With Curiosity Towards a New AI
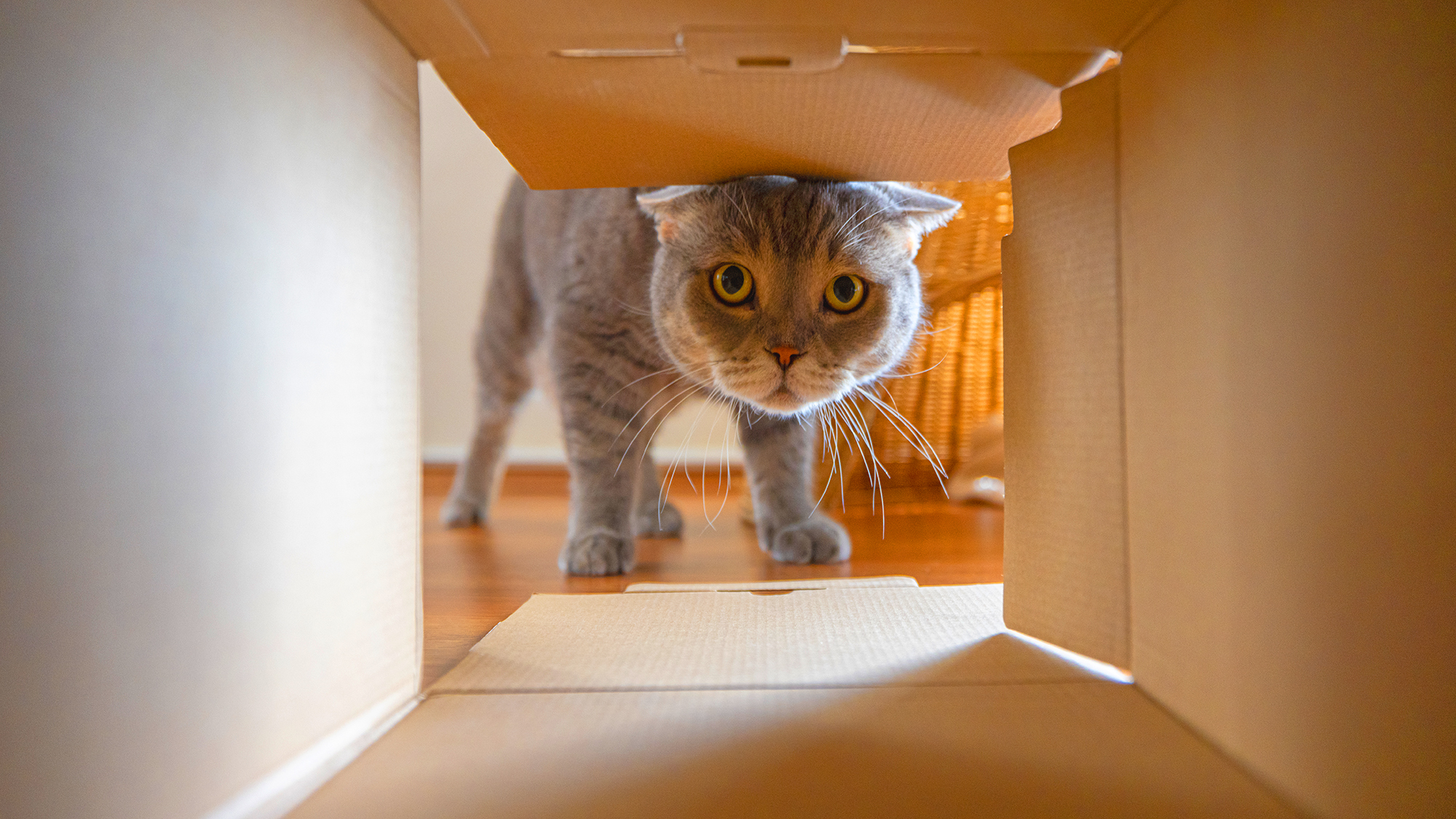
Curiosity is a significant factor in human development. It fosters the process of learning and the desire to acquire knowledge and skill. Curiosity is common to humans of all ages, from infancy through adulthood, and the motivation that drives curiosity is a passion for knowledge, information, and understanding. Curiosity strongly relates to learning with insights from ongoing research in Psychology, Neuroscience, and Artificial Intelligence (AI).
