Professor of marketing at the University of Pennsylvania’s Wharton School of Business Kartik Hosanagar offers an overview of the pragmatic and philosophical issues algorithms incarnate.
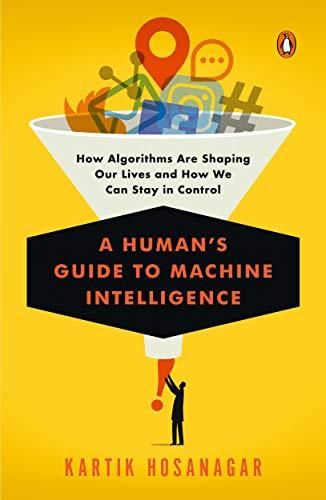
Algorithms Rule
Algorithms “nudge” you into choosing a certain movie, restaurant, song, lover or political opinion. Kartik Hosanagar – John C. Hower Professor of Technology and Digital Business and a professor of marketing at the University of Pennsylvania’s Wharton School of Business – explains that algorithms are basically recipes; designers don’t always know what they cook up. But mostly, according to Hosanagar, algorithms make better decisions than humans. He argues that greater transparency and an “algorithmic bill of rights” can empower users and hold tech companies accountable.
Human Flaws and Unpredictability
Algorithms stand at the core of almost all online activity. An algorithm is a recipe, a set of instructions. However, with improvements in machine learning, algorithms aren’t only making suggestions about what to watch or whom to date, but real-world decisions, such as navigating traffic.
Algorithms aren’t infallible. Humans create them and bake human biases into them. For example, a program that officials used to make prison sentences more equitable in Florida mislabeled white defendants as “low risk” twice as often as Black defendants, even when the Black defendants’ criminal records were far less damning. And, as do humans, algorithms can behave in unpredictable, irrational ways. So can they be trusted with human health, safety and happiness?
To discard [algorithms] now would be like Stone Age humans deciding to reject the use of fire because it can be tricky to control.Kartik Hosanagar
Hosanagar cites three types of unanticipated consequences that algorithms fall prey to. “Unforeseen benefits” occur when serendipity intervenes. A famous example is Viagra, which doctors originally created to reduce high blood pressure. “Perverse results” occur when your intervention worsens the outcome you seek to improve. “Unexpected drawbacks” occur when negative results appear in addition to positive ones.
Resilience and Predictability
Today’s AI programs have “deep learning” abilities through which they teach themselves. AlphaGo, for example, did not learn the rules of the game Go. It deduced them from playing the game over and over.
Technology is most useful when it helps us solve the most creative problems we face as human beings.Kartik Hosanagar
AlphaGo was a success, but made inexplicable, sometimes suicidal, moves. These were not human moves, and, Hosaganar notes with great interest, AlphaGo’s designers could not explain them. Like children, deep learning systems observe and emulate, which makes them resilient. But also like children, they are unpredictable. A resilient system offers greater security, because hackers can more easily penetrate predictable systems. But with the absence of transparency about its decision-making process, a resilient system can put people at risk.
Nature and Nurture
Hosanagar cites how, in China, the chatbot Xiaoice “learned” from users to be friendly and empathetic, but in the United States, Microsoft’s Tay learned to be bigoted and aggressive. Tay’s nurturing element revealed the structural weaknesses in her “nature” – her programming.
Algorithmic systems consist of the data that trains them, the logic in their design and interactions with users. What algorithms learn feeds into the next generation of data they will use to make further recommendations and decisions. From these interactions, intended and unintended outcomes arise.
Trust and Algorithms
People are comfortable with algorithms deciding the music they listen to, shows they watch or people they date. But most resist putting their lives in the care of decision-making machines. Hosanagar claims that self-driving cars have a much lower accident rate than human drivers do, then castigates people for believing humans have greater driving skill. But self-driving cars won’t be a viable option for some years; this makes Hosanagar seem somewhat credulous.
Deep learning algorithms can potentially transform diagnostic capacity, because they can analyze, compare and contrast millions of diagnostic images to make predictions. Doctors, however, resist allowing them to make unchecked diagnoses, but embrace predictive systems that grant doctors the final say.
A Bill of Rights
Hosanagar offers these guiding principles: awareness – designers must take into account the potential harm their algorithms cause; “access and redress” – victims of negative algorithmic behavior must have avenues to seek answers and obtain redress; and accountability – even if designers don’t know why an algorithm made a certain decision, they must be responsible for it.
Though Hosanagar doesn’t distinguish between these principles, accountability seems the one most likely to cause the gravest, most intractable disputes.
Explanation means that people whom algorithms affect have a right to know why those algorithms behave as they do. Frustratingly, Hosanagar does not explain how such explanations might be rendered. “Data provenance” means creators must keep a record of training procedures.
Where will we set boundaries when technology’s limits aren’t setting them for us?Kartik Hosanagar
In the greatest understatement of his book, Hosanagar notes that these are laudable principles, but require enforcement to be meaningful. He suggests that regulatory bodies of policy specialists and professionals who understand the technology could generate and impose rules of conduct. Hosanagar argues that users should have some control over how algorithms work, and receive alerts on unintended consequences.
Not Quite Complex
Despite Hosanagar’s admirable credentials, he offers only a basic – though erudite – overview of algorithms’ functions in society, medicine, driving and flight. Readers should regard this as a worthy, standard college text on the subject for those new to the topic and unaware of its complexities. Hosanagar is a superb writer, and rightfully most intrigued by algorithm’s inherent – and humanlike – contradictions and oxymorons. But his later chapters regarding consumer protection and regulation are surprising in their naivety. Hosanagar seems to ignore the fundamentals of today’s runaway giant tech capitalism and how nontransparent those giants are regarding the algorithms on which their market dominance depends. Or how unwilling they are to offer consumers any protection from them.