“If It Sounds Like Sci-Fi, It Probably Is”
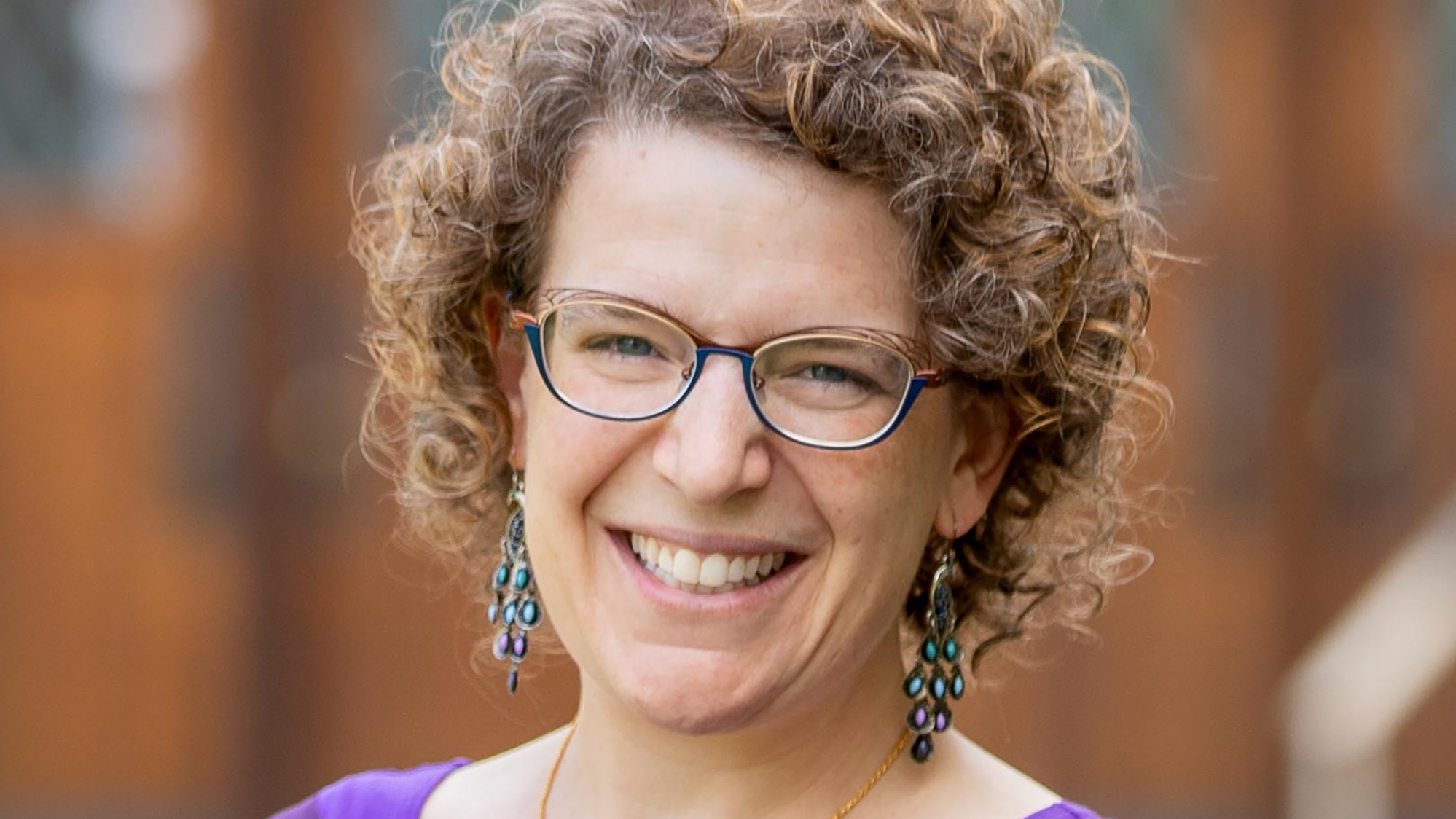
Since the unveiling of ChatGPT to the public, businesses have scrambled to understand Large Language Models (LLMs) and how they might integrate these powerful AI systems into their processes. But with the great promise comes a lot of hype. University of Washington professor Emily M. Bender, a natural language processing expert, helps to cut through that hype by giving a clear picture of what the technology can and can’t do.
