CEO and former philosophy professor Reid Blackman provides a detailed, thoughtful and thoroughly applicable ethical guide for companies vested in machine learning (ML) and artificial intelligence (AI).
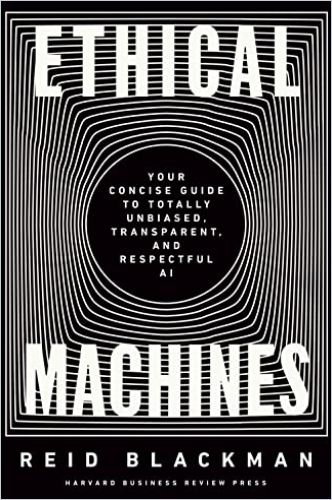
Equitable AI
Former philosophy professor Reid Blackman is founder and CEO of Virtue, and works with companies to integrate ethical risk mitigation into the development, procurement and deployment of emerging technology. In Ethical Machines, he argues that AI’s ethical problems affect humans and business ventures, and will worsen as AI scales up.
Ethics and AI
Businesspeople, scientists and engineers work with hard facts, not abstract concepts like ethics. Blackman emphasizes that ethics have practical applications.
If you’re particularly averse to the ethical and reputational risks of AI or you want to be known for being at the cutting edge of ethical AI, you can drive it into every facet of your organization.Reid Blackman
People who pursue “AI for Good” attempt to seek a positive social impact, such as reducing poverty. “AI for Not Bad” focuses on avoiding or mitigating AI’s many potential ethical problems. Blackman cites examples of AI programs in health care, the financial industry, and human resources, which discriminate by race and gender. These AI lapses are wrong in and of themselves. They also affect an organization’s reputation, and render it vulnerable to regulations and laws.
Filmmakers and speculative thinkers often associate AI with “Artificial General Intelligence” (“AGI”), which would mimic the human mind’s capacities on a large and powerful scale. AGI doesn’t yet exist. Businesses use “artificial narrow intelligence” (“ANI”), which relies on “machine learning” (“ML”) for concrete tasks, such as evaluating whether someone qualifies for a loan. Thus, ethical risks arise around ANI.
Blackman explains how AI and ML turn data inputs into outputs of mathematical operations. The input data might be a person’s rental or financial history. The output would be whether that person can rent an apartment or receive a loan. AI generates the output regardless of the validity and representativeness of the data used as input.
Discriminatory AI typically results from problems with the “training data” that people feed into the ML model. Prejudice is rampant – against women, Black people, and many others; the data people input for AI reflects such prejudices.
To address or mitigate AI’s bias and unfairness, Blackman suggests you analyze your data for discriminatory attitudes and practices. If you discover discriminatory outputs, seek more data from more diverse sources. Or, reconsider the goal of this AI and whether it internalizes biases, and whether you have an appropriate fairness standard for the AI you are developing.
Machine Explainability
If the bank denies your mortgage application, for example, naturally you want to know why. Today, loan decisions are often automated via AI. The AI is a “black box” – you can’t look into it and determine how it arrives at its outputs.
Blackman believes that machine explanations should allow the person whom an AI decision affects to understand that decision and whether it was sensible and fair. Other criteria for machine explanations should be “truth, ease of use and intelligibility.” If the issue is important, the explanation’s recipient should understand it. Qualified people must provide the template for such explanations; these are likely beyond the purview of engineers and scientists.
Privacy Concerns
Blackman reminds readers that companies will collect as much data about you as they can – whether or not you know they are collecting it – and train ML models for AI to make predictions and decisions that affect your life.
Privacy today exists to the degree that you control your own data. Privacy in AI ethics concerns transparency, control of collected data, the ability to “opt out,” and inclusive services. Transparency involves informing consumers that their data are being collected and who is using their data. Most consumers lack control over their data that end up online. Ideally, consumers should have to explicitly opt in; the company must convince the person that it uses his or her consumer data responsibly. Companies should also provide full services regardless of whether a user chooses to share data.
Many people, Blackman notes, erroneously believe AI to be “ethically neutral.” But, he points out, an AI’s character depends upon how people developed it and what data sets they gave it for training.
There is no such thing as ethically neutral AI… When you develop AI, you are developing ethical – or unethical – machines.Reid Blackman
Blackman advises you to build your own explicit “AI ethical risk program.” Start with articulating your values. Insist upon fairness and human respect. Reveal how you use customer data. Protect people’s privacy, and indicate how you use “anonymized” data. Offer ample opportunity for customer feedback.
Articulate your values by considering your ethical worst-case scenarios. For example, if you run a social media platform that aspires to connect people, its users might spread disinformation. Show how your values wed to your organization’s larger mission. Relate your values to what you regard as “ethically impermissible.” Educate employees on these issues, engage in ethical risk analyses at all stages of AI’s use, and track your AI’s impact.
Ethical Issues
Blackman points out that a significant portion of AI’s ethical risks relate to “bias, explainability and privacy.”
By now, you’re probably thinking, how the hell are we going to handle all these questions?Reid Blackman
Develop your ethical standards, he advises, and ensure everyone in your organization – from engineers and scientists to HR – understands them. Create a culture in which people take this issue seriously. Enable your product development people to evaluate the ethical risks inherent in the offerings they create. Give all employees financial incentives for taking AI ethics seriously and monitor the applications of your standards. Help product developers identify and agree upon ethical risks, and what they should do about them. Involve ethical experts or ethicists from the product design stage onward.
Sound Advice
Reid Blackman’s background as a philosophy professor and his current role as a CEO gives his advice a solid abstract and practical foundation. Blackman’s combination of expertise is, to say the least, unusual for an author of a guide to business practices. The former means he writes with skill and concision; the latter means he never loses sight of practical objectives. Blackman melds these universes quite well, balancing the philosophical and the practical to provide maximum understanding for readers from all fields. Blackman’s pragmatic, informed advice makes this a crucial first stop for entrepreneurs and executives in formulating a program for AI ethics.