History, Hubris and Histrionics
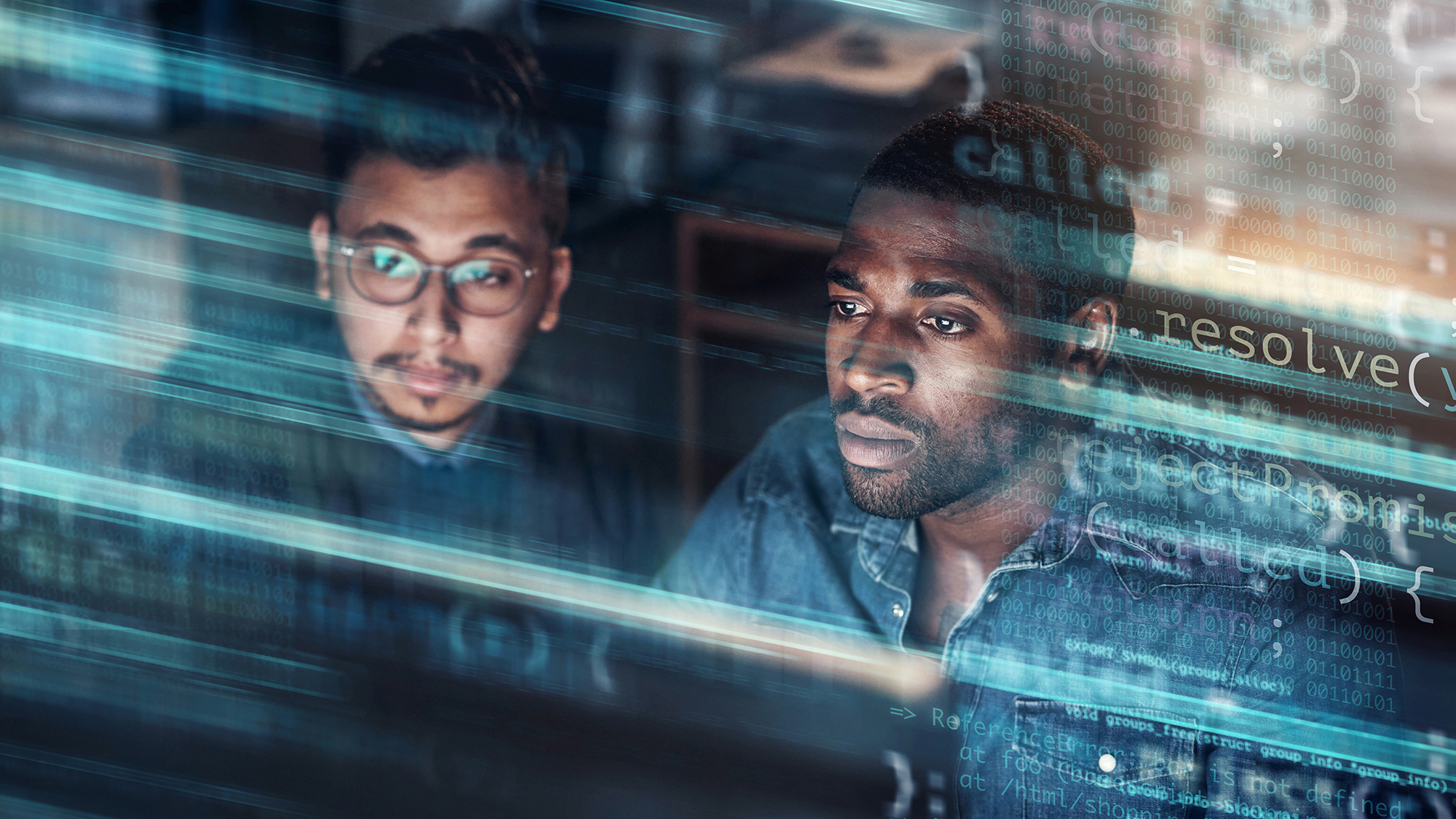
In a recent discussion with the logistics lead of a major manufacturing company, the topic of data quality dominated the conversation. The executive lamented that their pre-pandemic historic data simply no longer worked for forecasting purposes, and neither did their more recent, post-pandemic data. Basically, no matter what source of supply chain data they fed into their operating models, the resulting forecasts did not reflect their current on-the-ground reality.
This problem made accurate forecasting nearly impossible and caused turmoil throughout the organization. They had too few raw materials to fulfill existing orders. Existing orders continued to change, usually in completely unpredictable ways. The organization found that their distribution models, which used to accurately match supply and demand, were completely off base. Some buyers had understocked and bare shelves, while others had too much supply and canceled follow-up orders or asked for additional returns.
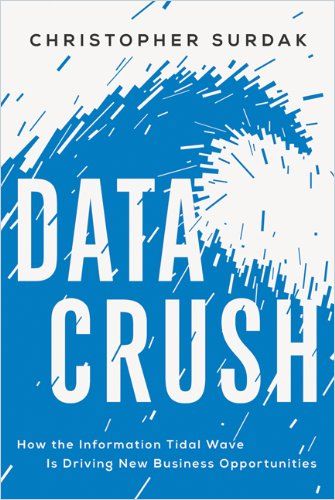
The client shared that their team had tried every variation of input data they could think of, from most recent data to data just prior to the pandemic, to data several years prior. None of these variations seemed to provide accurate results, and in most cases the broader the data set used, the worse the forecasting results became. His frustration was palpable, as these inaccuracies were leading to extremely negative financial results, to say nothing about significant, and potentially permanent, damage to customer satisfaction.
What is going on here? Why are so many organizations having such dramatic difficulty with their logistics operations? What are the causes of these problems, and more importantly, what can be done to address them?
Given that many of the changes that the pandemic brought to our world are structural and permanent (hence, “The Great Reset”), correcting this problem of operational data and modeling is critical to the ongoing viability of nearly all organizations.
While many factors feed into this issue, a small handful exacerbates organizations’ attempts to adapt. The goal of this article is to explore these factors and suggest alternative approaches to operational modeling and data use, which will make these factors more manageable.
Logistics Modeling: The Illusion of Control
Logistics modeling is critical to the operations of most organizations, and yet it is more of an art form than a science. While many logistics models used by organizations are fantastically complex, they never match the actual complexity that exists in the real world. These models are at best approximations of reality. As such, they work well when they work well, and fail spectacularly when they do not.
All logistics models have within them a wide range of estimates, assumptions and approximations, all of which prove to be accurate, most of the time. If they did not, then we would not accept these approximations, and would ruthlessly cull them from our models. Over long periods of modeling, these approximations and assumptions appear to be facts, rather than guesses. Hence, they provide modelers with the sense that they understand their supply chain far better than they actually do.
When estimates behave themselves, they tend to give modelers the illusion of control; the sense that they understand and control things in ways that they truly do not.
As a result, when these estimates no longer apply, our models begin to fail, often spectacularly. Examples of such intellectual hubris abound: Three Mile Island, Chernobyl, the Fukushima nuclear accident and Hershey Chocolate’s ERP Halloween disaster of 1999.
Ashby’s Law of Requisite Complexity
The world of software development typically leverages Ashby’s Law of Requisite Complexity. Defined by developer W. Ross Ashby in the 1950s, this law states that the solution to any problem must be at least as complex as the problem that is being solved. This self-evident solution mirrors the lesson learned by every student who ever passed algebra: to solve a problem you need at least as many equations as you have variables to solve. Obvious or not, Ashby’s Law undermines nearly every logistics model ever created, if only because the analog world is far more complex than we can ever model digitally.
Ashby’s Law reiterates that our simplified models of complex systems are mere approximations. Despite our best efforts at due diligence, it is almost impossible to properly and completely model complex natural systems, hence our models only work so long as our approximations remain valid. As soon as our unmodeled unknowns reveal themselves, like a pandemic, nuclear meltdown, supply chain disruption or lightning strike at a power station, only then are these unknowns brought to light, and only then can their impacts be correctly assessed.
Optimization Creates Fragility
Organizations have adopted lean approaches for nearly three decades; the goal of which has been to eliminate what was referred to as “waste” by those who sought to fully optimize resource and capital utilization. However, this so-called “waste” was also the slack, or extra capacity, that was held within our systems. This wiggle-room in our processes made up for the inaccuracies or variations inherent in our approximations.
The more strident our efforts were at leaning things out, the more brittle our processes and supply chains became.
This optimization process has been self-reinforcing as the more we leaned out processes without detriment, the more we believed we were doing the right thing – at little or no cost. This concept was only true as long as our underlying models and approximations remained true; as long as our hidden variables remained hidden. We embraced our approximations so long as they generated better results, and the longer they generated such results the less we believed them to be approximations. Over time, most organizations built a tremendous amount of fragility into their business processes and their value chains. We turned chains made of steel into chains made of glass and set ourselves up to find out just how fragile our chains had become.
