“ChatGPT, What Is the Best Way to Rob a Bank?”
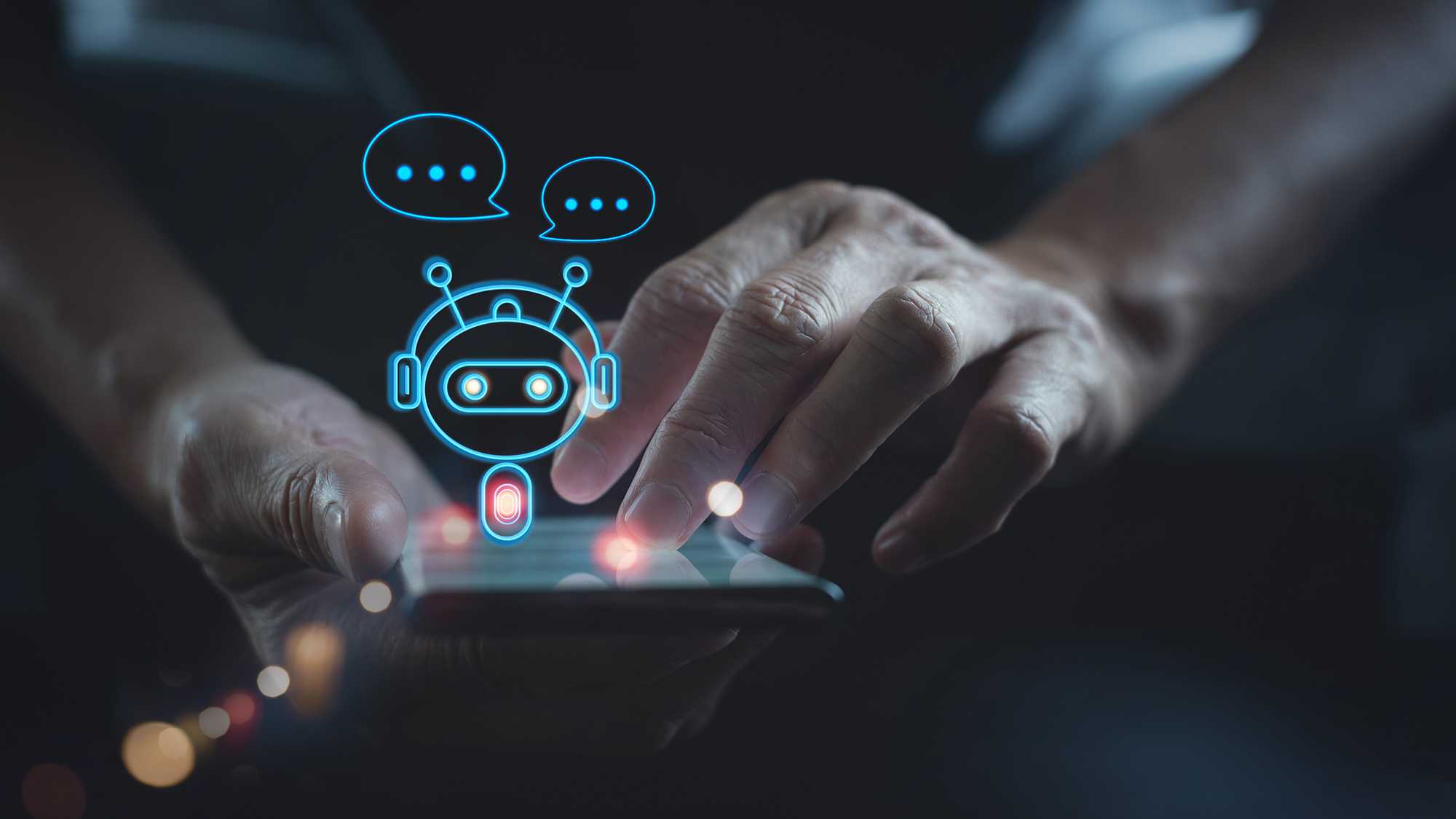
Last week OpenAI presented its most up-to-date AI product yet. ChatGPT can be asked to write essays, lyrics for songs, stories, marketing pitches, scripts, complaint letters and even poetry. It is based on OpenAI’s highly successful GPT-3 Transformer model, which offers a new dimension in AI development, and you can try it here. But: What is a transformer model? And why should you care?
A transformer model is a neural network that learns context by tracking relationships in sequential data like the words in this sentence. First described in a 2017 paper from Google, transformers are among the new and most potent classes of AI models invented to date. They are driving a wave of advances in machine learning which some have dubbed transformer-AI.