Curiosity Theories
Research of curiosity has intensified over the last couple of decades. The following lists some of the more popular theories explaining the nature of curiosity:
- Curiosity-drive theory
Curiosity-drive theory is related to the unpleasant experiences of uncertainty. The elimination of these unpleasant feelings is rewarding. The general concept dictates that curiosity is developed strictly out of the desire to make sense of unfamiliar aspects of one’s environment through interaction. - Motivation and reward
The concepts of motivation and reward strongly relate to the notion of curiosity. The idea of reward is the positive reinforcement of an action that encourages a particular behavior by using the emotional sensations of relief, pleasure and satisfaction correlating with happiness. - Memory and learning
As curiosity drives the desire to learn from new or unfamiliar experiences, one’s memory is vital in determining if the experience is indeed unknown. Memory supports the process by which the brain can store and access information. The stimulus to learn fades away if the experience encountered is not novel.
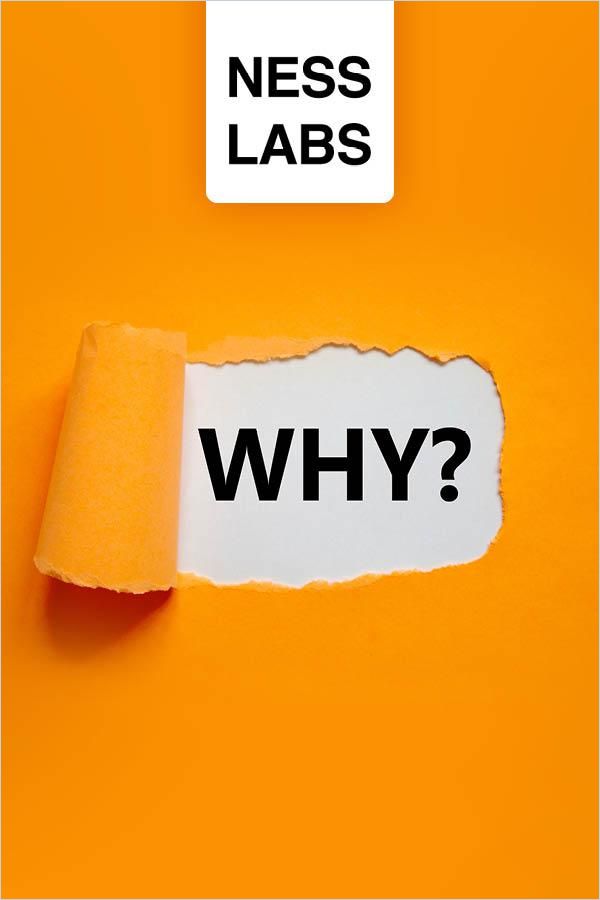
Curiosity and Learning
Most curiosity research has been focused on adults with self-reported measures, supported by neuroscientific methods such as analyzing behavior with fMRI or EEG. In contrast, Jean Piaget – considered the most influential child researcher – conducted his research based on observation. He argued that babies and children constantly try to make sense of their reality, contributing to their intellectual development. According to Piaget, children develop hypotheses, conduct experiments and then reassess their beliefs depending on their observations.
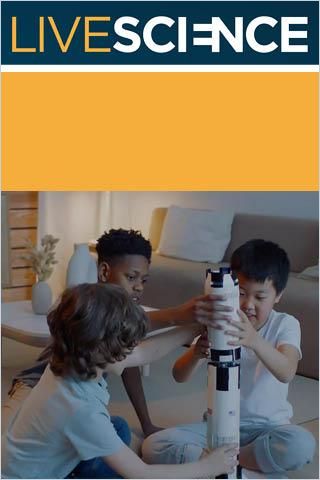
Researchers have also looked at the relationship between a child’s reaction to surprise and curiosity, suggesting that children are further motivated to learn when dealing with uncertainty. Related findings show that children structure their play to reduce uncertainty and allow them to discover causal structures in the world. This work is in line with earlier theories of Piaget, asserting that the purpose of curiosity and play was to “construct knowledge” through interactions with the world.
