Robots Need Bias Awareness Training, Too
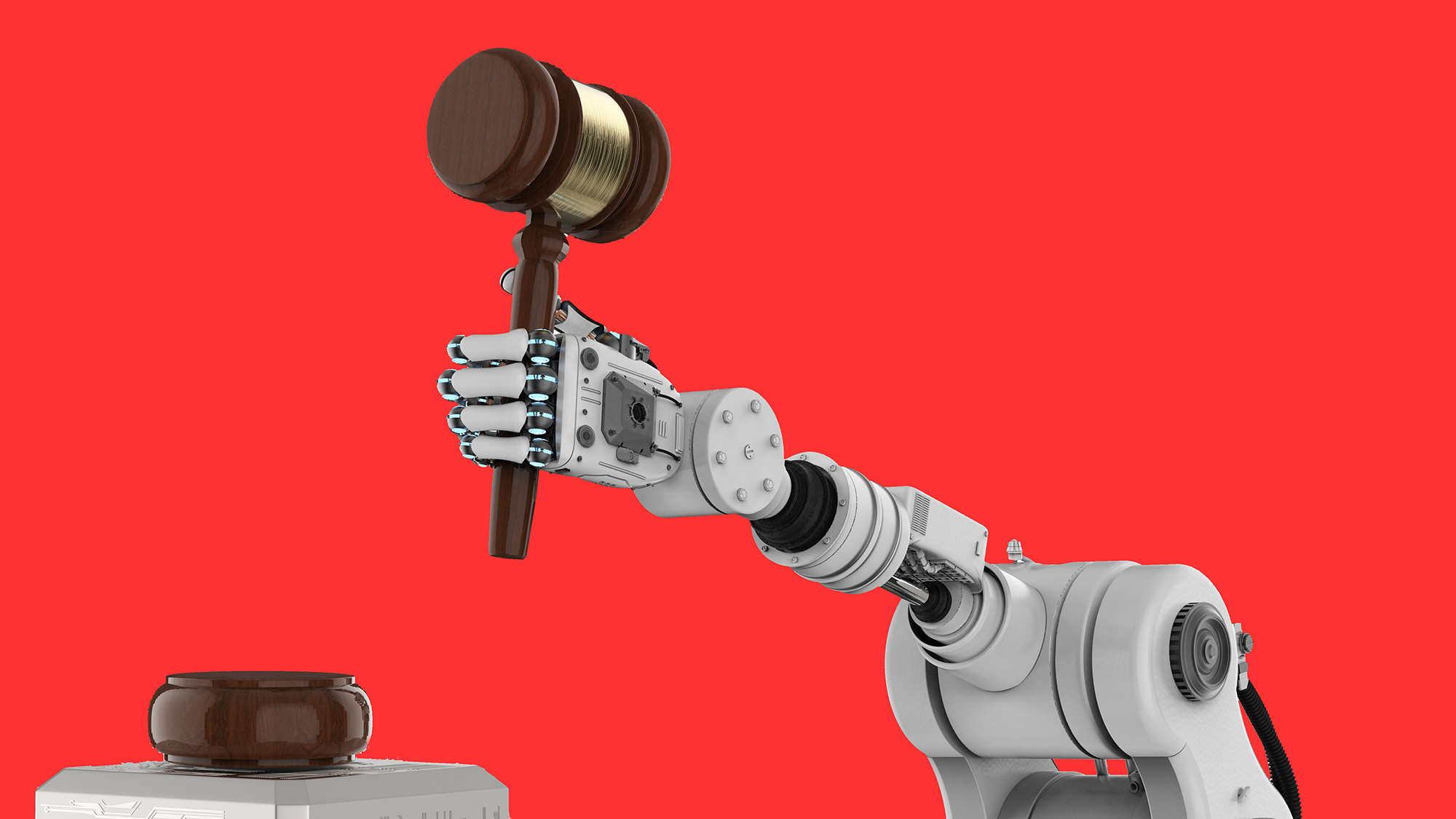
It’s piñata time! Imagine you’ve invited a bunch of kids to your daughter’s birthday party. You find yourself at a loss watching them squabble over who gets to take the first swing at the papier-mâché lama filled with candy. To prove your impartiality, you suggest drawing names from a hat. Or, if pen and paper are not your thing, you might opt for a more 21st-century version of the same – a Spin the Wheel app downloaded from your favorite app store.