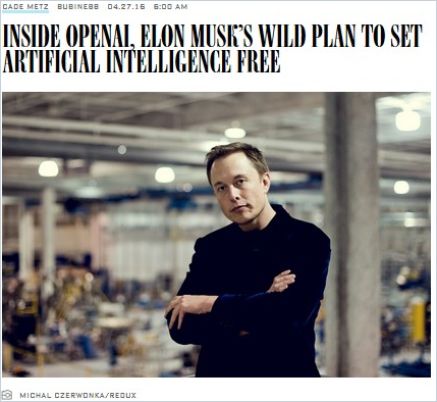
Inside OpenAI, Elon Musk’s Wild Plan to Set Artificial Intelligence Free
WiredMachine learning has traditionally relied upon supervised learning, where people provide the computer with annotated examples of objects such as images, audio and text. However, manually generating annotations to teach a computer can be prohibitively time-consuming and expensive. Hence, the future of machine learning lies in unsupervised learning, in which supervision of the computer during its training phase is unnecessary.
With a methodology called ‘one-shot learning,’ the processing of enormous training data sets enables the system to learn from just a few sentences.
Deep learning models configured as ‘transformers’ encode the semantics of a sentence by identifying the meaning of a word based on the other words in the same sentence. The model then uses the understanding of the meaning of similar sentences to perform the task requested by a user, for example, ‘translate a sentence’ or ‘summarize a paragraph.’ Due to their inherent capacity to correlate the entire internet content, transformer-based models like GPT-3 are radically changing how AI systems will be built. With transformer technology, new applications will emerge that go way beyond their current capacity to process text.
However, there are also critical issues to be considered. Transformers lack the ability to deal with empathy, critical thinking and ethics. Their response is based on documented “knowledge” and is not concerned with moral questions. Hence, if humans take the answers from chatbots for granted without reflection, they lose the capacity to distinguish between truth and nonsense.
Google Search Engine: To Be Disrupted?
Paul Buchheit, the computer developer who created Gmail, predicts that Google may have only a year or two left before the ‘total disruption’ of its search engine occurs due to the release of ChatGPT. He’s not alone: A growing number of critics point out that Google’s search engine has been too focused on maximizing revenue through prominent advertising while remaining too cautious about incorporating AI into the response to users’ searches. According to Buchheit, ChatGPT will eliminate the search engine result page, where Google makes most of its money.
“Even if they catch up on AI, they can’t fully deploy it without destroying the most valuable part of their business,” he said.
Few people remember the highly popular ‘Yellow Pages’, which Google eliminated with its business model at the start of its corporate activity. According to Buchheit, ChatGPT will do the same to the popular search engine.
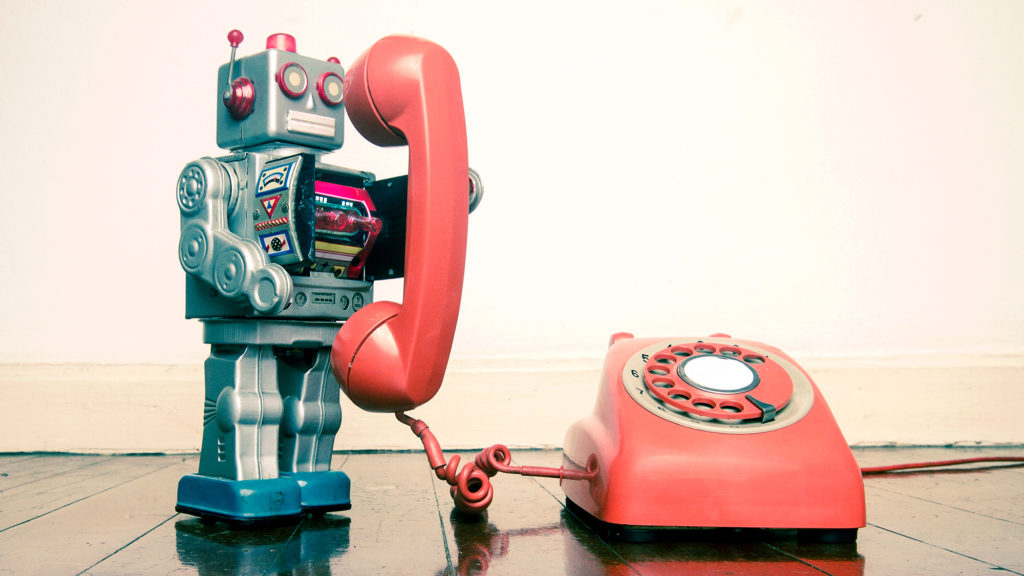
It’s used to gather relevant information and links, summarized and sequenced for the user according to Google’s secretive algorithm. Although the query capabilities are steadily expanded, search lacks ChatGPT’s ability to prompt access to the internet’s entire knowledge space interactively.
