AI experts offer a detailed overview of how AI predicts, how to apply its predictions and how to exploit the benefits thereof.
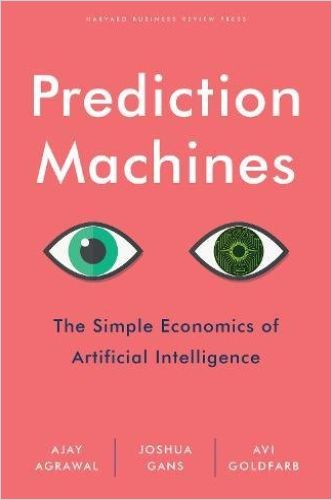
How AI Predicts
Professors at the University of Toronto Rotman School of Management Ajay Agrawal, Joshua Gans and Avi Goldfarb – international leaders in artificial intelligence (AI) and data science – team up to offer a unique, no-nonsense view of AI through the lens of economics. They focus on one of AI’s key features: making predictions. The costs of its predictions fall continuously as their quality improves. This results in more predictions for more things, which, the authors contend, devalues human predictors. So far, they emphasize, AI can make only routine decisions. Thus, those who turn AI predictions into worthy decisions become increasingly valuable. In terms of decisions about AI itself, the authors explore what firms and nations might do, including the extent to which they should embrace AI.
Forbes.com named this one of the Top Ten Technology Books of 2018. Robert E. Rubin, former US Treasury Secretary, found it to be “…a readily understandable guide to artificial intelligence and the immensely consequential effects it could have on our economy, our society and our political system.” Executive editor of Wired, Kevin Kelly, cut to the chase when he wrote the authors made, “… artificial intelligence easier to understand by recasting it as a new, cheap commodity –predictions.” Agrawal, Gans and Goldfarb focus, as per their title, on predictions, the fields that demand them, their cost relative to human or AI labor, and how the price of those predictions will drop as AI becomes ubiquitous.
Predictions
Agrawal, Gans and Goldfarb’s central premise is that by making predictions cheaper and decisions better, AI rapidly changes the world.
From an economist’s perspective, they describe how the near-term future of AI proves blindingly obvious. When the price of a thing drops, demand rises. Agrawal, Gans and Goldfarb explain that as the costs associated with better predictions fall, more people and organizations will rely on AI predictions.
The current wave of advances in artificial intelligence doesn’t actually bring us intelligence but instead a critical component of intelligence: prediction. Ajay Agrawal, Joshua Gans and Avi Goldfarb
When more data are available, AI learns better, and produces a stronger predictive model. The authors credit improvements in computer processing capacity, enormous data sets and falling prices that only recently made AI viable.
More Data
Agrawal, Gans and Goldfarb detail how AI needs historical data to train itself, real-time input data to make sound predictions, and feedback data to improve. The authors make clear that, over time, historical training data decline in importance as current data take precedence.
Prediction machines rely on data. More and better data lead to better predictions. Ajay Agrawal, Joshua Gans and Avi Goldfarb
People make predictions constantly every day. But, the authors note, machines have weaknesses. Foremost, they need lots of data to make worthy predictions. Worse, Agrawal, Gans and Goldfarb warn, AI can think it knows a thing, and provide wrong answers.
Machine Decisions
Agrawal, Gans and Goldfarb offer the example of how London cab drivers once had to study for years to pass rigorous tests of their familiarity with every London street and alley. Thus cabbies could predict the best routes on the basis of time of day, weather and other factors. Today, the authors recognize that GPS devices make predictions from historical and real-time traffic data that vastly outperform London cab drivers.
Agrawal, Gans and Goldfarb explain that the more prediction, judgment and decision-making can shift to machines, though, the lower the costs. Call centers, for example, seek to have machines handle as many customer calls as possible so they can reduce the number of human operators. Thus, the authors point out, the centers value the remaining human operators more for their more astute judgment.
Programmers can install judgment in self-driving cars, for example – but regarding life-and-death moments, Agrawal, Gans and Goldfarb ask, will people accept a machine’s decision? In the moral arena, should drones decide which enemies to kill? Should a self-driving car, for example, hit one person to avoid hitting five others?
All In
Where AI proves efficient at automating one part of a job, the authors found, employees focus on another. Diagnostic AI spots many abnormalities in X-rays, for example, better than radiologists do, but radiologists decide whether to take X-rays in the first place and consult with doctors in interpreting data.
Agrawal, Gans and Goldfarb are adamant that leading in AI requires all-in commitment. You must assess whether having a full in-house AI capability aligns with corporate strategy. If it does, no out-of-the-box solution will work. The authors assert that you must build your own.
Prediction machines carry risks. Any company that invests in AI will face these risks, and eliminating all of them is impossible. There is no easy solution. Ajay Agrawal, Joshua Gans and Avi Goldfarb
Existential threats aside, the authors caution, AI raises implications for privacy, jobs, income inequality, corporate exploitation and national competitiveness.
Primer
Agrawal, Gans and Goldfarb offer a primer on predictions – a deliberately reductive view that strives to clarify one crucial arena of AI as an applicable business solution. By doing so, they offer clear-headed, comprehensible advice to business leaders and students attempting to answer the most basic question: What is AI for? They succeed admirably with helpful examples and the welcome bare minimum of technical talk or technological, mathematical or algorithmical explanations. This reads like a textbook for adults, though professors and young entrepreneurs will benefit from Agrawal, Gans and Goldfarb’s approach. Those well-versed in AI and its applications may find that the authors too accommodating to beginners. But beginners are this book’s market.
Other AI business strategy books include Competing in the Age of AI by Marco Iansiti; Human + Machine by Paul R. Daugherty; and The Master Algorithm by Pedro Domingos.