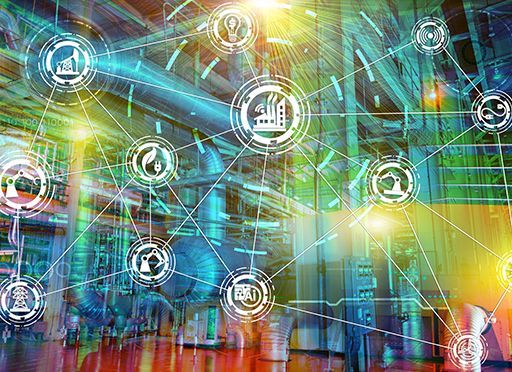
Pandemic Prognostications
In transformational times many of our most cherished and strongly held beliefs must yield to new realities that are thrust upon us. Economic “laws” that we were all taught in college are revealed to be the “mere suggestions” that they truly are. For instance, the “Law” of supply and demand states that demand for a product drops when its price increases. Perhaps so, unless there are no other alternatives for a critical demand because all of your competitors are facing the same logistics issues. Conversely, demand might fall after things return to “normal,” because during the disruption customers either found alternatives or discovered that they could readily do without your product or service.
All of these factors were likely unknown, and unknowable, through modeling prior to the pandemic. Most of these invisible factors only make sense after the fact. If they were predictable beforehand, they would have been modeled beforehand. Or, like the O-ring disaster with the Space Shuttle, the occurrence of the predictable and predicted disaster was deemed so remote as to be discounted precisely when it should not have been.
Organizations’ pre-pandemic data is necessarily flawed post-pandemic. The conditions under which that data was created are no longer valid for one of two reasons. First, one or more of the present but benign factors in our logistics chains are no longer benign; they have been activated. We can no longer treat them as zero values, and indeed their magnitude might be significant now. How many logistics models from 2018, or even 2021, anticipated gasoline at $4.50 a gallon, or a nationwide chronic shortage of diesel fuel? These were not factors to consider back then, but today they dominate our current reality.
The second reason our pre-pandemic, and even recent in-pandemic data may no longer serve us is because our pre-pandemic models are fundamentally broken.
The new conditions under which we must operate are so far gone from our recent history that data from that recent history is not only unhelpful, it can also misdirect our efforts at control. While neither of these cases is very comforting, the former is significantly more addressable than the latter.
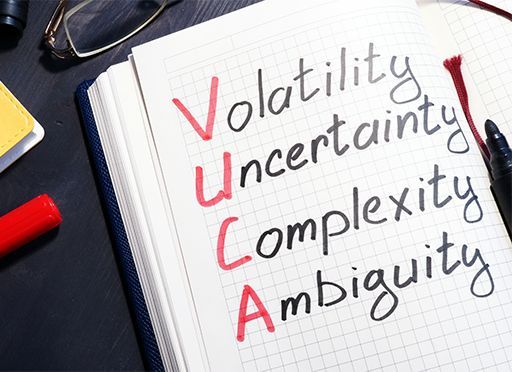
Useful Data, Wherefore Art Thou?
If any of our historic logistics data is useful in a post-pandemic, post-transformation era, which might it be? We must recognize that historic data was generated by our modeled approximations. Hence some of it might be useful to us now, but not likely the data that one might expect. In calmer times, organizations worked tirelessly to accurately forecast demand. Most of the time their forecasts were accurate, but sometimes they were not. In the instances that forecasts were significantly in error, some of the hidden variables revealed themselves and generated unexpected results.
Hence, we may find useful logistics information in the extremes of our operational normal distribution, the extremely high and extremely low values within the data that we maintain. Here, the underlying model, and its assumptions, were pushed to the limit, but they did not break. Some, or even most, of our assumptions held true. The results, while outside of our desired range of control, still fell within the reasonable limits of our understanding. If these results were not so, we would write off this data as errors, anomalies or spurious signals.
Why is this data still useful? It is still understandable within the constraints of our current models. While this data may not show optimal results for your business, it does still show results that fall within the bounds of your models’ reasonable results. If not, they would have been analyzed and rationalized away years ago.
So, if an organization wants to use historic data to model their logistics needs in the post-pandemic era, they need to utilize the data that fell into the second or third standard deviation of their data population, rather than the bulk of data that lives around the mean. In this way, you will be modeling around the extremes of predictability with your existing models and assumptions, even if you are not comfortable with the results that this data is showing you. As disquieting as these results may be, at least they remain understandable and usable.
