AI researchers and prolific authors Gary Marcus and Ernest Davis offer a wide-ranging, readable refutation of myths about AI capability and its likely threat or service to humankind.
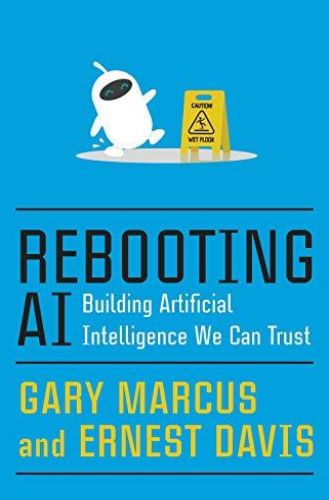
Don’t Believe the Hype
Leaders in AI research Gary Marcus – author of Guitar Zero – and Ernest Davis – author of Representations of Commonsense Knowledge believe that AI engineers focus too much on deep learning that relies mostly on statistical models and not enough on cognitive processes. Without true understanding of the world, AI cannot reliably navigate vehicles, empower robots or make ethical decisions. For AI to change lives, they insist, it must resemble the human mind.
AI
Marcus and Davis’s thesis is that current AI lacks the scope to be a threat or a great boon. Robots don’t know how to turn doorknobs, let alone enslave humans.
The narrow AI systems we have now often work…but they can’t be trusted with anything that hasn’t been precisely anticipated by their programmers.Gary Marcus and Ernest Davis
AI does what human programmers tell it to do. AI must demonstrate general intelligence that can adapt to an open-ended world, as humans do.
People believe machines are smarter than they are. But information does not equal knowledge. AI may be expert in closed systems such as playing chess, but had to play chess millions of times to gain that expertise. After seeing AI solve a problem once or twice, people assume it can solve that problem all the time. But while driverless cars operate acceptably on a quiet road in good weather, for example, they adapt poorly to new circumstances.
A robot that makes crème brûlée nine times out of ten, for instance, isn’t good enough because on the tenth time, it could set fire to your house. Because they require precise programming for every tiny task, robots make egregious errors and never understand that they do.
Deeper Understanding
Deep learning includes hierarchical pattern recognition and training. The first relied on visual systems, starting with an input layer of an image – which, through successive sorting layers, would eventually identify the image of, for example, a dog.
Deep learning doesn’t understand causal relationships, and can’t make comparisons. It requires enormous amounts of data to perform tasks humans perform instantaneously. With less data, AI makes errors. Its processes and decisions prove so complex that experts struggle to discover how they reach them, and why they sometimes don’t. AI can master “surface statistical regularities” in data sets, but struggles with abstract concepts and partial information.
Authors currently publish 7,000 medical papers every day, far more than any doctor could read in his or her lifetime. AI could provide great benefits if it could read and understand these papers, but a technology that makes connections between language and the real world remains out of reach.
The real reason computers can’t read is that they lack even a basic understanding of how the world works.Gary Marcus and Ernest Davis
To read and understand what it read, AI would need real-world experience from which to infer meaning. Understanding even a simple passage from a children’s book would require enormous amounts of knowledge about the world. Watson, the machine that excelled at Jeopardy, scanned Wikipedia for 95% of its answers.
AI doesn’t understand what it translates, and is of little use in high-stakes situations, such as interpreting a doctor’s notes. It doesn’t understand how words relate to parts of sentences – what linguists call “compositionality.” “Classical” AI is good at compositionality and creating cognitive models, but terrible at learning. Deep learning can sort immense amounts of data, but can’t understand it. Both lack crucial common sense.
Robot Butlers
Fears that superintelligent robots will rise up and conquer humankind are pure fantasy. Robots can’t turn even doorknobs or climb stairs, and contradictory signals confuse them. Their batteries need recharging. Robots perform one specialization in perfectly symbiotic environments. They can’t function in unknown terrain without human guidance.
Hardware exists that can run driverless cars and navigate the terrain on Mars, but lacks robust software. This software must do five things to demonstrate basic intelligence: It must know where it is, what is happening in its environment, what it must do that moment, how to implement a plan, and it must have a mechanism for achieving long-term goals. To accomplish any of this in real time, the machine must constantly cycle through the “OODA loop”: Observe, orient, decide and act.
Making a robot adapt to changes in its environment and react appropriately remains problematic. The robot requires “situational awareness” – anticipating what might happen next. While deep learning might identify many things in its environment, it cannot comprehend relations between objects, such as a mouse in a mousetrap.
Marcus and Davis offer these points to demonstrate the difficulties of creating AI intelligence that matches human intelligence: No “master algorithm” that reduces intelligence to one principle exists. Extracting words from their context in sentences destroys nuance. Intelligence requires integrating top-down – prior knowledge of the world – and bottom-up – knowledge people gain from experience – knowledge. Causal inferences fuel comprehending the world. The mind is not a “blank slate.” Nature and nurture don’t compete for dominance, but work in tandem.
Ethical Framework
Trust matters when building AI systems that will control how people navigate the world or make decisions about health and safety. For AI to be safe and reliable, it must undergo frequent debugging, testing and verification protocols with rigorously maintained backups.
Can you construct an AI with enough of a theory of the world to turn all the matter of the universe into paper clips, and yet remain utterly clueless about human values?Gary Marcus and Ernest Davis
Simple ethics to “cause no harm” prove problematic in a complex world – as, for example, choosing to steal medication to save a woman’s life. Programming a machine to resolve moral dilemmas is impossible today and will be difficult tomorrow.
A Relief
Marcus and Davis provide a genuine service: They fuel skepticism with incontrovertible facts. So the next time a media outlet or tech start-up touts a new, supposedly world-altering AI, you can ignore them and nod knowingly. Marcus and Davis, experienced authors, prove an evocative and highly readable team. They couch scientific and technological knowledge – and scientific and technological confusion – in comprehensible layperson’s language. Their goal is to enable laypeople to recognize AI myths or hype when it appears – and it appears all the time. Investors in particular will benefit from the authors’ informed pragmatism.