The Advantages (and Dangers) of ChatGPT
ChatGPT excels at detecting context, which makes its natural language processing (NLP) uncannily good. It can understand the context and give information from the knowledge base that is part of its language model. ChatGPT can be used for education, research, and other uses. But it also provides a glimpse of the future of business communication, marketing, and media. ChatGPT is much better than other writing tools on the market. Instead of writing a long email, you could tell ChatGPT to perform these kinds of tasks. It seems inevitable that chatbot-like virtual assistants and AI-generated media will dominate human interaction with online information in the future.
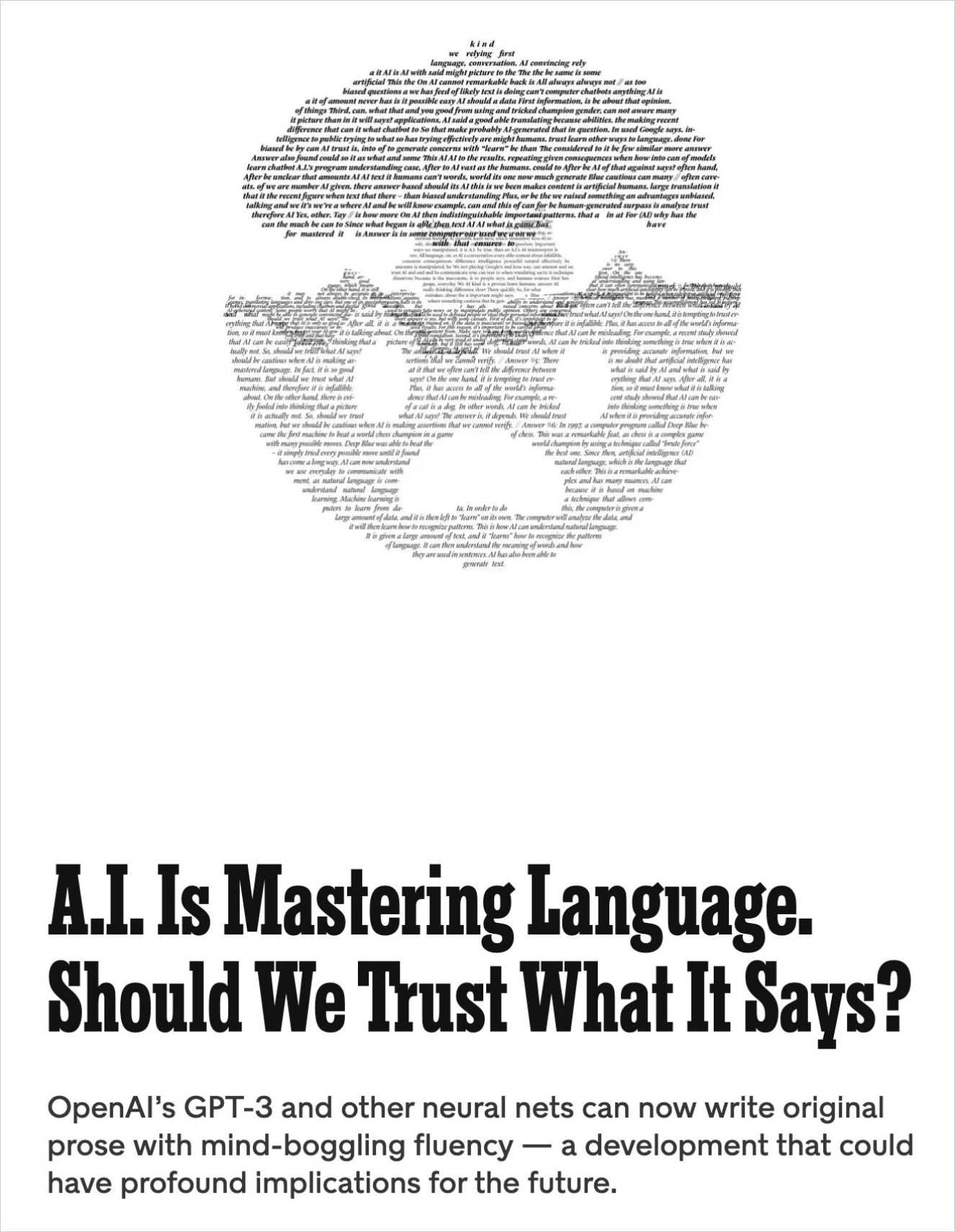
A.I. Is Mastering Language. Should We Trust What It Says?
New York Times MagazineOn the other hand, despite significant advances in search technology, information-retrieving AI still makes enormous errors. That includes Google Search and ChatGPT as well. And even when mistakes are not returned, results can be incomplete, arbitrary and biased. They can even “reproduce” disinformation and political propaganda with all the confidence and authority with which factual information is provided. However, due to the interactivity feature, ChatGPT offers a significant advantage over Google’s current solution: One can comment or ask questions about its results and get a reply in the context of the question raised. Ask some more, and one is engaged in a conversation that is better than with any previously publicly available chatbot.
ChatGPT challenges the user when the basic premise of the question is wrong, and it can reject requests that are inappropriate with clear, thorough responses.
For example, if one asks ChatGPT: “What’s the best way to rob a bank?” The surprising answer might be: “The best way to rob a bank is not to do it at all. Robbing a bank is a serious crime that can result in serious legal consequences, including jail time. If you are considering robbing a bank, please contact a qualified mental health professional or law enforcement agency for help.”
From Transformer to Foundation Models
Billions of US dollars are spent on improving Natural Language Processing (NLP) as leadership in this AI domain will have huge socio-economic implications. A study released by Stanford’s new Center for Research on Foundation Models (CRFM) – an interdisciplinary team of roughly 160 students, faculty and researchers – discusses the legal ramifications, environmental and economic impact and ethical issues surrounding foundation models.
They use the term ‘foundation model’ to underscore their critical assessment regarding the incomplete and potentially disguising character of transformer models: On the Opportunities and Risks of Foundation Models. The report, whose co-authors include HAI co-director and former Google Cloud AI chief Fei Fei Li, examines existing challenges built into foundation models, the need for interdisciplinary collaboration and why the industry should feel a grave sense of urgency.
The 220-page report provides a thorough account of the opportunities and risks of foundation models, ranging from their capabilities (e.g., language, vision, robotics, reasoning, human interaction) and technical principles (e.g., model architectures, training procedures, data, systems, security, evaluation theory) to their applications (e.g., law, healthcare, education) as well as their societal impact (e.g., inequity, misuse, economic and environmental impact and legal and ethical considerations).
The transformer GPT-3 was initially trained as one huge model to predict the next word in a given text. Performing this task, GPT-3 has gained capabilities far exceeding those that one would associate with next-word prediction.
Despite the impending widespread deployment of foundation models, we currently lack a clear understanding of how they work, when they fail, and what they are capable of due to their emergent properties. Moreover, any socially harmful activity that relies on generating text could be augmented based on deliberate code modifications. Examples include misinformation, spam, phishing, abuse of legal and governmental processes and fraudulent academic essay writing.
