“The Technologies Are Not As Robust As Anybody Imagines”
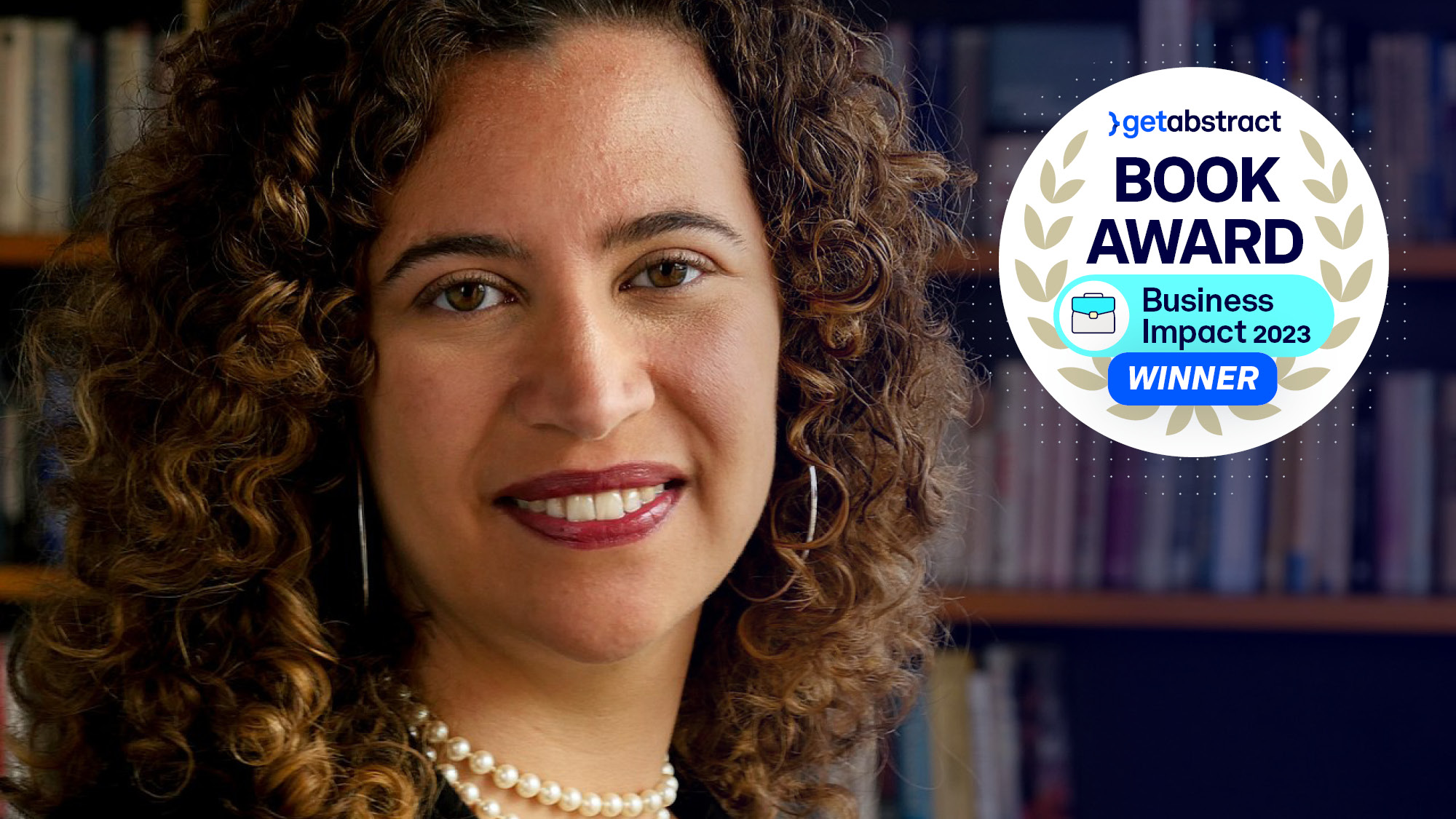
In More Than a Glitch, data journalist Meredith Broussard explores the vulnerabilities and biases that come with insufficiently vetted algorithmic technologies, as well as the change of culture in organizations that come to trust fallible systems over human judgement. To intelligently integrate these systems, operators must be mindful and disciplined about evaluating them for fairness and accuracy.