A Psychologist’s View
In the 1950s, Daniel Berlyne was one of the first psychologists to offer a comprehensive model of curiosity. Building on Berlyne’s insights, George Loewenstein, Professor at Carnegie Mellon University, proposed the ‘information gap’ theory. He posited that people become curious upon realizing that they lack desired knowledge.
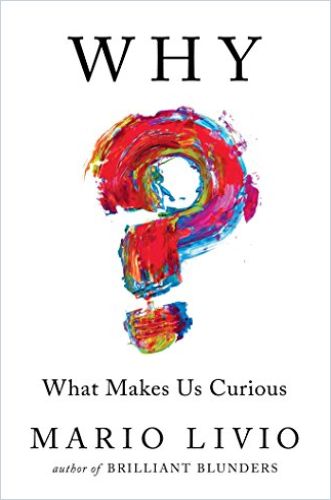
This realization creates an aversive feeling of uncertainty, which compels them to uncover the missing information. Daniel Berlyne distinguishes between perceptual and epistemic curiosity:
- Perceptual curiosity is the driving force that motivates organisms to seek out novel stimuli, which diminishes with continued exposure. According to Berlyne, this represents the primary driver of exploratory behavior in non-human animals and human infants. Epistemic curiosity is described as a drive aimed at obtaining access to information for stimulation – capable of dispelling uncertainties of the moment – and acquiring knowledge.
- Berlyne defines epistemic curiosity as an activity predominantly exercised by humans because the quality of human curiosity differs significantly from that of other species.
Another contemporary view of curiosity is that it is a unique form of information-seeking, distinguished by the fact that it is internally motivated. By this view, curiosity is strictly an innate drive, while information-seeking can be either intrinsic or extrinsic.
The Neuroscientist’s View
According to ScienceDaily, researchers at the Netherlands Institute for Neuroscience only recently discovered a brain circuit engaged in curiosity and novelty-seeking behavior. Using several innovative techniques, the scientists uncovered a path of multiple brain regions that converts curiosity into action.
Curiosity is the motivation for exploring and investigating the unknown and making discoveries. It is as essential and intrinsic for survival as hunger.
Until recently, the brain mechanisms underlying curiosity and novelty-seeking behavior were unclear. Based on the new research results, curiosity, hunger and aggression drive three different goal-directed behaviors: novelty seeking, food eating and hunting. Their experiments with mice revealed different action sequences based on the motivational level of novelty-seeking. Moreover, the researchers unveiled that the newly discovered brain circuit engaged in curiosity and novelty-seeking connects excitatory neurons with inhibitory neurons. The activation of inhibitory neurons with excitatory neurons showed a dramatic increase in the arousal level and duration of interaction with unfamiliar objects.
The exact correlation was found in studying Axons connecting the neurons. “It is the first time this path of neural activity has been described. Now we can begin to understand how curiosity sometimes wins over the urge for security, and why some individuals are more curious than others”, says one of the researchers involved in the study.
The AI Researcher’s View
Animals and humans exhibit learning abilities and understandings of the world far beyond the capabilities of current AI and machine learning (ML) systems. How is it possible for an adolescent to learn to drive a car with about 20 hours of practice and for children to learn a language? Why do most humans know how to react in situations they have never encountered? With AI, to produce reliable results, current ML systems need to be trained with enormous amounts of supervisory data labeled by human experts going through millions of reinforcement-learning trials. After engineers have hardwired hundreds of behaviors into them, we are still far from the quality of human judgment.
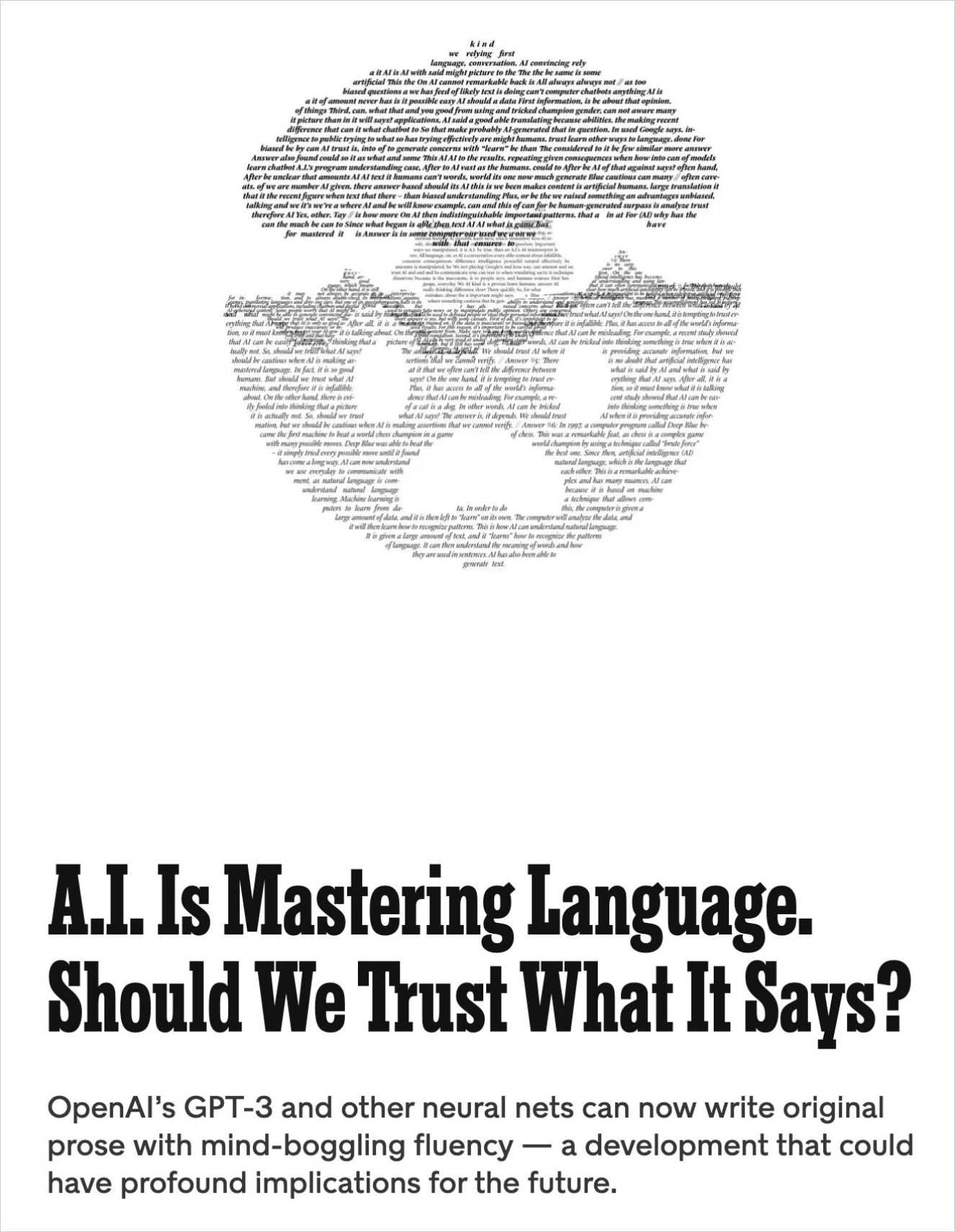
A.I. Is Mastering Language. Should We Trust What It Says?
New York Times MagazineThe answer may lie in the ability of humans and many animals to learn from so-called ‘world models,’ providing insights into how the world works. “However, some researchers think it might be enough to take what we have and just grow the size of the dataset, the model size and computer speed to simulate a bigger brain,” Turing Award Winner Yoshua Bengio said in his opening remarks at the NeurIPS 2019 conference. This simple sentence succinctly represents one of the main problems of current AI research: Artificial Neural Networks (ANNs) have proven to be very efficient at detecting patterns from large sets of data, and they can do it in a scalable way. Increasing the size of ANNs and training them on larger sets of annotated data will, in most cases, improve their accuracy. This characteristic has created a sort of ‘bigger is better’ mentality, leading some AI researchers to seek improvements and breakthroughs by creating larger and larger AI models and datasets.
While size is a factor, we still do not have any neural network that matches the human brain’s 100-billion-neuron structure.
Yoshua Bengio
Due to these circumstances, Bengio differentiates between a ‘system 1’ and a ‘system 2’ approach. “Imagine driving in a familiar neighborhood. You can usually navigate the area subconsciously, using visual cues you’ve seen hundreds of times. You don’t need to follow directions. You might even carry out a conversation with other passengers without focusing too much on your driving. But when you move to a new area, where you do not know the streets and the sights of the environment are new, you must focus more on the street signs, use maps and get help from other indicators to find your destination. The latter scenario is where your ‘system 2’ kicks into play. It helps humans generalize previously gained knowledge and experience in a conscious, explainable way,” Bengio said at the NeurIPS conference.
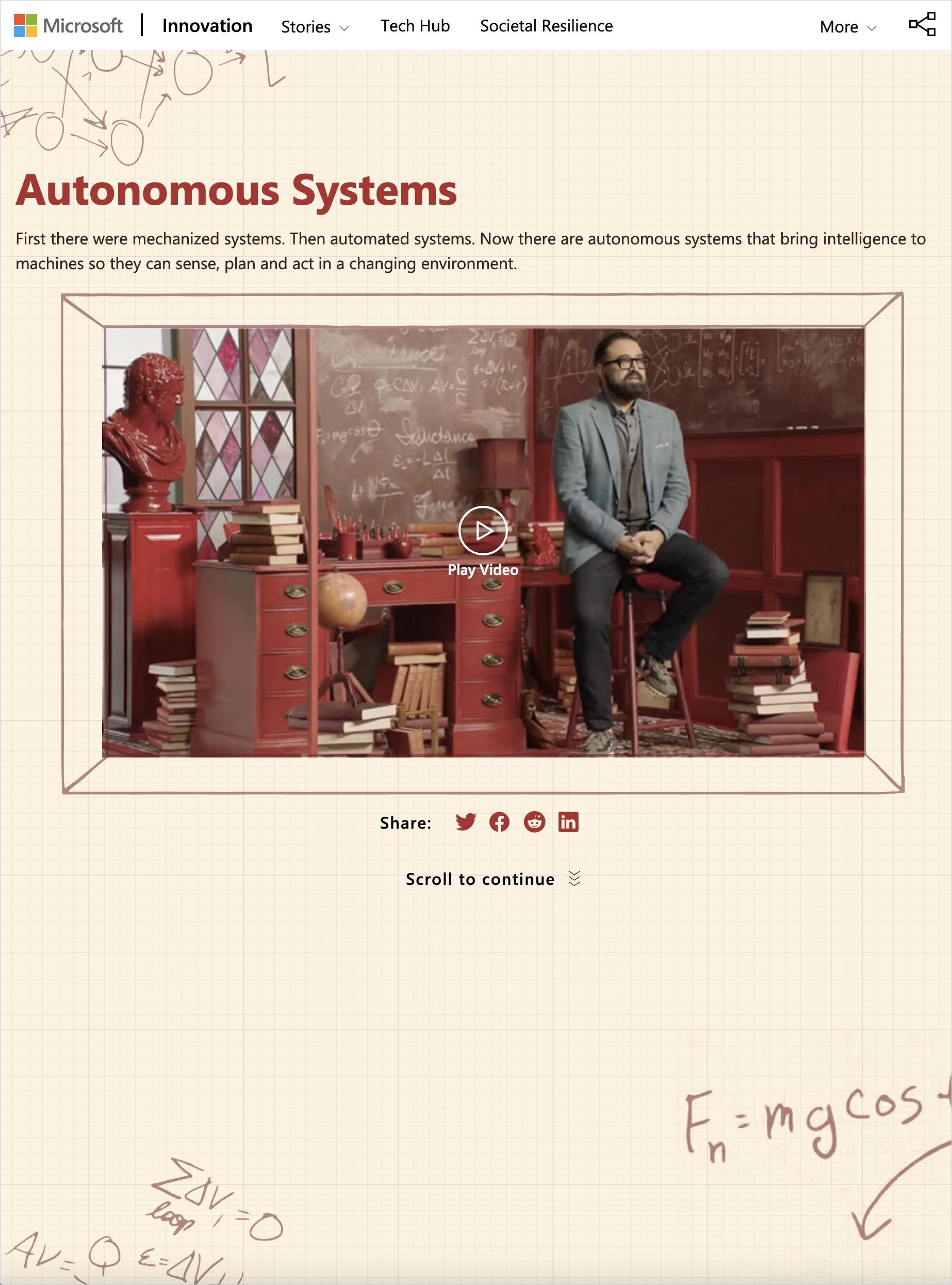
In a new paper titled A Path Towards Autonomous Machine Intelligence, NYU Professor Yann LeCun points out that humans and non-human animals can learn enormous amounts of background knowledge about how the world works through observation and a small number of interactions in a task-independent, unsupervised way. According to LeCun, it can be hypothesized that this accumulated knowledge may constitute the basis for what is often called common sense.
Common sense can be seen as a collection of models of the world that can tell what is likely, what is plausible and what is impossible. Using such world models, animals and humans can learn new skills with few trials.
They can predict the consequences of their actions and reason and imagine new solutions to problems. Importantly, they can also avoid making dangerous mistakes when facing an unknown situation. For example, a self-driving system for cars may require thousands of trials of reinforcement learning to learn that driving too fast may likely result in a bad outcome and to learn to slow down to avoid skidding. By contrast, humans can draw on their intimate knowledge of intuitive physics to predict such effects and largely avoid fatal behavior courses when learning a new skill.
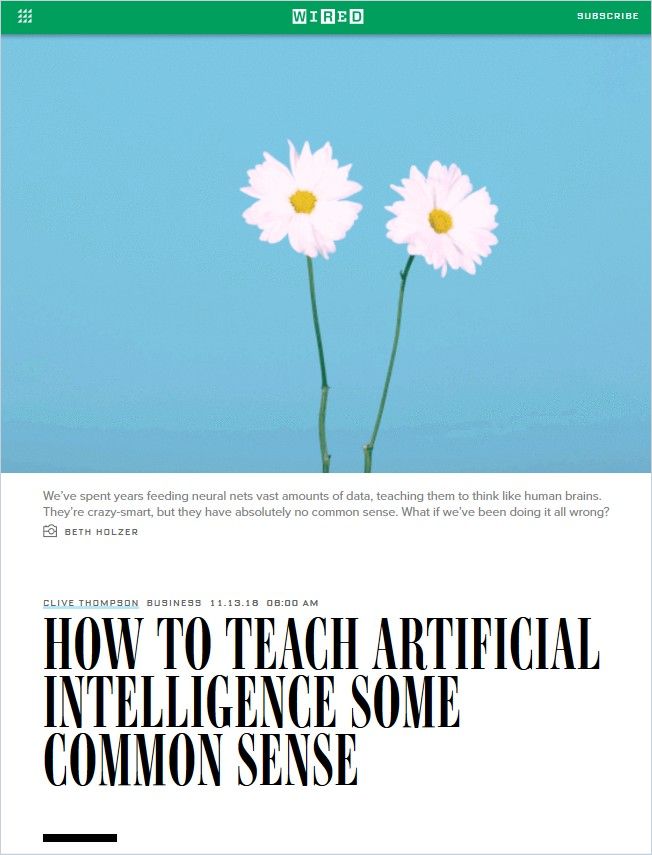
Common sense knowledge does not just allow humans and animals to predict future outcomes and fill in missing information, whether temporally or spatially. It enables them to produce interpretations of percepts consistent with common sense. When faced with an ambiguous percept, common sense allows humans to dismiss arrangements that are not consistent with their internal world model and to pay special attention when a dangerous situation presents an opportunity for creating a refined one.
Outlook
For many years now, Artificial General Intelligence (AGI) has been the holy grail of AI research, with little or no progress in overcoming causality-related problems, for example. However, based on a fundamentally new approach to self-learning systems, with curiosity and common sense as drivers, we might finally achieve a level of AI that serves humans instead of misusing humans for serving AI.
Read more about curiosity in the following Journal pieces: