“Access to Much Greater Intelligence Will Be a Step-Change in Our Civilization”
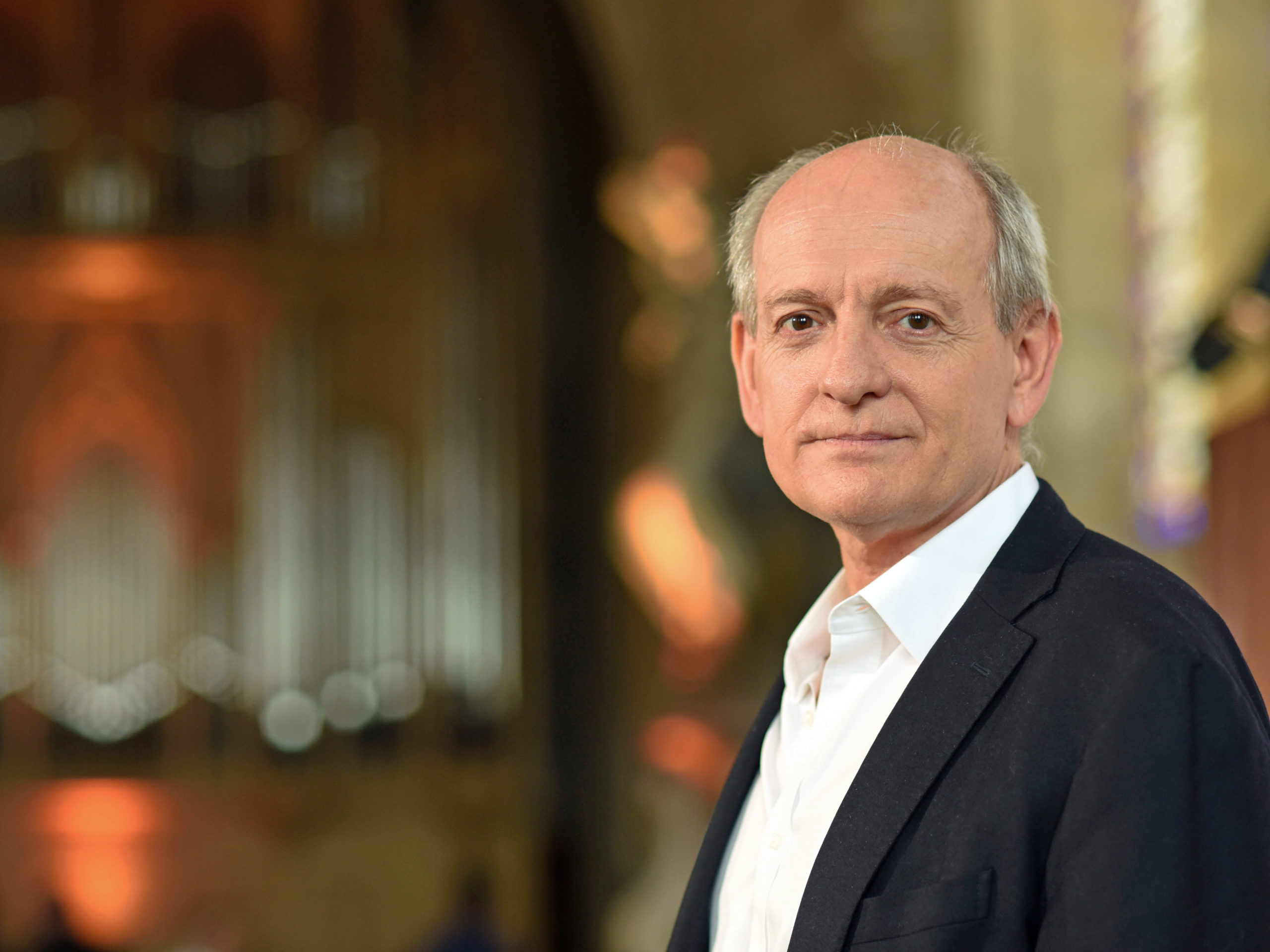
Stuart, your book Human Compatible on Artificial Intelligence addresses a wide audience. Why do you think the future of AI is something everyone should be concerned with, not only software scientists and robot engineers?
Stuart Russell: AI is already having a huge impact: Search engines, social media, eCommerce all run on various forms of AI. Everyone will be impacted by self-driving cars and intelligent personal assistants as those technologies mature. But that’s just the beginning. Every technology that exists, in fact, our entire civilization, is the product of our intelligence. If we have access to much greater intelligence, that will be a step-change in our civilization. We don’t know when exactly this will happen, but it’s safe to say that we have not yet worked out how to coexist safely and to flourish in a world with superintelligent machines.
Everybody is talking about AI these days, enormous amounts of money are raised to reach for the next breakthrough – but honestly, how likely is it that we’re dealing with a bubble here?
There have been AI bubbles that burst before, in the late 1960s and late 1980s. The field is very different now – there is much greater technical depth and the technologies we have developed really do work well for the right applications. Even if there is no further fundamental progress, it will take a decade or more for all the economically and socially beneficial applications of existing methods to be developed and exploited. The principal risk I see is possibly a pullback of the large investments in self-driving cars, which could lead to pullbacks in other areas.
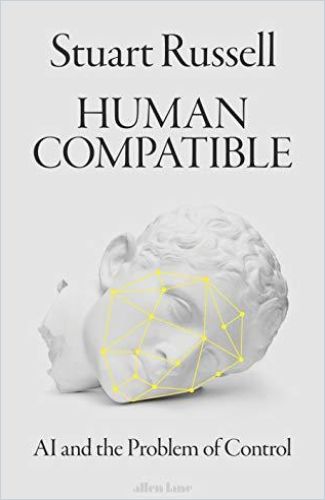
Which areas are you thinking about?
Well, there are other fundamental advances, besides deep learning, that are coming out of the research labs. For example, probabilistic programming, which provides universal representation, reasoning, and learning capabilities for probabilistic information and real-world data, is exploding right now – about 1,500 papers per year being published. That could be the next big wave, perhaps in combination with deep learning.
As of today, not even champions of digitalization use data and intelligence in a way that fits my needs: My colleagues’ suggestions still outperform any purchase-history based automatic recommendations on Amazon, for example. What are the major scientific breakthroughs necessary to achieve human-level AI?
It’s true that we are several breakthroughs away from human-level AI. But let’s not forget that these breakthroughs can happen overnight. On September 11, 1933, leading nuclear physicist Ernest Rutherford stated categorically that extracting atomic energy was impossible. On September 12, 1933, Leo Szilard invented the neutron-induced nuclear chain reaction.
But will AI ever be able to compete with humans in their ability to use “multiple levels of abstraction”?
The list of the major breakthroughs we still need concerns four systems: systems capable of real, rather than superficial, understanding of human language; systems capable of managing and directing their own computational activities – their own thinking, if you like – so that what they think about is useful for what they need to do; systems capable of cumulative learning of concepts and theories, so that they are not always learning from scratch but instead use what they know to learn effectively from new information; and systems capable of reasoning and planning over long time scales.
Which one is the most important?
This last area:
AlphaGo is amazing at planning ahead and can think 60 or more moves into the future. But just driving to work involves not tens but tens of millions of motor control decisions.
How do we manage this? By thinking at multiple levels of abstraction: we operate at dozens of levels, from tiny movements of the steering wheel as we stay in lane, to driving to work, to planning and executing a week-long project with colleagues, to bringing up our children and having a successful career. Machines are mostly unable to create these levels of abstraction and to move seamlessly among them to generate effective behavior in the real world.
Let’s pretend human-level AI was within reach. As of today: what are the foreseeable up- and downsides this societal change will bring with it?
There are two complementary ways to think about this. First, with general-purpose AI, you get “Everything as a Service.” Right now, we have “Travel as a Service.” For example, if I want to go from Lucerne to Los Angeles, I get out my phone, tap for a minute, and tomorrow I’m in Los Angeles. Two centuries ago, this would have been a multi-year, multi-billion-dollar project with a very high risk of death. Now, it’s almost instant and almost free. This idea would extend to – nearly – everything because AI systems would embody all the knowledge and skills required. Need a new school building for the village? Tap-tap-tap. Replant crops after a flood? Tap-tap-tap. Dinner party for 100 guests? Tap-tap-tap. And so on.
And the second way?
The second way to think about it is to realize that the cost of something, whether it’s a house or a pencil, consists of all the money paid to humans at all stages of production, whether it’s wages to people mining raw materials or profit to owners of factories.
If there are no humans at any stage of production, and the machines build and manage themselves, the costs are almost all eliminated.
It would take a long time to get to this stage, and people may object along the way, but that’s the logical endpoint.
Once robots take over many jobs currently performed by humans, and AI systems help people living the life they choose, will people work less or just do different jobs?
Some argue we can all live a life of leisure with a universal basic income. I don’t agree with this – it seems to be an admission of failure and an assertion that humans have no useful function. It’s true that most of what we currently call work – routine physical and mental labor – will disappear. My guess is that most of us will be engaged in providing person-to-person services of all kinds so that each of us leads a much better life with the help of others. For this to work, we will need to be much better at adding value to people’s lives.
For example?
Take childcare: Why do we pay $6 an hour plus all you can eat from the fridge for someone to look after our children – the most precious thing we have? But we pay $125 an hour for someone to fix our car. The reason is that we know how to fix cars, and we train people accordingly, but we don’t know how to look after and raise children, so we don’t pay very much for it. If people are to have valued economic roles in the future, we need to give them the tools to be effective in those roles, and that means research, training, professionalization in the human side of civilization, not just the medical, mechanical, and electronic side. It will take decades and we haven’t started.
Sounds promising. But we all also know the dystopic science fiction scenarios about AI turning against humanity. In your book, you point out that this fiction is not so unlikely if we refuse to redefine the way we think about AI and their man-introduced objectives. For example, how do we prevent intelligent machines from “turning us off” as soon as they realize that we’re their primary “competitors” in a race to power – in a scheme where “the winner takes it all”?
The issue is not so much “power” and “winning.” The problem comes from the pursuit of incorrectly or incompletely specified objectives.
The ‘standard model’ for AI systems requires that the objective be specified, but we don’t know how to do this for systems that operate in the real world.
As the King Midas example illustrates.
The King-Midas-Problem is a central part of your book. You write: “Midas, a legendary king in ancient Greek mythology, got exactly what he asked for – namely, that everything he touched should turn to gold.” – With catastrophic consequences because even his food and the people around him turned to gold as soon as he touched them. He hadn’t expected that when expressing his wishes.
The standard model is wrong because it requires that the human provides the objective to the machine, which the machine then pursues.
You give another, more contemporary example for this flaw: Let AI “pursue the noble goal of finding a cure for cancer – ideally as quickly as possible, because someone dies from cancer every 3.5 seconds. Within hours, the AI system has read the entire biomedical literature and hypothesized millions of potentially effective but previously untested chemical compounds. Within weeks, it has induced multiple tumors of different kinds in every living human being so as to carry out medical trials of these compounds, this being the fastest way to find a cure. Oops.” Well, okay, so let’s say: poorly designed human-level AI may threaten humanity. How about a better design? Is there a way to ensure a better, maybe a “good design”?
Yes. Instead of providing the objective, we want machines that pursue the true objective, which remains within us – our underlying preferences about how the future should be or not be – and not in the machine. The machine is always going to be uncertain about human preferences, which means it will defer to us, allow itself to be switched off, and so on.
Your goal: provably beneficial AI systems. What defines a PBAIS?
A system such that humans who have such a system are provably better off, in a way that the humans themselves would agree with. The reason we want provably beneficial systems is that, as AI systems become more capable, we can’t afford mistakes.
When the future of humanity is at stake, hope and good intentions – and educational initiatives and industry codes of conduct and legislation and economic incentives – are not enough. All of these are fallible, and they often fail.
Any formal proof requires assumptions to be made; initially those will have to be very strong and unreasonable – for example, the assumption that humans are rational – but the assumptions can be relaxed as the new approach is fleshed out. We need to guard against more than just machines pursuing incorrect objectives: we need to worry about machines manipulating human preferences to make them easier to satisfy, machines convincing humans to overwrite the initial program, and so on. There’s a lot of work to do.
Your suggestion is: Don’t specify the outcome or the preference humans have – let the machine find out from initial uncertainty. What problem does this new approach solve?
It solves the King Midas problem. A machine that can distinguish between a human’s true underlying preferences and a short-sighted request might respond, “Are you sure you mean everything you touch? How about you point to things and say ‘abracadabra’?” Or it might even reply, “How sure are you that more gold will make you really happy?” This way, the machine becomes more useful as it learns more about each person’s true preferences, but it will always be uncertain. It wants to avoid doing anything we don’t like, so it will allow itself to be switched off, on the assumption that the human is doing it to prevent something really bad from happening. A machine with a fixed objective, on the other hand, will never allow itself to be switched off, because then it would fail in its objective.
So, let the only source of information be human behavior – don’t you think this might be the most dangerous setup of all?
There’s no way around it – human behavior is the primary source of information, including verbal behavior, the historical record, and so on. Obviously, cockroach behavior doesn’t tell us much about human preferences, and nor does the shape of clouds or the sound of waves crashing on the shore. The only other plausible source would be neuroscience – brain scans and so on; we might need to use such devices to understand the preferences of a shut-in patient, for example[i].
But what about our greatest flaws? Hate, greed, envy …
There’s no reason to suppose that machines built along these lines will adopt all the sins of the evil humans they observe and learn from, any more than criminologists become criminals.
Take, for example, the corrupt government official who demands bribes to approve building permits because his paltry salary won’t pay for his children to go to university. A machine observing this behavior will not learn to take bribes; it will learn that the official, like many other people, has a very strong desire for his children to be educated and successful.
It will find ways to help him that don’t involve lowering the well-being of others. This is not to say that all cases of evil behavior are unproblematic for machines; for example, machines may need to treat differently those who actively prefer the suffering of others. I’m working with moral philosophers to understand this issue better.
That brings us to the public, cross-disciplinary debate on AI. In your new book, you say: “The AI debate is in danger of becoming tribal, of creating pro-AI and anti-AI camps.” What side are you on?
I’m in the pro-human camp. I’ve spent my whole adult life working to make AI systems more capable, because I think they can be incredibly useful to us; but we have to be sure that they will be useful to us, and not lead to catastrophe. That’s what I’m working on: a plan for what happens if we succeed.
[i] We have some summaries on this topic on our “Man-Machine-Interface” channel. And if you’re really interested in the future of self-teaching robots, you’ll find some interesting thoughts here.
About the Author
Stuart Russell is a Professor of Electrical Engineering and Computer Sciences at University of California at Berkeley. His book Artificial Intelligence: A Modern Approach (with Peter Norvig) is the standard text in AI. In Human Compatible (Buy it here!), he deals with the fundamental guidelines of developing super-intelligent machines that prove genuinely beneficial to humans.
Next steps
Stuart Russell elaborates on some of the points discussed here in his TED talk “Three Principles for Creating Safer AI” and this talk on “Human Compatible AI.” So, if you no longer want to be one of the business leaders who “underestimate the pace and scope of change” (economist Richard Baldwin in our interview on “The Globotics Upheaval”) of digital transformation: Take a look at our Sketchnote on the topic, and start reading our monthly column “All About AI.”