What is technochauvinism? How does it work to obscure biases in the world? How does it add to them?
Meredith Broussard: Technochauvinism is a kind of bias that says that technological solutions are superior to other ones. Instead, we should think about using the right tool for the task. Sometimes, the right tool is absolutely a computer. Sometimes, it’s something simple like a book in the hands of a child sitting on a parent’s lap. One is not inherently better than the other. When people are too deeply invested in technochauvinism, they tend to make decisions that privilege using technology over using common sense. I read a story recently about a school district that needed more bus drivers. They were having trouble finding people. So, instead of raising the wages for the bus drivers so they could keep and hire more people, they invested in an algorithm to redo the bus routes. Kids ended up being on the buses for hours. Really, what they needed were more bus drivers; they didn’t need more technology.
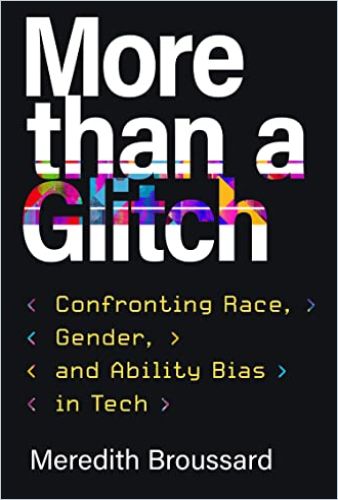
That example is a great illustration of a point you make throughout your book that mathematics and social realities don’t always align.
In the book, I tell the story of when I was a kid and there was just one cookie left in the cookie jar. My little brother and I would fight over who got that last cookie. We’d divide the cookie, but then there would be another fight over who got the big half of the cookie and who would get the smaller half. I was thinking one day about word problems and how a computer would solve the problem of one cookie, two kids. A computer would say: Easy. Each child gets 50% of the cookie. That is a mathematically fair decision. But in the real world, there’s a big half and a small half, and when you divide the cookie and children fight, it’s a big mess and there’s tears. When I was a kid, if I wanted the big half of the cookie, I would say to my little brother, alright, you let me have the big half now and I will let you choose the show we watch after dinner. My brother would think for a second and he would say, all right, that sounds fair. That was a socially fair decision. Mathematical fairness and social fairness are not always the same thing. Computers can only solve for mathematical fairness. We run into problems when we start trying to use computers to solve problems that require social fairness.
You argue that police departments should not be able to use facial recognition technology and offer some pretty chilling examples of misuse. Can you describe some of the harms caused by this technology in policing?
Facial recognition technology is biased. It has been shown to be better at recognizing light skin than dark skin. It’s better at recognizing men than recognizing women. It is generally not good at recognizing trans and non-binary folks. When it’s used in policing, it has disparate impact. Lots of people think, oh, well, if we just increase the diversity of skin tones in the training data that will make the facial recognition more accurate. And yes, you will make it more accurate, but that does not change the way it gets deployed. It does not change the way it gets weaponized against communities of color, against poorer communities, against gender non-conforming folks.
An interesting way of thinking about different technologies and the risk factors of them comes from the EU legislation [on AI] that is going through right now. They divide AI into high risk and low risk applications.
With something like facial recognition, the facial recognition used to unlock your phone is probably low risk. It doesn’t work most of the time, but it’s not really a big deal. You can just unlock your phone with the passcode and get on with your day. A high-risk use of facial recognition might be police using facial recognition on real-time video surveillance feeds because, again, it’s going to misidentify certain kinds of people more often and certain people are going to get caught up in the legal system unnecessarily, and they’re going to suffer.
You talk about the case of Robert Williams. That case illustrates the harassment that comes when police are sure that their technology is working perfectly.
Robert Williams is a man in Michigan who I discussed in the book. He was arrested because of a false facial recognition match. Somebody stole watches from a store in Detroit. Police sent the grainy video footage to a facial recognition system which ran against Michigan driver’s license photos, among other things, and it found a partial match to Robert Williams’s driver’s license photo. Instead of investigating, the police just went over and arrested him. He is totally innocent. He spent the night in jail and has suffered immensely as a result of this false facial recognition match. This is a good example of what happens when organizations have too much faith in their technologies. The technologies are just not as robust as anybody imagines.
Perhaps even more chilling is the idea of predictive policing. Not just because the tech doesn’t work as advertised, but can you describe the change of culture inside police departments that have adopted the use of this predictive technology?
So, technochauvinism says that computers are more objective, more unbiased, more neutral, more talented than humans. When you believe that, you start to believe in the efficacy. You start to believe that computer systems are infallible. And then you start to make bad decisions. We see this over and over again in policing. There are a number of predictive policing options out there, the idea being that people want to build machines to predict where crime is going to happen, where and even when, or to whom or by whom.
Most of these systems don’t work. There’s a kind of destructive feedback loop that happens.
Crime statistics are not actually records of where crime has happened. They are records of where arrests have happened. Black and brown communities are overpoliced because of decisions that have been made by human beings, because of racial bias. So, you have lots of data about arrests made in certain communities and locations. That gets fed into the computer and the computer says: Well, things happened there. Things are more likely to happen there again. Then you get intensified policing, surveillance, oversight, harassment of these already overpoliced communities. There’s a really interesting project called the White Collar Crime Risk Detector that upends this paradigm. They look at what are the likely locations for white collar crime based on white collar crime statistics. It’s the exact opposite of what you see on most so-called crime maps. The hotspots are Wall Street, which is downtown Manhattan, and I think there’s a hotspot on the Upper East Side.
For all of the thought and coding and time and money and effort that goes into these predictive technologies, it’s a pretty superficial premise to begin with.
It is a superficial premise. It’s just statistics. It’s based on really kind of thin numerical data. It’s not as sophisticated as most people imagine.
You also note that there was no correlation between this greater technological ability in police departments and overall crime rates going down.
Yep.
And how does the idea that these systems can be fixed lead to even more technochauvinism?
People don’t like to hear that sometimes the best solution is not to use technology at all. This often happens because people already spent the money and are sad they’ve spent a lot of money on a thing that doesn’t work. So, they just believe in it for longer than they ought to.
What is the danger of algorithmic predictive technology in the education space?
Well, I wrote about a case during the pandemic when the International Baccalaureate program, which is a prestigious international secondary diploma, couldn’t administer their usual in-person end-of-the-year tests to high school students. So instead, they decided to predict the grades that they thought the students would have gotten on the tests that didn’t happen. This sounds completely absurd. Why would you generate imaginary grades for real students? IB grades are very high stakes. If you get good enough scores on your IB exams, you can get up to two years of college credit, which is a really big deal for families struggling to pay for college. So, I wondered how this decision came about. It was clearly an example of technochauvinism, and it reflected misplaced faith in the ability of computers to determine the future. It’s a good example of why, especially in education, people should not get carried away with the idea that we can automate absolutely everything because we can’t.
To predict the future, these algorithmic systems are looking backward at sometimes irrelevant data. At least in this case, the results were so absurd the students questioned the process. It also shows this quantitative bias, the idea that if you can just reduce things to a number, you can solve any problem. I can see the appeal of it, for sure.
Oh, yeah, absolutely. There’s a quantitative bias inherent in technochauvinism. I think this comes from mathematics. Computer science is a descendant of mathematics, and in mathematics, there’s this kind of great man theory, this idea that mathematicians are geniuses and they don’t have to be bothered with the ordinary concerns that ordinary people have to deal with, that they should just be left alone to think their great thoughts. I am all for people being able to think great thoughts, but then we look at who gets considered a genius. We look at the persistent race and gender problems inside mathematics. We look at the way computer science has inherited those problems – other fields, like physics or politics, also have these kinds of problems – and it becomes very clear there are a whole lot of problems co-occurring.
This helps explain how a white male bias might get applied in different fields as they digitize. Let’s talk about this in medical diagnostic technologies and also get to some of the solutions you propose – evaluating systems for physical limitations, like sensors that don’t detect dark skin, computational biases and bias in how results are interpreted. Diagnostic technologies is maybe a good place to start because racism can be built into both the technology and the human aspect of it, which is legacy racism.
Let me tell you about bias in kidney disease and kidney allocation. For many years, there was an algorithm used to estimate a glomerular filtration rate, an EGFR measurement. This was a standardized measurement used to evaluate when somebody’s kidneys were functioning and when they had deteriorated enough that the person needed to be placed on the kidney donation waiting list. When your EGFR got down to 20, meaning you have about 20% kidney function, you are eligible to wait for a donor kidney. This calculation for EGFR had what was called a race correction built in. So, if you were Black, you got a multiplier that, essentially, meant that Black people got placed on the kidney donor waiting list later than other people because Black people are thought to have greater muscle mass and therefore needed this multiplier. Now, this is an untrue belief. That’s not how bodies work. It is a racist belief. But there are all kinds of racist notions embedded in medicine and embedded in diagnostic technologies.
When you’re building a technological system based on a racist diagnostic criteria, then the racism gets baked into the technological system. It becomes impossible to see and almost impossible to eradicate.
I’m really pleased to say that due to the efforts of activists, patients, doctors, the EGFR formula was changed. It changed while my book was in copy edits, so it now does not use race as a factor in calculations. It’s an example of how these things do not have to be set in stone. But we do have to question our social beliefs that are embedded in our scientific processes, and we’re always going to have to update our technological systems as the world changes. Most people tend to think that technological systems can be something where you can write it once and run it anywhere, and then you can just fire all the people and the computer system will just work forever. That is not at all true. It’s very expensive and time consuming and labor-intensive to update and fix computer systems. Sometimes, it’s easier to just have a human system.
You mention in your book that you have to pick your bias when you’re looking at fairness measurements.
There are different kinds of bias and there are different kinds of mathematical fairness, which a lot of people don’t realize. When you’re evaluating a system for fairness, you have to figure out what kind of fairness or bias you are trying to optimize for. Most people are a little uncomfortable with that, especially when it comes to medical diagnostic systems.
And, generally, the practice is to err on the side of caution, like the alert in medicine is “go see a human,” right?
Yes, which fits with my own sense of caution. People are trying to do AI-based diagnosis for cancer, and we are absolutely all united in wanting to have better treatment for cancer, having better early detection and better treatment. However, we’re not going to be able to replace humans with AI for cancer diagnosis anytime soon. One of the things people often don’t realize about machine learning systems for cancer detection is that these systems have to be set for whether you want a greater rate of false positives or a greater rate of false negatives. That’s just the mathematical reality. So a false negative means that the system says, nope, no cancer when there is actually cancer and a false positive says, yep, probably cancer when there isn’t actually cancer. So, it is totally appropriate that these systems are set to give higher rates of false positives. But then people get sent into a spiral of testing. People who imagine that the AI-based diagnosis is right around the corner are not thinking about the economics and the holistic nature of the whole system.
Can you talk about the EU guidelines for AI and other promising legal remedies to some of these problems?
The EU AI Act recommends using something called a regulatory sandbox in order to test out algorithms and evaluate them because if you’re going to legislate around algorithms, you need to test and evaluate them. I think it’s a great idea.
It seems very sensible.
I worked with my colleague Cathy O’Neill, the author of Weapons of Mass Destruction, to build a system called Pilot, which is a platform for testing algorithms for fairness. The testing and evaluating ecosystem is developing rapidly.
About the Author:
Data journalist Meredith Broussard is the author of More Than a Glitch and Artificial Unintelligence. She is an associate professor at the Arthur L. Carter Journalism Institute of New York University and Research Director at the NYU Alliance for Public Interest Technology.