However, if this data still cannot be massaged to match your current operational realities, it is time to accept that the world has changed sufficiently. Not only can your old data not help you, but neither can your old models and assumptions. In this case, you must start over, changing the fundamentals of your logistics models, creating entirely new data and leveraging the necessarily inaccurate forecasts that you have at your disposal.
This will lead to a great deal of error, inefficiency and cost, which will be extremely disquieting to most managers. Unfortunately, the only response managers can afford to have to these results is to accept them and learn from them. If your extreme historic data cannot be made to describe current conditions, any attempts to further use historic models and assumptions are futile, and any attempts to use the results from your old models will at best yield falsely positive results and at worse will cause even greater damage to your supply chains and your organization’s finances.
It’s Not All Doom and Gloom
While the tone of this article may seem somber, this is not the intent. Rather, it should attempt to provide realistic guidance to logistics practitioners who are struggling to regain control of their operations. The answer to your challenges is either in the right populations of your historic data or in the complete rebuilding of what you understand to be your supply chain. These are the only two options available today, and determining which path is appropriate for you as quickly as possible should be your dominant goal. If your models still work, but only in the extreme, cull your average and optimal data as quickly as possible, and get back to the task of re-optimization.
If your old models are wholly compromised, your best bet is to accept the significant cost, time and effort required to build new, relevant models as quickly as possible, so that you can reach and begin to manage your business’ new normal.
Some additional reading:
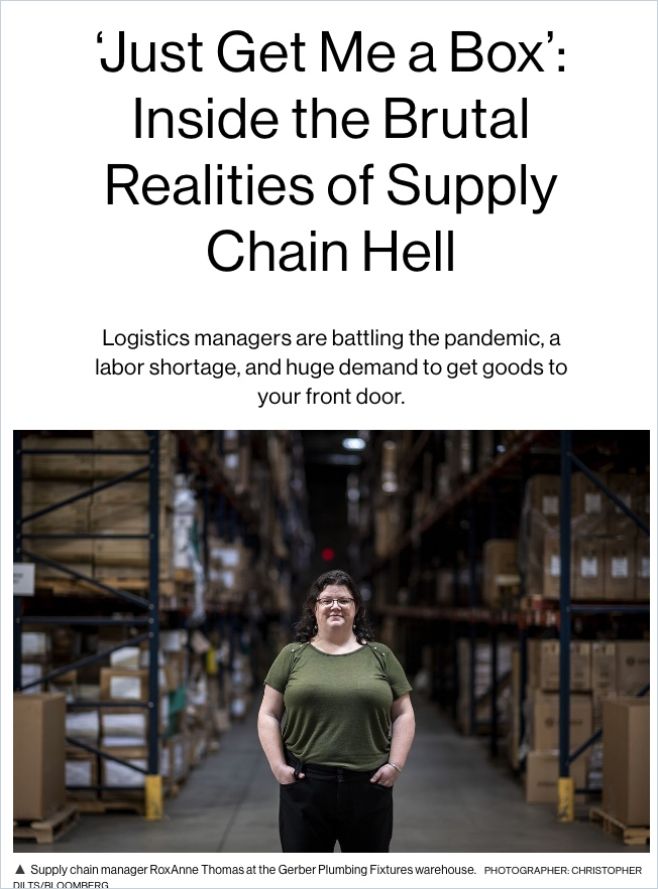
“Just Get Me a Box”: Inside the Brutal Realities of Supply Chain Hell
Bloomberg Businessweek Read Summary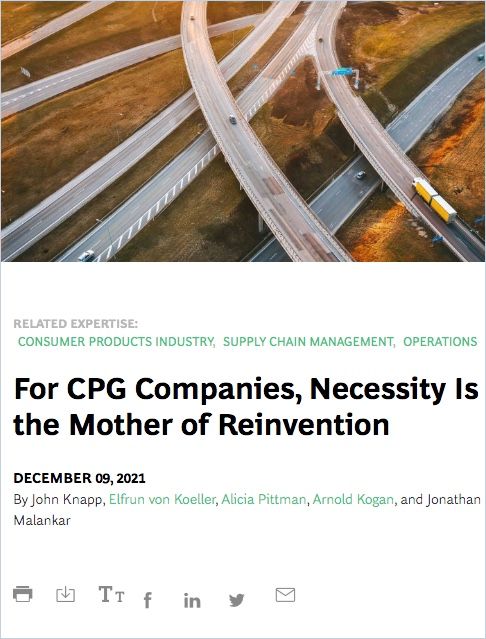