A similar logic has inserted itself into decision-making of all kinds. Circumvent human bias by letting an impartial algorithm make the consequential decisions. Which job applicants should I invite for an interview? Which of the 10 bank robbers I have in custody can I safely release on bail? Which fake meat start-up should I invest in?
Computers to the rescue! They are color-blind, impartial and devoid of ulterior motives. No personal preferences or childhood traumas to skew their rational thinking powers. And when it comes to piñatas: They don’t even like candy!
Humans are narrow thinkers. Temporary states of mind and moods can sway you one way or the other. Even the best education won’t save you from making decisions on a whim. Did you know that judges rule more favorably after than before their lunch break?
Sorry to break it, but the world is more complex than a piñata party!
We have more than 100 kinds of cognitive biases. Caryn Hunt outlines some of them in her guide, Curb Your Biases.
So what can go wrong with using robots and AI to take bias out of decision-making?
As it turns out, a lot! Much of it has to do with a common concept in computer science and mathematics: Garbage in, garbage out (GIGO). Or, phrased in a more sophisticated way:
The quality of output is determined by the quality of the input.
…also known as “GIGO”
If the data sets are biased, then the output of the algorithm that learns from the data will be biased, too. Here are some examples of AI bias uncovered over the years:
- A Google research team in 2016 discovered a case of sexism in the company’s own data when an algorithm figured out what words often occurred close to each other in texts: “Man is to computer programmer as woman is to homemaker” and “Father is to doctor as mother is to nurse.”
- Google Translate defaults to use of the masculine pronoun “he” rather than “she” because “he” statistically appears twice as often as “she” in text searches.
- India and China comprise 36% of the world’s people, but only provide about 3% of ImageNet’s 14 million pictures. This explains why its vision algorithms identify a woman in a white wedding dress as “bride,” but label a traditional Indian bride photo “costume” or “performance art.”
- Google “three Black teenagers,” and then “three Caucasian teenagers.” The search result for Black teenagers often yields mugshots, while the other search delivers “wholesome” catalog photos from a stock photography library.
- The software for sentencing guidelines that courts used across the United States was more likely to label Black defendants as high risk, leading to longer sentences and higher bail for them. The software doesn’t ask the race of the defendant, but racially profiles the defendant’s answers to a 137-question survey. Because it’s software, the results gain a veneer of objectivity.
Don’t fall into the trap of thinking that software-generated results are by nature objective.
So how do you make computer algorithms less biased? Here are three suggestions:
1. Throw More AI at It
In an “AI audit,” the machine itself recognizes inequities and stereotypes. The auditor then modifies the relationships between words and pictures. For example, the AI auditor could be instructed to find a correlation between the word “woman” and the words “queen” and “homemaker,” and correct for any identified bias. The app Textio has been used to rephrase job descriptions to attract candidates from underrepresented groups. Meanwhile, the AI-powered service Mya has been deployed to find patterns of bias in a firm’s hiring history.
2. Send Your Algorithm to Bias Awareness Training
Technologists can train programs to make inclusive choices. Training AI to be unbiased may involve showing it inclusive images. Face-recognition technology isn’t as accurate for a dark-skinned woman as it is for a light-skinned man; the discrepancy results from the profusion of white faces in easily accessible training data. Remedies include new, representative data sets or special algorithms for distinct groups – that is, in effect, “digital affirmative action.”
3. Hire More Diverse Coders
People who create algorithms do not represent the world’s diversity. They belong to dominant groups and unintentionally perpetuate their own biases. Various factors compound the issue: Hiring and promotion practices tend not to favor diversity; training programs do not accommodate varied learning styles; workplace culture doesn’t emphasize flexibility; and pay for women and minorities isn’t on par with that for white men. Fixing these problems will likely take a concerted effort between various departments, including HR and IT.
AI can play an important role in helping us make more impartial decisions. But don’t fall into the trap of thinking that software-generated results are by nature objective. Sorry to break it, but the world is more complex than a piñata party!