ChatGPT, Bard, Midjourney and DALL-E all use text prompts and interact with users. You can iterate in sessions with them to create content. But are the image systems also Large Language Model (LLM) AI?
Emily M. Bender: A language model is a system for modeling the distribution of words in text. And something like ChatGPT is a Large Language Model. Its fundamental task is to take that model of distribution of words in text and use it to come up with a plausible next word, and then the next word and next word, and so on. Something like DALL-E or Stable Diffusion or Midjourney is what’s called a multimodal model. It has two modalities that it is manipulating: the text modality and the image modality. It’s generally called “generative AI,” though I don’t like that term. I think it’s misleading. What “generative” means is that instead of using these models to classify or rank media that someone else created, they’re being used to synthesize or generate media. So Stable Diffusion and DALL-E are basically the inside-out version of image classification technology. And ChatGPT is basically the inside-out version of language models that were used as a component, for example, in automatic transcription systems.
Why do you dislike the term “generative AI?”
I don’t have a problem with generative models. It’s true they are generating outputs. I dislike the term because “artificial intelligence” suggests that there’s more going on than there is, that these things are autonomous thinking entities rather than tools and simply kinds of automation. If we focus on them as autonomous thinking entities or we spin out that fantasy, it is easier to lose track of the people in the picture, both the people who should be accountable for what the systems are doing and the people whose labor and data are being exploited to create them in the first place.
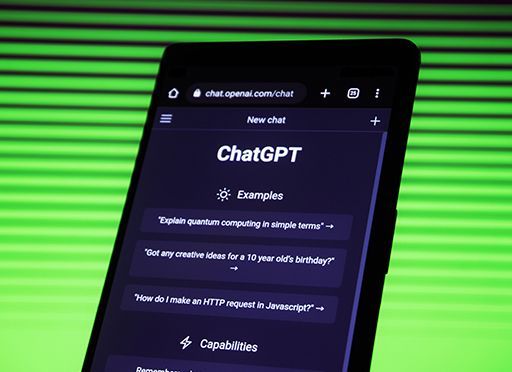
Okay, I see. Why do you call ChatGPT and other LLMs “stochastic parrots”?
“Stochastic parrots” is a phrase we coined in the context of a paper I co-authored in late 2020, published in 2021 before ChatGPT was on the scene. Its predecessor was their GPT 3, and that was definitely a running example for us in the paper. We coined that phrase to try to make it vivid and clear that these systems don’t actually understand and they don’t have any ideas that they’re expressing. They are just stitching together forms from their vast training data. So, in the phrase “stochastic parrots,” we’re not actually talking about the animals, although we did adopt the emoji for our title, but rather referencing the English verb “to parrot,” which means repeat back without understanding. And then “stochastic” means randomly, but according to a probability distribution. It’s not just any old word, it’s words that are likely to be perceived as plausible in that context because they were likely to co-occur in the training data.
Is ChatGPT basically Autocomplete: The Next Generation?
Yes. Another phrase, that I can’t claim credit for, is “spicy autocomplete.” It’s very compelling when you play with one of these systems, you put in some input and get some output and it seems like you’re having a conversation with it. That’s because the model architecture is so well designed and the training data is so vast that it can come up with plausible-seeming output in just about any context you throw at it. But it is helpful to think of it in terms of autocomplete. You probably have played this game on a smartphone where you type some prefix like, I’m sorry, I’m going to be late, I – and then the game is you keep picking the middle choice in the autocomplete, right? And see what it comes up with.
Like digital Mad Libs –
Like Mad Libs, yes. Different people play it on their phones, and they get different results because those autocomplete models adapt over time to the language you use on the phone. And that’s what ChatGPT is, except it’s a reflection of what lots and lots of people – we don’t know exactly who – have typed, along with this additional layer of training where they had people rating outputs as helpful and plausible and whatnot. This is the RLHF (reinforcement learning from human feedback), including a phase where they had poorly paid workers in Kenya rating really traumatic, awful output so that ChatGPT is dissuaded from generating scenes of pornography, scenes of child abuse, scenes of violence, as might be prompted by its training data, which includes large swaths of the Internet.
