How Much Artificiality Can Artificial Intelligence Take?
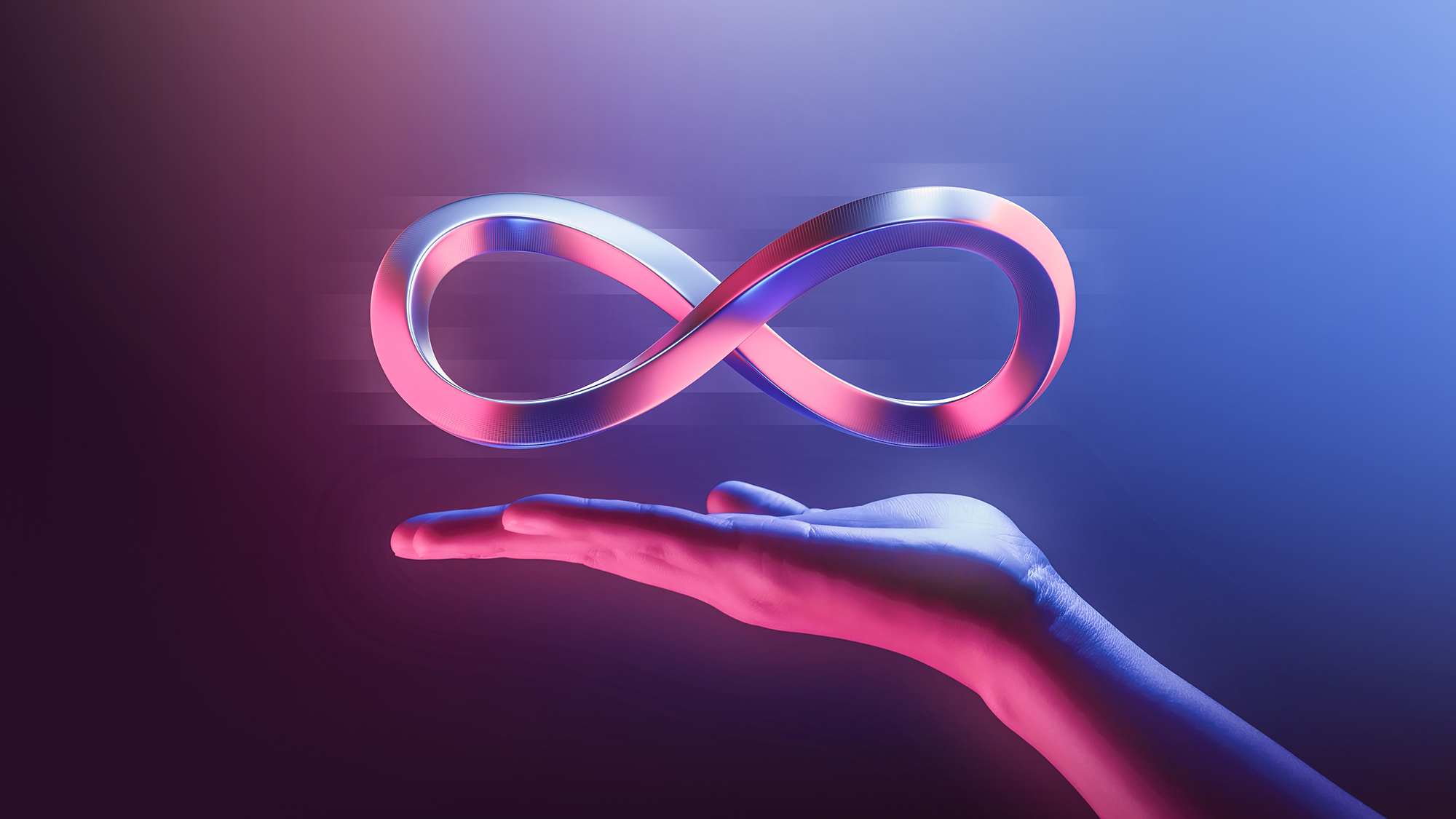
Generative AI is booming. According to a new McKinsey study, as many as half the employees of leading global companies use this type of technology in their workflows. Other companies offer new products and services with built-in generative AI. The data used to train large language models (LLMs) and other transformer models, such as GPT-4, is based on human-generated content: books, texts, videos and photographs created without the application of Artificial Intelligence (AI). Now, as more people and organizations use AI to produce and publish content, an obvious question arises:
What happens as AI-generated content proliferates through the internet and AI models begin to train on artificially generated content instead of the content generated by humans?
Generative versus Traditional AI
To understand generative AI, it is helpful to understand how its capabilities differ from the ‘traditional’ AI technologies, where companies use statistical data to predict client churn, forecast product demand and make next-best-product recommendations. The key difference is its ability to create new content. This AI-based content can be delivered as text, images that look like photos, videos and 3-D representations of artificial objects.
Today most generative AI models produce content in one format, but multimodal models that create a web page with text and graphics are also emerging. This is achieved by training neural networks with vast amounts of data and applying so-called ‘attention mechanisms’ as techniques for AI models to focus on a specific problem. With these mechanisms, a generative AI system can identify word patterns and relationships based on the context of a user’s interactive prompts, thereby creating an answer to a question without the need to provide its own human-generated data.
The Problem of Reusing Artificially Generated Content
As human-generated content is limited and costly, AI-generated content will eventually be reused to train the next iterations of generative AI models. According to a study by researchers at the University of Oxford, the University of Cambridge, Imperial College London and the University of Toronto, machine learning models trained on content generated by generative AI will suffer from irreversible defects that gradually compound as iterations continue.
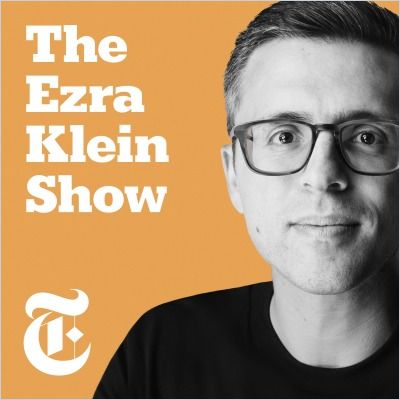
The only way to maintain future models’ quality and integrity is to ensure they are trained on human-generated content. But with LLMs such as GPT-4 enabling content creation with practically no limits, access to human-created data might soon become a luxury that few can afford.
In their paper, the researchers investigate what happens when text produced by GPT-4 is used as training data by subsequent models. They conclude that learning from data produced by previous models causes model collapse – a degenerative process whereby models forget the actual underlying data distribution, even without a shift in the distribution over time.
Machine learning models are statistical engines that try to learn data distributions. This is true for all kinds of LLMs, from image classifiers and regression models to the more complex models that generate text and images. The closer the model’s parameters approximate the underlying distribution, the more accurate they become in predicting real-world events.
However, even the most complex models are just approximations of the natural world as they tend to overestimate more probable events and underestimate fewer probable ones. Hence:
One can conclude that companies or platforms that have access to genuine human-generated content have a market advantage when training their own LLMs.
However, one must remember that human-generated content can be biased and that bias is hard to detect.
An Analysis from Neuroscience
According to a recent ETH Zurich paper, our retina tries to process visual information in the most useful way possible. However, the researchers question whether our senses provide the most complete representation of the world or do they mainly assure our survival?
For a long time, the former was the dominant view in neuroscience. In the last three decades, psychologists such as Nobel Prize winners Daniel Kahneman and Amos Tversky have shown that human perception, as fed by our senses, is often anything but complete and instead is highly selective. However, researchers have not been able to fully explain under what conditions these distortions come into play and when exactly – in a perceptual process – they begin to influence us.
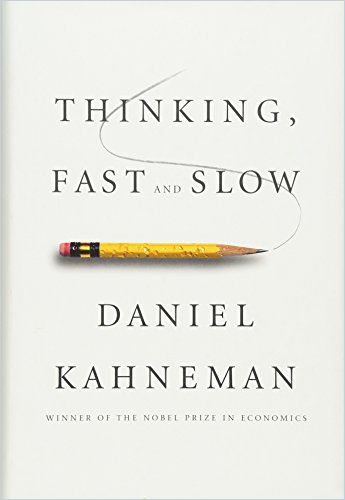
The study led by ETH Professor Rafael Polania and University of Zurich Professor Todd Hare now shows that the visual perception from our eye’s retina is adjusted by our brain when it is in our best interest to do so. Or, to put it another way:
We unconsciously see things differently regarding our survival or well-being.
The study’s results may also shed new light on discussing bias in humans and AI agents. These distortions are difficult to identify and change because they are an unconscious part of vision long before we can consciously think about what we physically see. The fact that our perceptions are programmed to increase value rather than to fully represent the world does not make things any easier. Yet – according to the researchers – the study’s results can also help us define new ways to identify and correct biases.
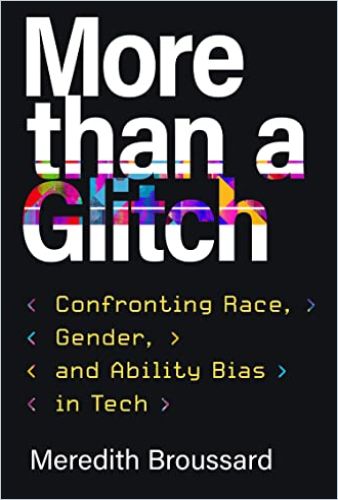
The Trend Towards Synthetic Data
According to an article just published by Quanta Magazine, researchers are turning to synthetic data to train their artificial intelligence systems because actual human-generated data can be hard to obtain. “Machine learning has long been struggling with the data problem,” said Sergey Nikolenko, the head of AI at Synthesis AI. This company generates synthetic data to help customers make better AI models.
One area where synthetic data has proved to be useful is facial recognition. Many facial recognition systems are trained with vast libraries of images of real faces, which raises issues about the privacy of the people and, most likely, the lack of consent for using the pictures. Bias poses a problem as well since various populations are over- or underrepresented. Researchers at Microsoft’s Mixed Reality & AI Lab have addressed these concerns, releasing a collection of 100,000 synthetic faces for training AI systems. These faces are generated from a set of 500 people who gave permission for their faces to be scanned.
Microsoft’s system takes elements of faces from the initial set to make new and unique combinations, then adds details like make-up and hairstyle. Another advantage of synthetic faces is that the computer can label the scanned face, which helps the neural net to learn faster. In contrast, real photos must be labeled by hand, which takes much longer and is never as consistent or accurate.
Due to the availability of more powerful GPUs, the process of generating valid synthetic data has improved as well.
Erroll Wood, a researcher currently working at Google, is using GPUs for an eye-identification project. This is a difficult task since it requires recording the movements of different-looking eyes under varied lighting conditions at extreme angles with the eyeball only barely visible. Usually, it would take thousands of photos of human eyes for a machine to identify the structure of an eye. Based on the synthetic representation, the researchers produced one million eye images. They used them to train a neural network that performed as well as the same network trained on actual photos of human eyes for a fraction of the cost and in much less time.
Outlook
Over the last decade, content firms have become increasingly adept at releasing productions with crowd-pleasing visual effects. Ultimately, that raised the bar on quality, says Rick Champagne from NVIDIA. As a result, many companies are straining to keep up with insatiable consumer demand for more and more high-quality content. At the same time, the cost of content acquisition is rapidly rising, favoring early movers with the resources to follow this trend.
However, in the long run, this might backfire as the reuse of artificially generated content might cause a model collapse with unknown consequences to the overall market.
Decentralization with smaller networks might offer one way to resolve this problem.
Learn more about recent AI and skills management developments in our related Journal articles: