The Four Horsepersons of the AI Apocalypse: Part Two
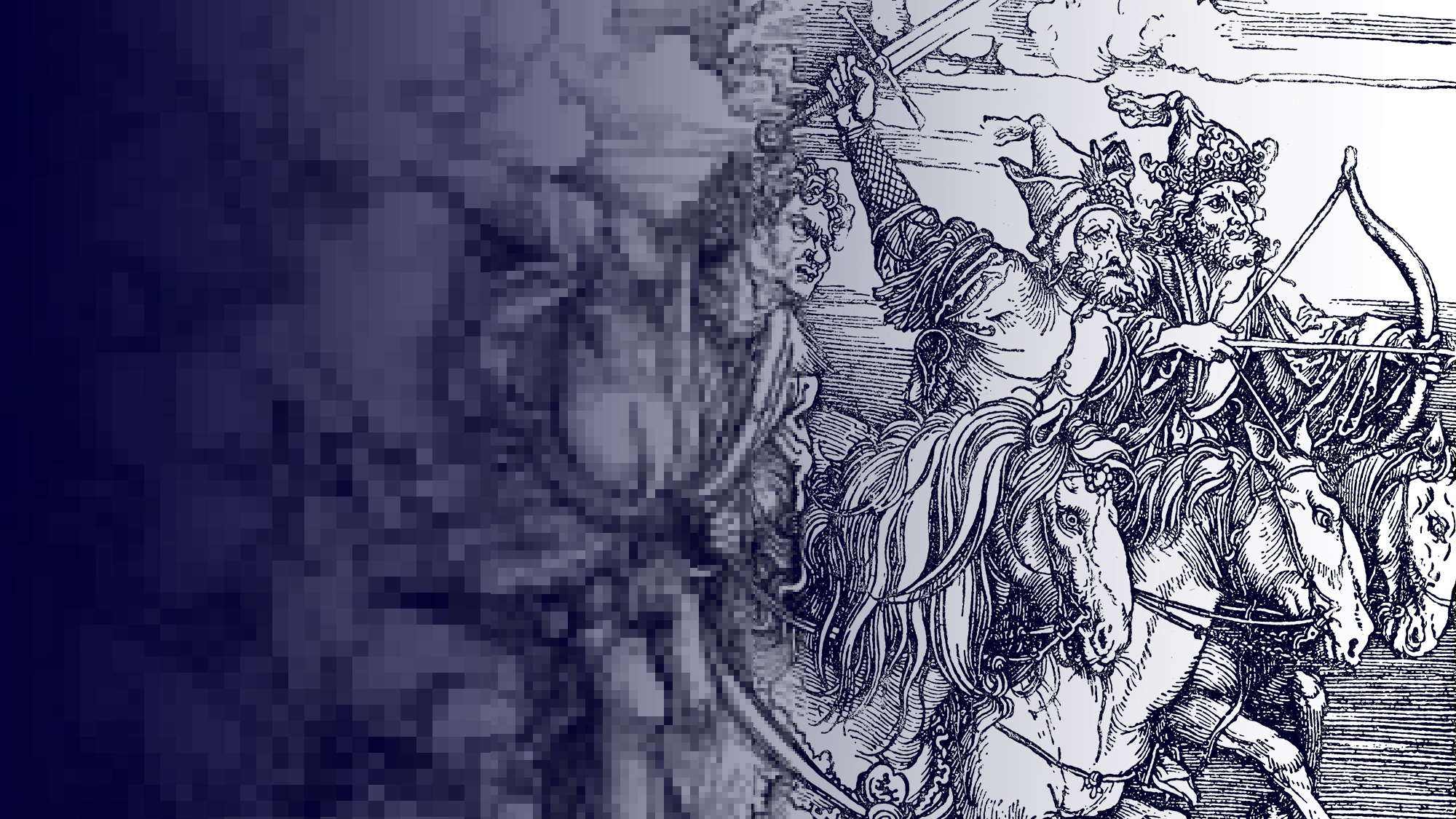
Organizations make four key errors in their attempts to do something useful with AI. If there is a 95 percent chance of getting AI wrong, one or more of these factors is likely the cause, and your project will die a slow, painful, and likely very expensive death. In Part One, I introduced you to two of these “Horsepersons of the AI Apocalypse.” Here are two more:
Horseperson #3: You Don’t Know You’re Wrong
One of the most pernicious problems in the world of data analytics is dealing with false signals. There are many types of false signals, but the two worst offenders are false-positives and false negatives. Briefly, a false-positive is where something appears to be true but is not. A false negative is where something appears to not be true, but is. These seemingly simple definitions belie the fact that false positives and false negatives can be extremely damaging to complex systems.
The greater a system’s complexity, and the greater the dependency on systemic accuracy, the greater are the costs when you are wrong.
I was fortunate to learn about false signals very early in my career. As a spacecraft systems engineer, one of my primary tasks was to make sure that a billion-dollar spacecraft, perhaps carrying a half-dozen astronauts, did not catastrophically fail. Regrettably, my colleagues and I did not always succeed in this mission. But what we did do is learn from our mistakes, or rather, our lack of knowledge.
In analyzing failures in our work, we typically found one of three possible causes. First, we had a false positive; we thought that something had occurred when in fact, it had not. Second, we had a false negative; we thought something didn’t happen when in fact, it had. Third, we screwed up and missed something.
In our experience, false positives cost money, as you thought you had to fix something that you did not need to fix. False negatives cost lives because you failed to prevent something that you should have.
Screwing up costs livelihoods, as space flight is a very expensive endeavor that does not suffer fools or the lazy. Modeling and testing were used to mitigate the first and second cases. Pride and payroll substantially mitigated the third.
The takeaway here is that it is critical to proactively test for both false positives and false negatives. The greater the costs and risks associated with being wrong, the more investment in this step is warranted. Every possible source of these false signals should be assessed, replicated in data, and tested against your solution to minimize potential losses. Then, ensure that your system is designed to fail gracefully when the unexpected inevitably arrives.
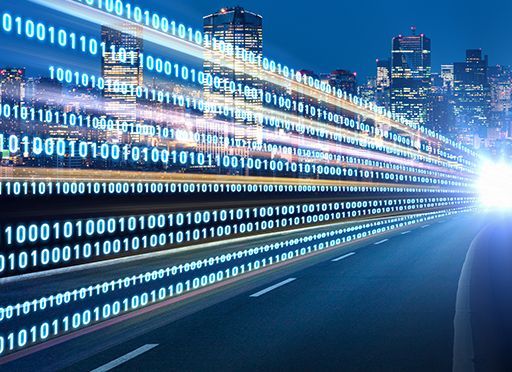
Horseperson #4: You Don’t Accept That You’re Biased
There is an old saying that “ignorance is bliss.” While there may be some truth to this phrase, it is woefully incomplete. To finish the thought, we must add, “until reality shows up!” Blissful ignorance only remains so until the bill comes due. Then, suddenly, not knowing what is going on is often replaced with a decidedly uncomfortable comeuppance.
The subject of bias in data analytics is nothing new and yet effectively dealing with bias appears to be wickedly difficult for some. Some of the types of bias to consider can be found on Wikipedia, yet this list is far from exhaustive. Further, it is important to note that some types of bias, such as the Dunning-Kruger effect, are psychological rather than numerical. Hence, an analyst must consciously determine what effect these psychological biases might have on a data set and then proactively hunt them down to weed them out.
It is not enough to examine your data to find and remove potential bias. Rather, as discussed in the prior segment, to build a system that can operate free from bias, you need to actually build in some biased data, and then teach the AI to find it and adjust to it.
This biased data should exercise both false positive and false negative filters to ensure that bias isn’t contributing to these already challenging use cases.
This is particularly true of psychology-based biases, as how these biases manifest in data can be exceedingly subtle. It is these very subtle signals that tend to get overemphasized in statistical modeling (particularly in neural networks) and lead to outsized impact post-training. Fans of the sci-fi movie “The Matrix” might recall that the character Neo resulted from the accumulation of errors in The Matrix’s calculations. Over time, these errors grew to create a super being capable of destroying the Matrix itself; this is an incredibly apropos analogy to today’s AI systems.
This sort of rounding-error-concentration is a real concern in real-life data modeling. As with Neo in the movie, when a subtle bias finally does reveal itself in your models, its accumulation won’t just be annoying. It will be dodging bullets, flying-faster-than-the-speed-of-sound annoying.
How can a data engineer respond to bias? Start off with a big, long list of the potential biases to be considered.
Then, brainstorm how each might manifest in your data as positive, negative, false positive and false negative signals. In each case, create data sets that represent these four data states and then include that manufactured data in the training data set. Finally, define what you expect the AI to do once it encounters each data state, and confirm that you get those results post-training. If this sounds like a lot of work, it is. But this is what it takes to ensure that you are in the five percent of successful AI projects rather than the ninety-five percent of AI “fashion shows.”
Success with AI is possible, if rare. Often the difference between the machine learning “haves” and “have nots” is how well the AI has been trained. Perhaps AI is not so different from humans after all.
Read about two other “Horsepersons of the AI Apocalypse” in Part One of this column series.
Or look here for additional reading:
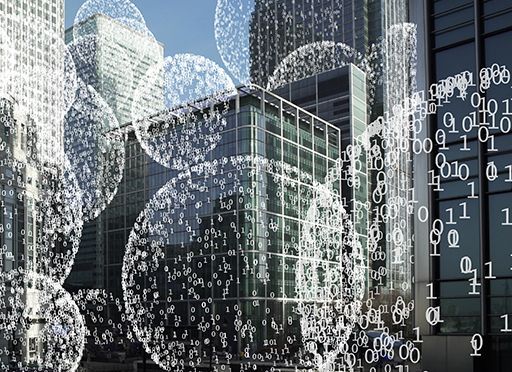