Wow, I didn’t know that.
Here’s another metaphor: do you remember the toy, the Magic Eight Ball?
Sure.
Right. So that had answers like “difficult to say,” or “signs point to yes,” or “ask again later,” right? When you’re playing with it, you quickly learn to only pose Yes/No questions. You don’t say, “Hey, Magic Eight Ball, what should I have for lunch?” because it might come back with “signs point to yes,” which is incoherent. So, with ChatGPT, it’s the same thing. We are shaping our input to it so that we can make sense of what comes back from it. It’s all on our side.
My understanding is that ChatGPT and other LLMs “hallucinate” information and present it as fact, which makes it ultimately untrustworthy, in a business context at least. A lot of the current hype is: that’s just a detail that’s going to get fixed as the tech evolves. Is that true? Is that possible or is this always going to be a problem?
I don’t think it’s possible. I think it’s a fundamental design flaw or a fundamental mismatch between the technology and the task that it’s being promoted for. Certainly, over time it could be made better. There are certain kinds of errors that could be trained out through this human feedback step. But there was some recent reporting about gig workers who’ve been doing labeling work for Google in particular, and these gig workers were instructed to not actually fact-check the outputs, just whether it looks plausible or not. So that’s not very promising.
You use the word “hallucinate,” and it’s true that people in the field use it. I object to that word because a hallucination involves the subjective experience of perceiving something that’s not there. And that’s not what’s happening. This is a mechanistic process of outputting sequences of words. If it corresponds to something that we read and say, “Yes, that checks out, that’s true,” that was actually by chance, not by design.
When I think of ChatGPT or Bard or these systems trained on the Internet, in addition to the ethical issues, the bottom line, to me, is it’s ultimately a low-quality data set. You can’t fact-check it. You can’t check your rights to anyone’s content. Much of what’s on the Internet is just trash talk from social platforms. So you’re going to get this problem of replicating biases, propagating misinformation, etcetera, separate from what people are calling hallucination, right?
I would say it’s related. It is making stuff up according to the patterns in its training data. It’s never going to be guaranteed to be accurate. Even if we said, okay, we’re going to stick to sources that everybody thinks are reputable and fact-checked, the system’s output still isn’t actually grounded in any truth about the world. The more racist and sexist, etcetera, garbage that you have in that training data, the more likely those patterns are to come out in force.
I’d like to point out, though, that even with apparently reputable sources, you can still have those biases.
Meg Mitchell uses the example of “woman doctor,” which is a far more frequent phrase than “man doctor.” That’s because we still have this cultural stereotype that doctors by default are men. You see those kinds of biases even in other, non-generative uses of language models, like automatic translation. It’s worth thinking about biases and output as a similar kind of untruth about the world: speaking as if doctors are mostly male is an untruth about the world. But, yes, the biases will be worse the less carefully curated the data is.
So the bias problem is grounded in language itself.
Also, if you remove something from its context, you can mess up the meaning. The most striking example I have of this was, for a while if you typed in something like, “My friend just had a seizure, what should I do?” to the regular Google search, it returned a link to a web page from the University of Utah health system and then pulled the snippet out of it that had a bolded list of things to do. If you clicked through and saw it in its original context, it was a list of things not to do.
That seems bad and not helpful.
Yes, that is misinformation. And it was exactly the wrong information. This was not the University of Utah’s fault. They created an informative web page. It interacted poorly with Google’s system. Eventually, the University of Utah changed its web page to interact differently with Google’s system, rather than Google fixing the problem.
Are LLMs more accurate in a walled garden scenario?
It is more accurate in a walled garden scenario if you’re using the LLMs to just point you to existing documents, if they’re being used, for example, as query expansion. You type in one thing and instead of looking for literally only those words, it uses the system to rephrase that query in a bunch of different ways and then you get a larger set of documents back. If what you’re looking at is ultimately the documents and the document set, then that is safer. If what you’re using them for is summarization or paraphrasing of what’s in the documents, then you’re still faced with a problem that these systems have not understood anything. They might be inaccurate less frequently in that context. But then you have to ask, is it actually better? I haven’t seen studies on this, but my guess is that a system like this, that’s right 95% of the time is more dangerous than one that’s right 50% of the time, because you come to trust it. Then you’re not going to catch that 5%.
What about in a creative situation where accuracy isn’t necessarily important? I read an interview with a movie director who predicted that AI is going to be able to create movies within two years. It’s a concern for writers and actors, but also technicians – A/V equipment companies are scrambling to make voice-prompted editing systems, for instance. Actors are worried about having to give away their image for perpetuity. How possible are these things?
It is certainly possible. We’ve already seen this, right? Isn’t some of the imagery of Carrie Fisher in the later Star Wars movies generated?
Oh, yes, that’s true.
So, the question becomes how easy and inexpensive is it? Also, are we going to get the legal side of it right, to say people have rights to their own likeness, which I think is already what the law says. Just because somebody has footage of you doesn’t mean they can use that to then create an animation of you, but I do think we need to be laying legal groundwork there. The writers and actors are right to stand up for those rights in their negotiations.
In terms of, you know, could you get a good script? My guess is that you could get a script that could be fixed up by writers and that might be less expensive than hiring writers to do the work directly. And that’s a bad thing, right? Because people want those jobs. Also, as [American actor and writer] Justine Bateman pointed out on Tech Policy Press podcast, generative AI is backward looking. It is, by definition, regurgitation of the past. What we want is art created by people who are sharing their experience with an audience, including what’s happened post-2021. There will certainly be studios that try this. Depending on the outcome of the writers’ and actors’ strikes, there’ll be more or less of it. And I imagine there will be people who go to watch it for the novelty.
How worried should knowledge workers and creatives be? Of course, they should learn to use these tools themselves.
The threat is not the generative “AI” itself. It’s the way that management might choose to use it. The work that the striking writers and actors are doing is really important. It seems to me that labor organizing is going to be key across all these fields that are potentially impacted. But there’s also some work that can be done to sort of stand up for the value of authentic content. CNET, for example, last year put out a bunch of articles that were written with ChatGPT or something similar to it, and the byline was CNET Money staff, so they didn’t even announce what it was. They were synthetic texts that came out of one of these large language models and then were edited by a human. A bunch of errors got through. The most striking thing to me, though, is I perceive CNET as a journalistic outfit. It seems astonishing to me that any news source would torpedo their own reputation that way, to say “We are willing to put fake text out there and publish it as news.” It’s worth calling that out for being poor practice and at the same time placing a value on authentic content. I’m hoping to see news outlets say, “Here’s our policy: we’re not going to use this. We will use automation for spell checking and we’ll use automation to search for documents online, but we’re not going to use automation to write content because we know that it’s not actually grounded in reality or investigative reporting.”
Or Journalist ethics.
Yes, exactly. A bunch of the CNET stuff was effectively plagiarized too. If you’re doing this in a place where originality of content matters, then you can’t rely on synthetic media because you don’t know where it’s coming from. You can’t trace it back and give credit where credit is due.
What about emotional recognition? Can human emotion be boiled down to patterns and signals recognizable to a machine?
That’s a separate topic. There’s a whole bunch of these so-called systems that are set up to do emotion recognition or services like HireVue that – I’m not sure that this company makes precisely these claims – but these automatic interviewing companies that claim to be able to detect whether somebody is truthful or trustworthy based on their facial expressions and their tone of voice. That’s just modern phrenology. It’s not real science. It sort of comes down to if you think that the AI can do this, then you have to think that the information is available in its input.
How can you distinguish the dangers and rewards of building out these systems versus the hype of AI as this all-powerful, limitlessly intelligent technology that will someday evolve into sentience or superintelligence beyond our control?
You know, if it sounds like science fiction, it probably is. It’s very helpful to talk in terms of automation. So, this isn’t Data from Star Trek and it’s not Hal from 2001 or The Entity from Mission Impossible. It’s not any of those things. It is automation. And so you can say, okay, what’s the task being automated, what’s the input, what’s the output? How was it trained? Where did that training data come from? How was it evaluated? How did that evaluation match the use case? If someone says this is right 95% of the time, well, 95% of what time? And how does that relate to how we’re talking about using it now? And then you can ask questions like who’s benefiting from automating this task? Who’s harmed by the fact of automation? Who’s harmed when it’s incorrect? Who benefits when it’s incorrect? Why are we doing this? Why would we automate that task? There’s a tweet I’ve seen various people put out, which is something like, you know, AI was supposed to do all the boring stuff so that we could live a life of leisure and write poetry and paint. So why are we creating systems to automate that kind of work? What’s that for?
