Thinking, Fast and Slow (and Artificial)
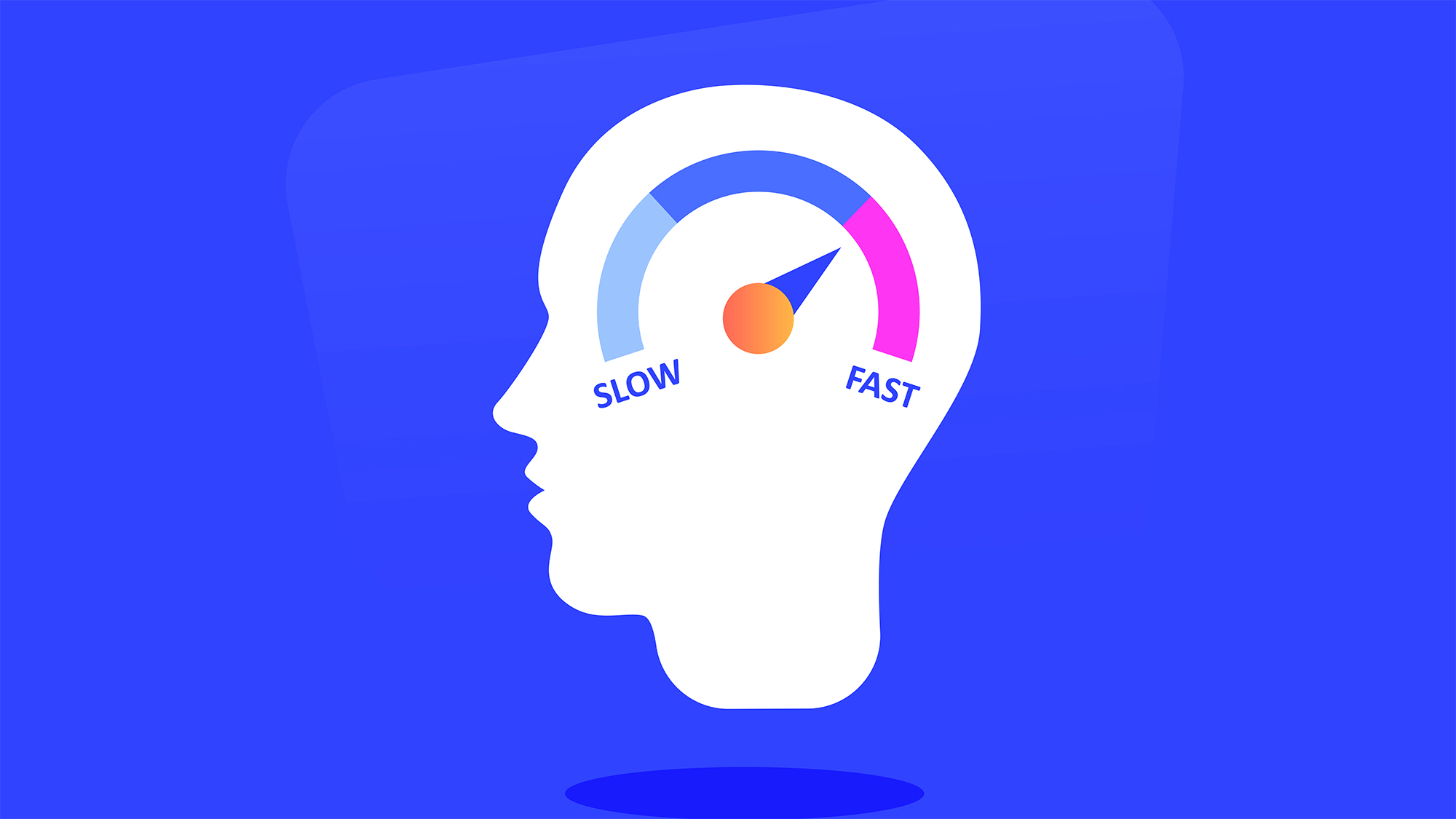
Ten years after its first publication, Daniel Kahneman’s Thinking, Fast and Slow continues to top Amazon’s bestseller list in the category of strategic management. The phenomenal success can be attributed to the fact that the book opens readers’ awareness of two concepts of how humans think in an intuitive way. The book further reveals many insights into human behavior under various conditions, following decades of scientific research. The growing interest of the AI community in neuroscience, linking neural brain activity with behavior in decision-making, offers a new positioning of Kahneman’s theory, especially as Artificial Neural Networks (ANNs) and Machine Learning (ML) continue to struggle with issues related to causality and commonsense reasoning.
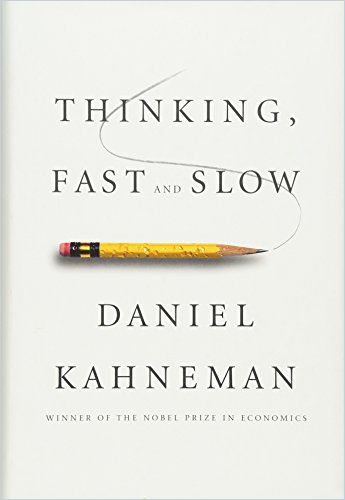
Daniel Kahneman’s Theory
According to Daniel Kahneman’s theory, human decisions are supported and guided by the cooperation of two different kinds of mental abilities: a thinking pattern defined as “System 1,” providing tools for intuitive, imprecise, fast, and often unconscious decisions (“thinking fast”) and a second thinking pattern, defined as “System 2,” handling more complex situations where logical and rational thinking is needed to reach a complex decision (“thinking slow”).
System 1 is guided mainly by intuition rather than deliberation. It gives fast answers to quite simple questions. Such answers are sometimes wrong, mainly because of unconscious bias, or because they rely on heuristics or other shortcuts such as emotional impulses. However, System 1 can build mental models of the world that, although inaccurate and imprecise, can fill knowledge gaps through causal inference and allow us to respond reasonably well to the many stimuli of our everyday life. Individually, we make hundreds of decisions every day without being consciously aware of doing so and we engage our System 1 for about 85% of our decision-making.
When the problem is too complex for System 1, System 2 is involved with access to additional cognitive and computational resources applying sophisticated logical reasoning. While System 2 seems capable of solving harder problems than System 1, System 2 still maintains a link to System 1 and its capability of causal reasoning. The causality skills of System 1 act as support of the more complex and accurate reasoning of System 2 on problems that are cognitively more difficult to solve.
With this mental capacity of switching between System 1 and System 2, humans can reason at various levels of abstraction, adapt to new environments and generalize from specific experiences. This allows us to reuse our skills to solve other problems as we learn from experience how to integrate both mental models for better decision-making.
Adding Neuroscience to the Equation
In Bursting the Big Data Bubble, James Howard, a professor at the Department of Business and Management at University of Maryland University College, explains why intuition, heuristics and emotional impulses have important roles in decision-making. Throughout evolution, the human brain has adapted to dealing with different types of decision situations. Emotions trigger the release of chemicals, stimulating the gain-potential or loss-avoidance in neural circuits. Unmanaged, these emotions take the form of impulses that raise the probability of bypassing our cognitive system. In emergencies, this may be the best course of action. For example, when speed of response is important, the amygdala region of the human brain may send a message to the cognitive system causing an automatic reaction to facilitate survival or avoidance of a threat. This emotional response in turn triggers the question of how the brain can coordinate the fast thinking of Kahneman’s System 1 with the slow thinking of System 2.
From neuroscientific research, we know that connections between neurons and associated memories can be strengthened by deliberate learning, so that when System 1 takes the lead in decision-making, the probability of good decision-making is enhanced if the cognition ability of System 2 is engaged as well. In a recent study, “How We Make Complex Decisions,” MIT neuroscientists explored how the brain uses reason to discern probable causes of failure after going through a hierarchy of decisions. They discovered that the brain performs two computations using a distributed network of areas in the frontal cortex region of the human brain. First, the brain computes confidence over the outcome of each decision to figure out the most likely cause of a failure and second, when it is not easy to discern the cause, the brain makes additional attempts to gain more confidence.
Creating a hierarchy in one’s mind and navigating that hierarchy while reasoning about outcomes is one of the exciting frontiers of cognitive neuroscience.
Mehrdad Jazayeri, MIT McGovern Institute for Brain Research, and senior author of the study
The MIT team devised a behavioral task that allowed them to study how the brain processes information at multiple timescales to make decisions. The researchers used this experimental design to probe the computational principles and neural mechanisms that support hierarchical reasoning. Theory and behavioral experiments in humans suggest that reasoning about the potential causes of errors depends to large degrees on the brain’s ability to measure the degree of confidence in each step of the process.
This two-level reasoning approach supports Kahneman’s behavioral theory that System 1 and System 2 complement each other in decision-making scenarios. Hence, a thought model based on duality – evolved as part of human evolution by differentiating good from bad, true from false or distinguishing between body and mind, for example – is key to developing future AI systems for decision-making and problem solving.
A Foundation for a New Generation of AI Systems
Taking advantage of the exponential performance increase and cost reduction in computation, one part of the AI community is focused on building ever-larger ANNs in support of new ML algorithms. Experience with GPT-3, one of the largest ANNs with billions of parameters, shows that performing reasoning tasks, such as generating natural language (NLP) does not produce consistent results. Another fraction of the AI community is making attempts to overcome these limitations, going from a “one-system” approach as stipulated by Deep-Machine-Learning (DML) applications to a “two-system” approach, adding symbolic and logic-based AI techniques (also referred to as Classical or Symbolic AI) for handling abstraction, causal analysis, introspection, and various forms of implicit and explicit knowledge in decision-making tasks.
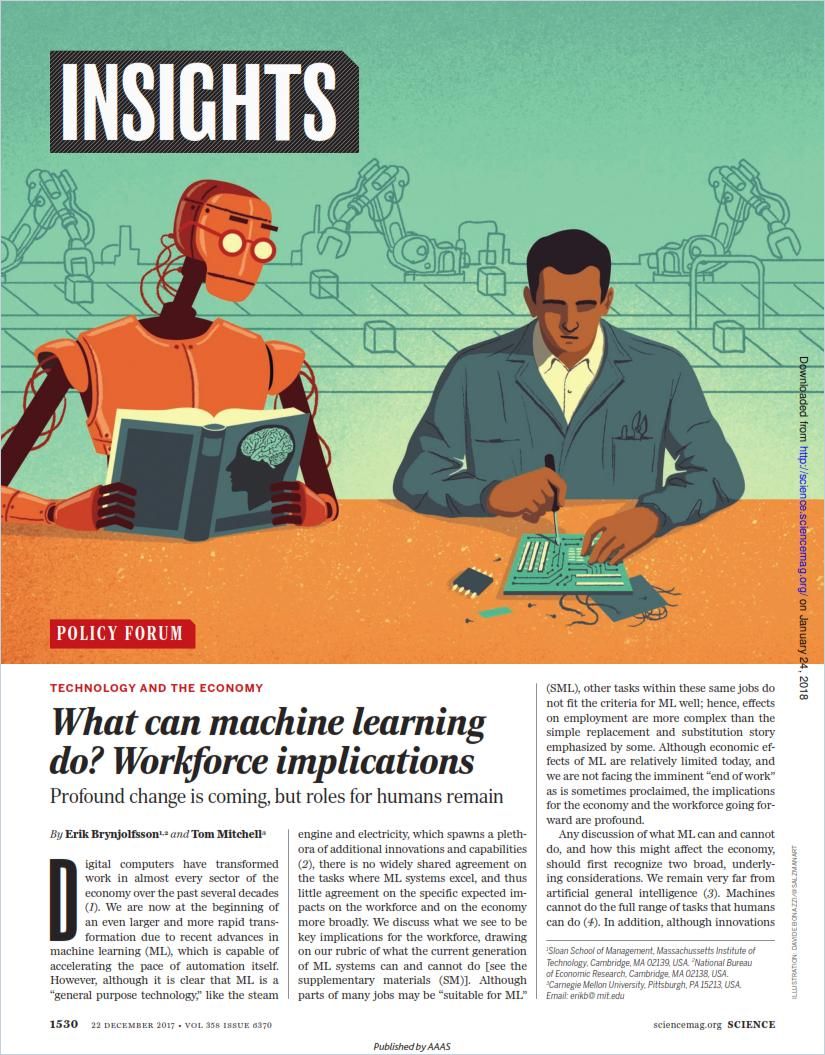
Symbolic AI relies heavily on the proposition that human thought uses symbols and that computers can be trained to think by processing symbols. A hybrid concept, combining DML with Symbolic AI, offers an intriguing possibility of drawing a parallel between the mental mind-sets represented by Kahneman’s System 1 and System 2. Analogous to System 1, DML is used to build models from sensory data. Perceptions such as seeing and reading are addressed with DML techniques, for example for image- or voice-recognition. However, this approach lacks causality, a requirement for commonsense reasoning.
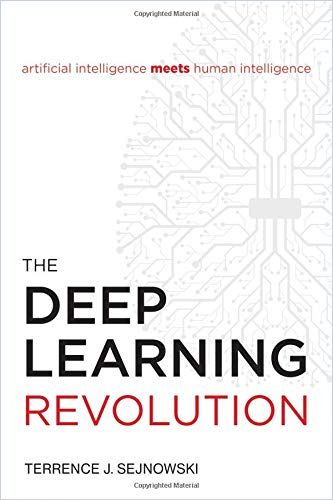
The ability of System 2 to solve complex problems stipulates the application of Symbolic AI technology, employing and optimizing explicit knowledge, symbols and high-level concepts. The quality of DML applications is measured by the degree to which they can achieve the desired result, e.g., accuracy, precision or recall in image recognition. In contrast, the quality of a Symbolic AI is measured by the correctness of its conclusions based on preprogrammed rules.
A Hybrid Approach for the Advancement of AI for Improved Decision-Making
The combined ‘System 1–System 2’ approach which switches dynamically between the two mind-sets implicates a hybrid model for AI-supported decision-making. Humans live in societies and their individual decisions are linked to their perception of reality, which includes the world, other agents and themselves. These models are not perfect, but they are used to make informed decisions and provide a testbed to evaluate the consequences of alternative decisions. However, such models are not based on exact knowledge, but rather on approximate information on the world and on beliefs of what others know and believe.
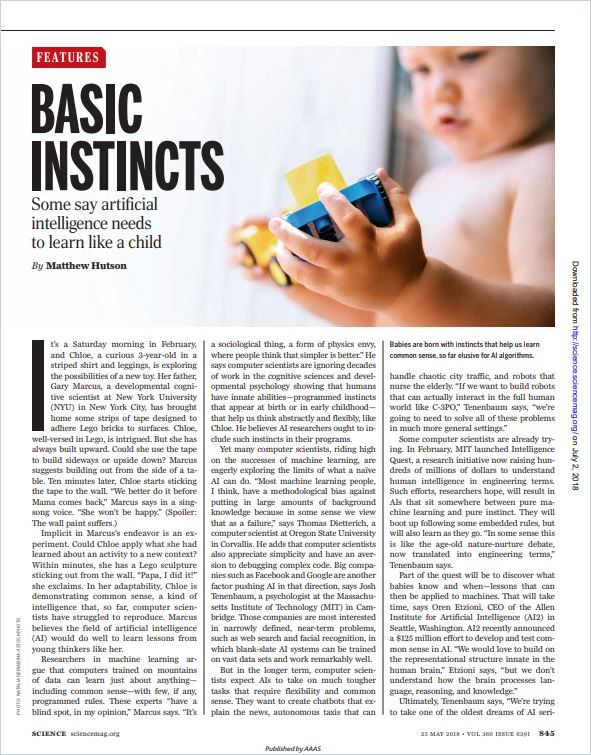
Hence, applying a hybrid concept, AI systems should include several independent components to be triggered when needed. This implies that the best structure for this kind of AI system is based on a multi-agent architecture where the individual agent focuses on specific skills and problems, acts asynchronously and contributes to building models for the problem to be solved. According to Kahneman’s theory, System 1 and System 2 support each other in specific decision-making scenarios. Monitoring and recording these activities provides the experience needed for optimizing future decision-making. This correlates with the latest neurobiological findings on human behavior and related brain activities with multiple brain regions involved in decision-making, challenging and supporting each other for reaching the optimal answer to a problem to be solved.
Conclusion
Moving from data-driven to AI-driven processes represents the challenge that businesses must meet to remain competitive and profitable. Embracing AI in our workflows affords better processing of structured data and allows for humans to contribute in ways that are complementary. Hybrid systems with massive cognitive bandwidth and data-processing power, combining a System 1 and System 2 mind-set, complemented by human’s unique resources of intuition and self-reflection, stipulate the need for a decision-making architecture that can instantaneously adapt in handling unexpected problems. Hence, the interface between humans and machines becomes the decisive factor for successful problem solving and decision-making.
Implementing such a strategy in a business context requires a mind-set of emotional – and networked –intelligence throughout the organization.
Learn more about AI and its usability:
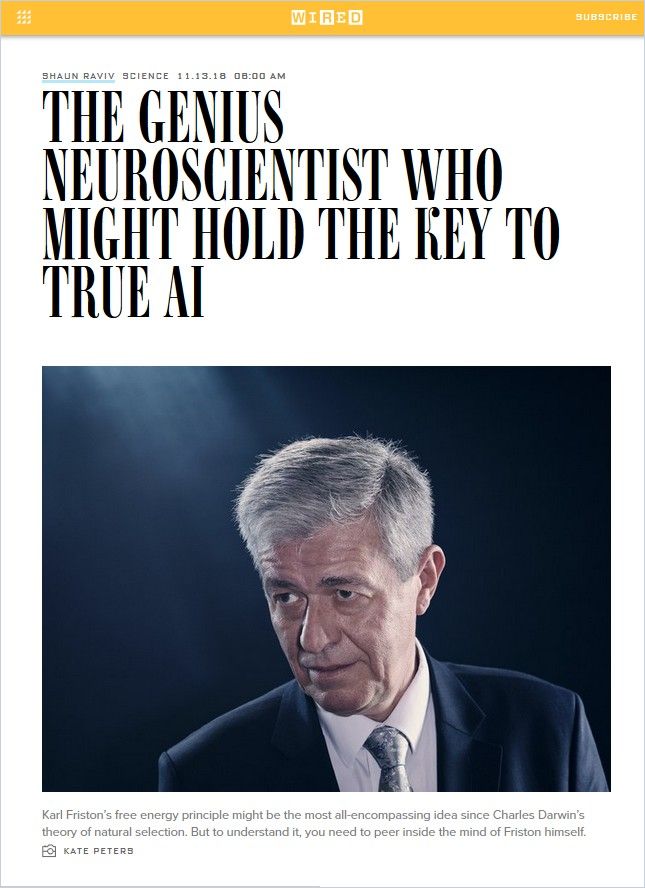
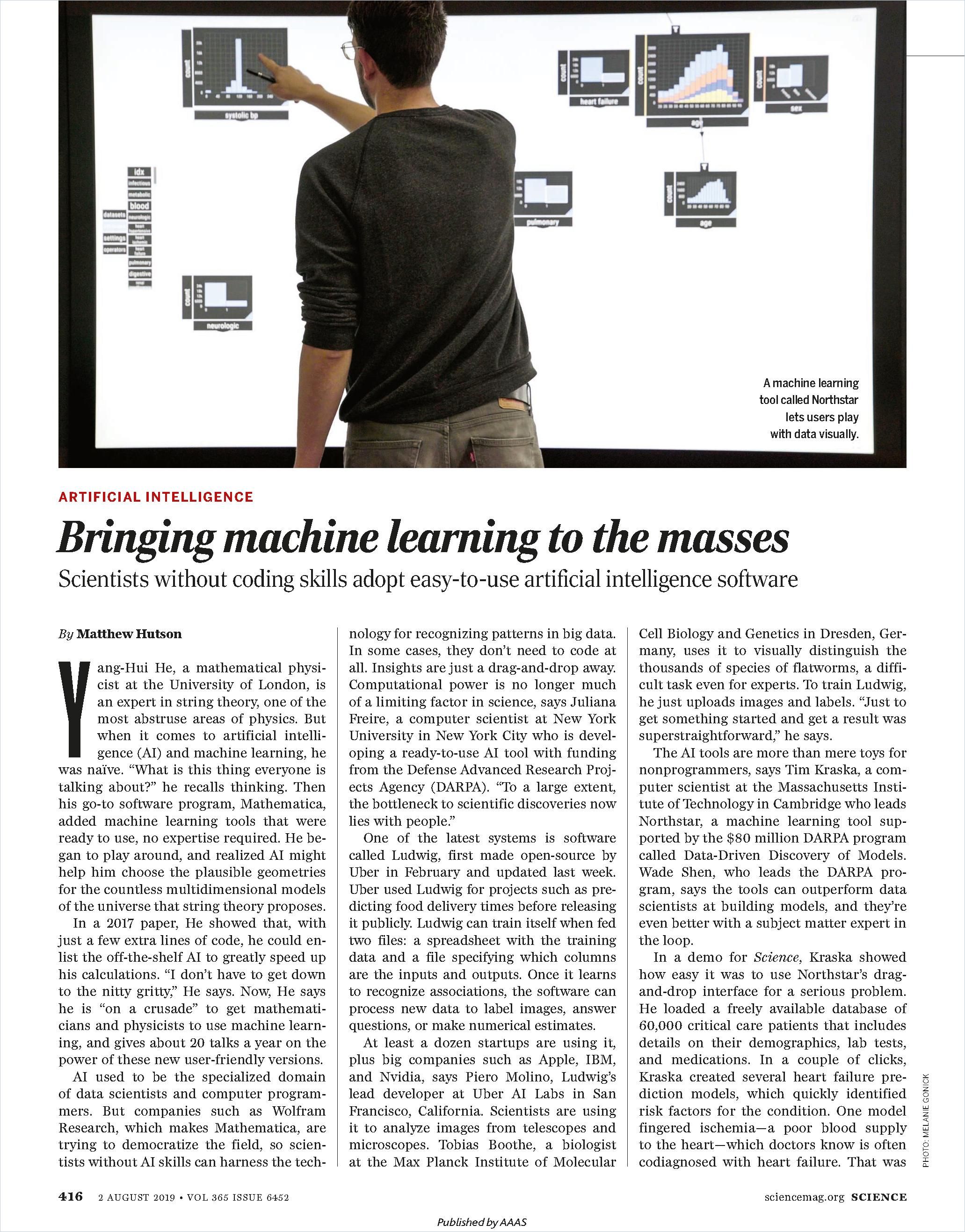
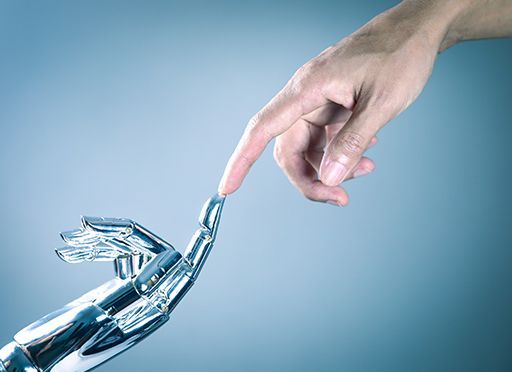