New York Times technology reporter Cade Metz presents the evolution of AI through the brilliant personalities who developed it.
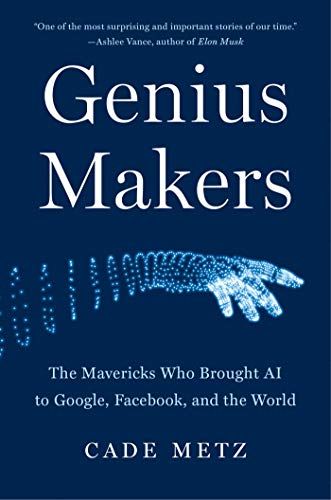
AI Evolution
Cade Metz, technology reporter for The New York Times, offers a historical overview of artificial intelligence, full of intriguing characters, technological breakthroughs and occasional dead ends. Metz discusses deep learning, China’s determination to be the supreme power in AI and concerns regarding intelligent machines.
Neural Networks
Researchers assumed that by emulating brain function, computers could learn to identify objects and understand spoken language.
In 1958, professor Frank Rosenblatt of Cornell University unveiled a neural network, the Perceptron. It performed simple tasks, such as determining whether a card bore a symbol on its left or right side.
The Navy revealed the embryo of an electronic computer today that it expects will be able to walk, talk, see, write, reproduce itself and be conscious of its existence.The New York Times, July 8, 1958
In 1969, Marvin Minsky of the Massachusetts Institute of Technology and colleague Seymour Papert published the book Perceptrons; it discredited neural networks.
Symbolic AI
Minsky endorsed symbolic AI: Voluminous, detailed rules told computers how to respond to various situations.
Since the [AI] field was created, its leading figures had casually promised lifelike technology that was nowhere close to actually working.Cade Metz
AI researcher Geoff Hinton connected with researchers in a Southern California PDP (parallel distributed processing) group, which included neuroscientist Francis Crick, the co-discoverer of DNA’s structure. The group envisioned a more sophisticated Perceptron that could identify complex objects, such as a photograph of a dog.
“Backpropagation”
In 1982, at Carnegie Mellon, Hinton co-wrote a paper on backpropagation, a concept that broadened neural networks’ abilities. The Carnegie Mellon AI lab put backpropagation to practical use in 1987, with an attempt at a self-driving car.
In 1989, Yann LeCun of Bell Labs created LeNet, an image-recognition system that could read handwritten numbers. With Bell colleagues, LeCun built ANNA, a neural network–specific microchip that processed neural network algorithms at unprecedented speeds. Johns Hopkins neuroscientist Terry Sejnowski built NETtalk, a program that could recognize printed words and read them aloud.
Deep Learning
At the University of Toronto, Hinton worked on “deep belief networks” through which he could feed unprecedented amounts of data into a neural network. He renamed the concept “deep learning.”
By 2004, a neural network was seen as the third best way to tackle any task – an old technology whose best days were behind it.Cade Metz
Li Deng, while working on a speech-recognition system at Microsoft, learned of Hinton’s approach in 2008. Hinton told Deng that with deep learning, neural networks could work with speech. Deng invited Hinton to his research lab at Microsoft. The pair developed a working system that Deng recognized would grow more able with more data and greater processing power.
Deng, with Hinton’s students George Dahl and Abdelrahman Mohamed, switched from standard CPU chips to a more powerful graphics processing unit (GPU) card. Their prototype exceeded the performance of any speech projects Microsoft had in development.
Google hired Hinton student, Navdeep Jaitly, who trained a GPU-powered machine to outperform Google’s Android smartphone speech recognition.
Google Brain
Google launched Project Marvin, which researched deep learning for image recognition, in 2010, under the direction of Stanford computer science professor Andrew Ng and his Stanford colleague Sebastian Thrun. They created enormous neural networks by combining hundreds or thousands of computers. Using more than 16,000 computer chips, they taught their network to recognize a cat, a breakthrough in neural network capabilities. The project spawned Google’s dedicated AI lab, Google Brain.
Engineers were beginning to build machines that could learn tasks through their own experiences, and these experiences spanned such enormous amounts of digital information, no human could ever wrap their head around it all.Cade Metz
By fall of 2012, Hinton and students Ilya Sutskever and Alex Krizhevsky built a neural network – Alexnet – with an accuracy that far exceeded the current best systems. Hinton, Sutskever and Krizhevsky formed DNNresearch.
Big Tech
In 2013, Facebook founded its deep learning lab – Facebook Artificial Intelligence Research (FAIR). Mark Zuckerberg hoped Facebook’s AI technology could respond to spoken commands, identify faces and translate language.
The Chinese tech company Baidu hired Andrew Ng, founder of the Google Brain lab.
Microsoft lagged behind as Facebook poached Microsoft researchers. Microsoft executive vice president Qi Lu pressed the company to acquire DNNresearch, But Google snapped it up.
China
In 2015, DeepMind’s AlphaGo system beat champions at Go – a more complex game than chess. In 2017, AlphaGo defeated Ke Jie, the world’s top player.
During the Go tournament, Google CEO Eric Schmidt implored the Chinese to adopt the new Google software system TensorFlow, which the company hoped could become a standard for AI platforms. Schmidt was unaware of the progress China’s big tech companies, including Tencent and Baidu, had made in deep learning. Two months after the match, Chinese officials announced a program to make China the dominant player in AI by 2030.
Dangers
In 2017, Google worked on the Pentagon’s Project Maven to boost its use of machine learning. More than 3,100 Google employees signed a petition demanding Google cancel the project and in 2018, Google did not renew its contract.
The risk of something seriously dangerous happening is in the five-year time frame. Ten years at most.Elon Musk, 2014
How a machine learns depends on the data engineers give it. An intern at Clarifai documented biases in a library of stock photos the company used to train its object- and face-recognition system. More than 80% of the images were of white people, and more than 70% were of men. This bias permeates academic and industrial AI research.
Clarifai, Google and IBM market facial recognition systems to government agencies. Google and Facebook installed the software in their apps and phones, and Amazon offered Amazon Rekognition to police departments.
Elon Musk feels humanity is losing control of intelligent machines.
Names
Cade Metz wraps the evolution of AI around the scientists, researchers and developers who created, developed and enhanced it. This approach produces a blizzard of names and more “begats” than the Old Testament. If you prefer your technological history presented via personality – the “great men” rather than the “great man” approach – this is the book for you. Otherwise, laypeople might struggle to keep track of everyone. Those in the field, however, will find Metz’s overview enthralling and illuminating.