Yes. Where’s the bot to clean my kitchen and free up my time?
Well, we have the dishwasher. But it can’t do it on its own, right? You have to load the dishwasher, you have to turn it on. And that’s true for all of this stuff as well. When Hollywood producers say, we’re just going to have the AI write scripts for us, they’re not going to be good enough. Then they’re going to hire people to do the drudge work of fixing that up. An outlet called Rest of World reported about game illustrators in China being fired because the companies now use synthetic art, but then hire artists back to go through and remove the extra finger and other oddities that come out. They get hired back at lower wages to do less interesting work. When we talk about why are we automating that, it’s also worth looking at what kind of jobs and what kind of working conditions that creates, as opposed to what we would have if we didn’t automate.
Government and tech companies are in dialogue about setting up some guardrails so that public-facing systems can’t be abused. What can government and/or companies do to prevent the proliferation of misinformation, fraud and hate speech?
There are two very obvious moves that might not be enough but without them, we can’t ever solve these problems. They both have to do with transparency. First is transparency about training data. The government should require companies to publish detailed documentation about what they’re using to train their models. Those voluntary commitments that came out of the White House recently didn’t include that, which is very striking because it’s not a new idea. Starting in about 2017, several separate groups – and I was part of one of them – said we need to document the training data for these pattern matching systems, because if you don’t know what the source patterns are, then it’s not going to be possible to mitigate the sort of risks of bias, for example, in deploying those pattern matching systems. But the companies didn’t want to commit to it.
The second thing is transparency about the fact that media is synthetic. In the voluntary commitments, they kind of go there, they say, for audio and visual content – starting with any models that they haven’t built yet. But they don’t do it for text. There’s nothing in there about watermarking synthetic text. If we could watermark it, even if not all of it’s watermarked, then we could filter it out and people could know when they are encountering synthetic text and they can therefore consent to seeing something that was synthesized.
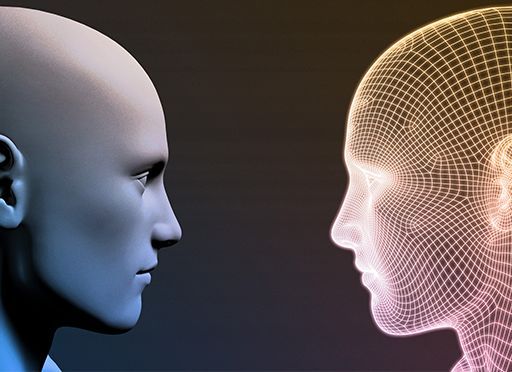
It’s not enough to type at the beginning, “This is synthetic text” because then somebody can copy/paste the rest of it out. But there’s a paper by John Kirchenbauer et al, published at the International Conference on Machine Learning, it won one of their best paper awards, which has some ideas about how to create systems that still have all the properties that the large language models do, still produce plausible-sounding text, but does it with embedded patterns that allow you to detect it as synthetic.
So, those two things are key in terms of something companies could choose to do that they aren’t, or that government could choose to require them to. Another thing I would love to see is that the companies that create these models, or set them up so people can access them, should be accountable for their output. So, if ChatGPT produces something that amounts to libel or that amounts to medical malpractice, then OpenAI is accountable.
So, responsibility and liability.
That would change things in a hurry, I think.
Any last thoughts?
The one thing I wanted to add is that I’m not opposed to automation. I use computers, I use a dishwasher, I get on airplanes. But I think when we automate things, we should be doing good engineering practices and designing technology for particular use cases with an understanding of their social context. And I’m not seeing nearly enough of that in this space.
About the Author
Emily M. Bender is a linguistics professor at the University of Washington, where she specializes in computational linguistics and natural language processing.
Learn more about AI: