Really, that’s what it is.
That sounds less daunting.
We also refer to this wave of technology as “intelligent automation,” which makes it sound like automation done in the past was unintelligent, which is not the case. In effect, RPA is software macros with a governance layer.
Take-Aways:
- Robotic Process Automation (RPA) involves deploying software bots to automate business tasks.
- Unlike other IT acquisitions, what makes RPA expensive is not the technology, but the talent necessary to deploy it.
- RPA can help you deliver to customers faster in the “context economy.”
- RPA, correctly implemented, leads to transformative change.
You say that RPA is probably the first step a lot of companies will take to build out their human-machine interface. I particularly liked your suggestion of starting small – learning to juggle first with red rubber balls instead of chainsaws.
Every wave of new technology generally has about a four percent to five percent success rate for early adopters. That tells you the technology works, but rare is the person who knows how to make it work. Finding, obtaining and retaining the person that knows the technology well enough to implement within that success rate is exceedingly hard, especially given the escalating global war for talent, and they’re really, really expensive. Few organizations are willing to recognize that, and most are unwilling to pay for that level of talent. It’s an interesting problem that everyone is facing right now.
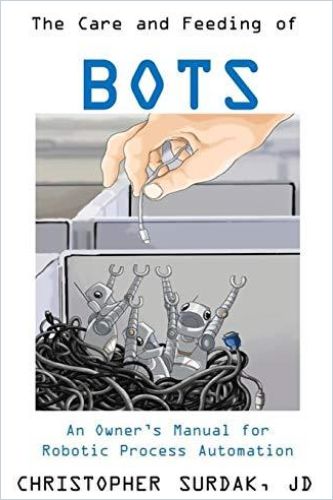
So, bots are single task oriented, right? They’re software that handles other software. What kind of tasks should businesses be thinking about digitizing through RPA?
An optimal target task for a bot is something that is highly repetitive, strictly rules-based, and you can articulate what those rules are with few, if any, exceptions. Usually it is information that crosses multiple existing platforms, so we call it “swivel-chaired.” Many times we have multiple systems in an organization and we pay a human $20 or $25 an hour to read what’s on the screen, swivel their chair, and type it into this screen over here.
This is why I don’t like the term “intelligent automation.” There’s a presumption that if a business task or business process today is performed manually, it’s inherently bad. Having people do it manually may well be the cheapest way to achieve that task.
If you have a task that’s still manual, it’s almost certainly because of some combination of what I call the Three C’s: cost, complexity and compliance.
Christopher Surdak
It’s either too costly or too complex to have automated in the past or compliance prevented me from doing so, meaning it’s a “human in the loop” kind of process; like a maker-checker process at a bank.
Is there low hanging fruit that companies are coming to you for their first automated tasks?
There is a a well-known “top 20” list of places to start. It’s usually in HR or it’s in finance. It’s things like processing invoices at the end of the month, processing resumes and applications coming into HR or automatically processing expense reports; things that have a lot of rules associated with them. There’s a lot of data coming in that needs to be assessed against those rules and then fed somewhere else down the chain. After a decade of experience with this technology it is readily apparent where to start, or rather it should be. Another challenge is most people try to automate processes end to end, and that is remarkably difficult to achieve. You’re far better off automating task by task. It’s hard to dis-aggregate the menial tasks that a knowledge worker does and the cognitive part that tells them what’s the right thing to do at a particular time. It’s easy to automate the repetitive stuff. It’s nearly impossible to fully capture the cognitive and articulate in software.
There are different kinds of bots, for instance online chatbots or apps, with different maintenance needs. Can you describe one of the heavier duty, workhorse type of bot?
Those are the ones that do something very menial, but very useful and productive. An example would be a telephone carrier that has to resolve billing issues for 20 million people every month. It’s synchronizing data from two different systems for those 20 million monthly transactions. In this instance, they created a bot that does that and freed up five hundred people to work on something else. It’s a very simple task. It’s a menial task, done a ton of times, and it’s really valuable. Because it was simple, it was rule-based. It was a very closed loop. It was an ideal instance of that form of automation.
You argue that deploying RPA only makes sense at scale. Why is that?
RPA is different from other information technology that’s come before it. And this is based upon actual experience. Every information technology that companies bought over the last 60 years has one thing in common: The cost of acquisition is greater than the cost of ownership. By that, I mean, if I’m going to use an ERP system to run all of my company’s financials, I might spend half a billion dollars to buy it and deploy it. And then I spend perhaps $20 million a year to run it. So, the cost to acquire is much higher than the cost of operation. Historically, this leads to a certain behavior where, when you want to pick the technology you’re going to use, you’re getting married to it. You’re going to be using it for the next 20 years. You put a lot of effort into vetting and choosing and picking and purchasing. You’ll have a six month long competitive bid and all this other crazy oversight stuff, because the cost of acquisition is very high. RPA is the exact opposite. The price point for buying RPA software is remarkably low. I can buy a single software license for a bot for about ten thousand dollars a year. But the people that support it are extremely expensive.
For the first time, we have a technology where the cost of acquisition is significantly less than the cost of ownership. Well, we’re not wired to work that way.
Christopher Surdak
You need to prove the value in a given use case, so people will deploy bots one at a time, two at a time. Purchasing will spend $10,000 for a software license, and that’s the cost of really looking at it, but then they have to hire an RPA engineer for $150,000 a year, and a business analyst for $75,000 a year, and a project manager for $120,000 a year, or outside consultants at $250 an hour to replace someone that makes $16 an hour doing data entry. So, if I’m only replacing that one person making $16 an hour with all of these six-figure, higher educated, hard to find people, they can’t understand why they can’t get a positive ROI.
But if you spin that out to the long-term view –
So that’s what I mean by the “20 bot barrier.” That project manager, engineer and business analyst that I hired to come up with the first bot, they can do 20 bots easily. That’s how I keep them fully utilized, and this replaces 20 people that make $16 an hour. I can now cover the cost of those three people. My software cost is still relatively low. It’s the human cost, the startup cost of the people that support it that’s really high.
I see. That makes sense.
But usually the CFO doesn’t like the math, the CEO doesn’t like that math, the controller doesn’t like that math because that’s not how the software company sold them on the idea. They say “Oh, it’s only ten thousand bucks for the software.” But everything else is wickedly expensive.
And it makes sense too that you would need to hire someone who not only can take care of the bots, but also someone who’s looking forward to the future because, just on the face of it, some kind of software infrastructure that lasts 20 years isn’t going to cut it at today’s pace of change. You emphasize a mindset shift in your book Jerk, and you also talk about how going through this automation process forces companies to change and to be more nimble because they’re leaving incremental progress behind. Can you elaborate on this?
Incrementalism is a disease that we’ve invented and embraced for at least 40 years. It’s how I started, you know, process improvement and statistical process control and Kaizen are all about incremental improvement. Organizations embrace incremental change because it’s easy to fake, and they don’t like major change because it’s risky. I call it “Snickering.” You know, the Snickers bars that you have at Halloween? They get smaller and smaller every year. They don’t make them better, they don’t make them cheaper. They just make them two percent smaller, hoping you don’t notice. And that’s their two percent improvement. Eventually, Snickering leads to, I mean, they’re like the size of a fingernail now, right? And when they make them two percent smaller next year, they will be so small you won’t be able to put a peanut in it any longer and then it becomes a Milky Way bar. So Snickering is this relentless application of pretend-improvement that organizations suffer from, but it’s perceived to be less risky.
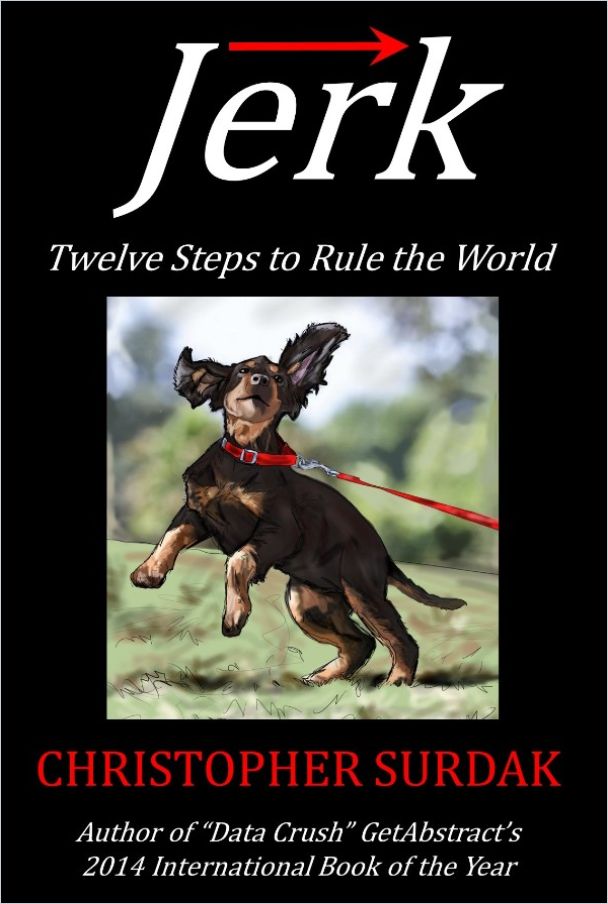
How has going through this process transformed organizations from this kind of incremental thinking into a more transformative way of thinking about business?
Part of the challenge is what I call “diet-pilling.” I want to lose weight, but I don’t want to stop eating pizza. I want a different outcome without the effort that comes with change. RPA vendors go to these companies and say, “Hey, just buy this really cheap software. Take a week to implement it, and you’re going to save all this money.” And then you, as CFO, can get your bonus because you hit some arbitrary financial target. Sounds compelling. It’s like buying a diet pill on late night TV. Is it really going to work? No. But the psychology behind it is well established. That doesn’t lead to breakthrough results. If the design that you end up with in any kind of automation process is the same as what you thought it would be at the beginning, then you’ve done something wrong. The act of automating a process changes the process that’s being automated.
Unintended consequences, having to make more radical changes as you go along, is the indication that you’re heading in the right direction. Without that, what was the point of automating?
Christopher Surdak
One of your main points in Jerk is how agile companies disrupt incumbent organizations because they’re super-focused on customizing the customer experience and creating that “market of one.” And I’m wondering does RPA fit in with that goal?
RPA has fantastic ROT: Return on Time. In today’s world you have 30 seconds or less to give me what I want or I’m gone and I’m never coming back. RPA, in being able to eliminate the expenditure of time in business processes, can be phenomenal in meeting customer needs from that perspective: keeping them engaged, identifying a market of one and meeting those needs as quickly as possible.
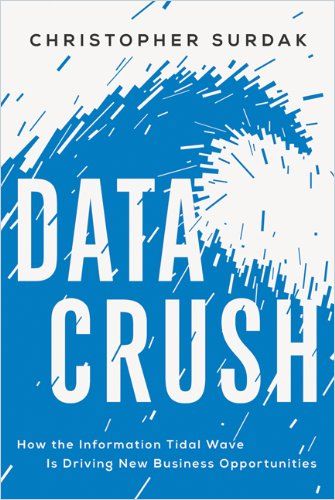
You talk about this – contextualization – as a major trend in digital transformation in Data Crush.
Despite losing a quarter of a trillion dollars of value recently, Facebook is a successful example of a context engine. They live in the context economy. What’s the context economy? Where I am in space and time matters and what I want in space and time matters. If you take a demand in space and time and match it with the satisfaction of that demand in space and time, you create value. That’s Uber, right? Here’s someone who needs a ride. Here’s a car that’s able to give him a ride. Putting the two together in space and time creates value.
The context economy is “I’m expecting you to know me and my needs intimately,” on the verge of being creepy. I literally call it “creepy analytics.”
Christopher Surdak
You know I’m going to drive by the same Starbucks I always drive by in the next 20 minutes. You know my favorite drink, and you’re going to give me an instant coupon if I stop by in the next four minutes. It’s creepy, but I love it. Now, if you did the exact same thing to my daughter: too creepy, I don’t love it. The challenge is our “creepy line” is also contextual. I love that you know me that well, but in this other context, it terrifies me. So that’s context.
That’s another fascinating topic unto itself.
The most valuable companies in the world right now are context engines. If you go to Facebook, here’s a picture of a black cat. Could be anybody’s cat, but I say, oh no, that’s Karen’s mom’s cat. I added context. Now, who added the context? Not Facebook, me: I did the work. Now, it’s Karen’s mom’s black cat. Oh, and next week is its birthday. And Karen’s mom would really like to have a diamond-studded collar for the cat. And here’s a 20 percent off coupon for it. That’s contextification. You and I, in our participation on those platforms, manufacture contextual-based wealth free of charge that they then turn into a trillion-dollar market capitalization. That’s contextification.
Personally, that sounds creepy too.
Yeah, if you’re doing it right, we’re literally right on your “creepy line.” And every once in a while, I help write these algorithms that will slightly go over what we think your creepy line is just to know exactly where it is. And then we’ll pull back with a cool coupon or a cool post or something and make you forget that we just freaked you out.
(Laughs) Okay, good to know.
That’s how this works.
Okay, so the most crucial part of utilizing this avalanche of data is analyzing it, right?
Not necessarily. I’m working on my next book, Data Diabetes, which describes what organizations are now suffering, because they have an super-abundance of data and an overabundance of analysis paralysis; a complete inability to act upon it. Like a diabetic who feels hungry or thirsty, and they drink a sugary soda, hoping that it makes them feel better, it actually makes them sicker. Most organizations that are older than 20 years have not fundamentally changed their business processes for those 20 years; they’ve just been Snickering.
Think about 20 years ago, you used dial up on AOL to get on the internet. You had a Nokia phone that would only let you text. There was no such thing as an app. There was no such thing as social media. So, your business processes were designed to metabolize data at those rates. 56k modem rates.
Christopher Surdak
Today’s world operates with a metabolism of broadband, 4K video on your phone. Now companies are “data diabetic.” They’re getting swamped by this massive amount of Big Gulp-like, sugary data, that they cannot properly digest, making them diabetic. They get frozen by their inability to make decisions. They have this mindset of “if I had just a little bit more data, a little bit different data, a little bit better data, then I’ll decide.” Whereas a world of appification and contextification requires that we make decisions instantaneously, and in fact, we get humans out of the loop of decision making. We’re the diseased organ in the body of the company, preventing it from being healthy.
Your book sounds really interesting. I’m always looking for ways to write about how leaders can use AI to enhance their decisions. But the idea of removing them completely from the decision loop is an unexpected recommendation. So, I assume you wouldn’t want to take them completely out, right?
You want them out of the process; you want them on top of the process. I was working on some research on what I call the cybernetic organization, what companies will look like by 2030 – because this process is relentless and you will either adapt or die as a company. Covid accelerated that. In cybernetic organizations, we as humans are what slows everything down. That relentless demand for context and appification means we have to go faster and faster. We’ve got to get out of the process and we have to then monitor the process. We’re observing it and we’re looking for things to potentially go wrong.
In addition to financial, design, operational and technical problems deploying bots, you talk about governance problems. Is this what you mean?
Yes, absolutely. And I love the operational ones. I had a client that designed a bot and it was going to work twenty four hours a day. It was going to save ridiculous amounts of money. But no one told them that the systems that the bot had to interact with had a 12 hour overnight production freeze every night. So, if you thought to ask the question, you would have known right off. And in fact, that made it not a viable, positive ROI implementation.
So, it comes back to finding the right talent. Bots are not “set it and forget it” solutions. In your book, you talk about “achievers” that create an ecosystem supporting thousands of bot operations. To recap, that ecosystem looks like you have a Chief Bot Officer, you have engineers who are able to deploy and maintain that layer of governance, overseeing the automated tasks. Any other major structural changes come to mind? Or do you need to wait to see what you get back to adjust?
Organizations have been heavily leveraging rules to dictate what people should do. This is part of what I call the “Analog Trinity,” of Bureaucracy, Process and Rules, which capitalists used to create and manage capital wealth for the last 250 years. If you look at the typical governance of a company, it’s going to be a policy manual 700 pages thick; it’s like the U.S. tax code. Every time we find our rules are inadequate in some way, we put in more and more rules to give more and more guidance to everyone, to make sure they follow the rules. To the point where it’s impossible to follow.
The illusion and delusion of control comes from an ever-deepening rulebook that becomes so big nobody can actually follow it. We can automate the hell out of rules. But the question is, is it a good thing to automate all those rules?
Christopher Surdak
The rules we implement are our best approximation of the right thing to do at a particular time. The world isn’t that static. The world is dynamic. So the best approximation of the right rule to follow 20 years ago is probably no longer valid today. But if I’ve encoded it into how I operate, I’m forcing myself to become obsolete. What’s better than this and actually global, is principles: Do the right thing, the customer comes first. There’s a handful of principles I can use, and this is where machine learning and artificial intelligence comes in. Don’t give me ten thousand rules that I need to follow and guarantee, and all those middle managers that guarantee you follow the rules whether it’s the right answer or not. The principles can guide us. Then I need two managers, not 20,000. Then we qualitatively reassess in the given situation: did I follow the principles correctly, given the unforeseen and unforeseeable circumstances that just occurred?
So instead of making rules, then amending the rules due to a circumstance, and amending the amendment due to later circumstances, sort of recreating bureaucracy digitally –
Exactly. The level of complexity in our society has grown to the point we’re crushing ourselves under the rules-based approach. Inevitably, the principle-based approach has to replace it. We’re trying to replicate our 250-year-old capitalist thinking, analog thinking, I call it in Jerk. We’re trying to encode that in more rules and more automation and call it digital transformation. That’s like taking a worm and stapling wings on it and calling it a butterfly. It feels great, but it’s not going to work.
About the Author
Christopher Surdak is an industry-recognized expert in mobility, social media and analytics, big data, information security, regulatory compliance, artificial intelligence and cloud computing with over 25 years of experience. He is the author of the 2014 getAbstract International Book Award winning Data Crush, as well as Jerk and The Care and Feeding of Bots.
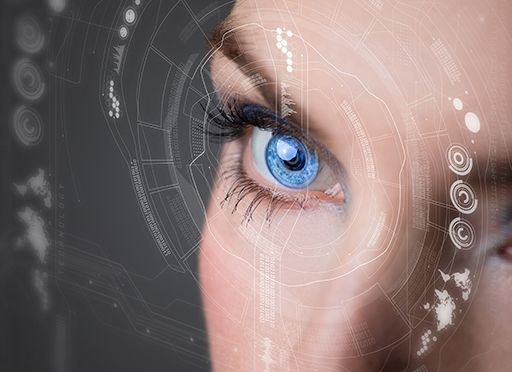
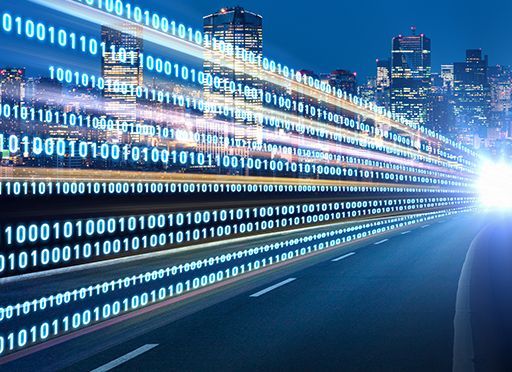