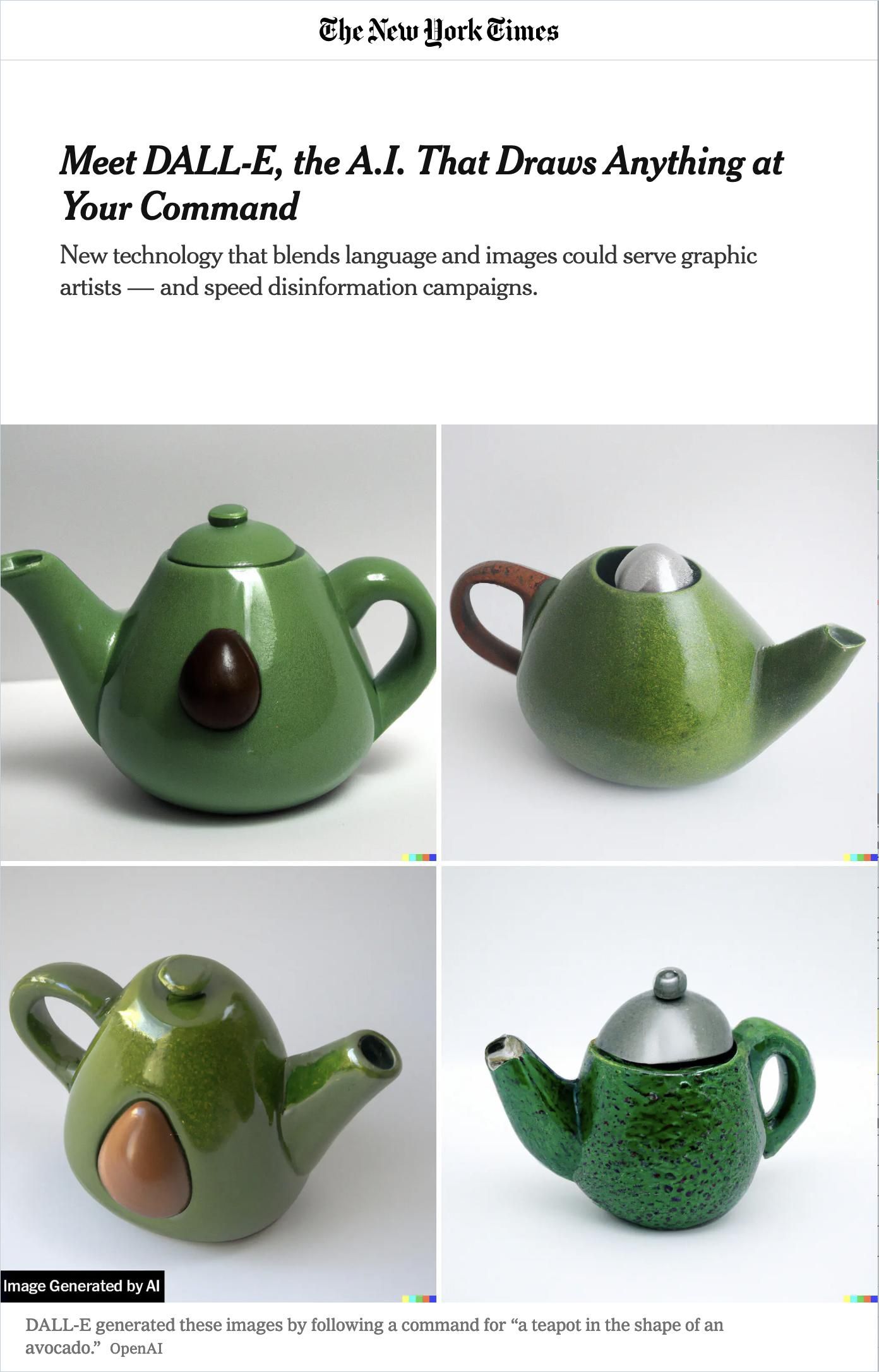
Meet DALL-E, the A.I. That Draws Anything at Your Command
The New York TimesThe misuse-potential of language models increases as the quality of text synthesis improves.
The ability of GPT-3 to generate synthetic content that people find difficult to distinguish from human-written text represents an increasingly concerning ethical issue. The relationship between the liability of users, foundation model providers, and application developers, as well as the standards of governments to assess the risk profile of foundation models, needs to be resolved before foundation models are deployed beyond the current prototyping phase.
Humans and Machines Need Each Other
The rise of foundation models is attributable to the oft-repeated mantra of ‘bigger is better’ for machine learning applications. This intuition is backed by studies that show that model performance scales with the amount of computing time, making it a consistent method in advancing the state-of-the-art.
OpenAI reports that the amount of computing capacity used in training the largest Transformer doubles every 3.4 months.
Creating high-value content engages humans who are knowledgeable in the technical domain of intelligent machines and those who are knowledgeable in the domain of psychological behavior. Foundation models link the capacity of humans from both worlds. This ‘co-creative’ effort can potentially drive humanity to the next higher level of evolution.
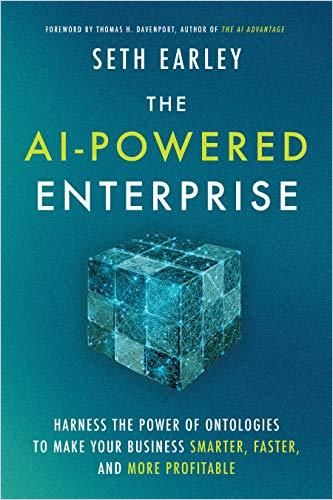
The roadblocks to getting there, however, are enormous. We are closing in on a decisive moment in human history. Only the future will tell how our socioeconomic structures will evolve vis-à-vis the continuous expansion of scientific and technical knowledge supported by the application of foundation models.